Building sector consumption encompasses 40% of global primary energy and contribute to in excess of 30% of CO2 emissions [1]. In particular, the residential users comprehend more than 60% of the total energy consumption of the building sector [2]. Thereby, the research community and governments are paying more attention to energy efficiency and renewable energy technologies application in the residential sector in order to cope with its increasing energy demand.
In this framework, the residential sector stands out as one of the most significant energy consumers and highly affected by the actual directives. The energy and environmental policies coincide in highlighting the role of energy efficiency and climate change estimating, in the horizon of 2020, a reduction of its overall emissions to at least 20% below 1990 levels as well as an increase in renewables energy use to 20% [3].
Buildings perform most efficiently if all systems are controlled and integrated. Without upgrading existing appliances, the building efficiency can increase up to 30%, with an appropriate energy integration [4]. Furthermore, energy savings increase with the level of integration and can achieve more than 80% when the system design is optimized [5]. Thus, polygeneration systems pop up as a large potential once it promotes the energy integration required to achieve building energy efficiency and environmental targets.
Polygeneration applied to buildings has been broadly studied in the literature. For instance, Calise et al. [6] compared the polygeneration system applied to an office and a gym in different configurations. The proposed system was more profitable in the application with higher hot water demand, in this particular case, the gym. Roseli et al. [7] evaluated the integration of an electric heat pump driven by a photovoltaic field in two different configurations. In the first case, the system was connected to the grid. In second configuration, the system was also connected to grid but a battery bank was added as well. In both configurations, the system was applied to a small office and a reduction in primary energy consumption was verified. Acevedo et al. [8], designed a system combining photovoltaic/thermal collectors, an evacuated tube collector and a wind turbine to produce electricity, sanitary hot water and desalted fresh water for a single-family house. Sigarchian et al. [9] evaluated the effect of the operational modes on the performance of a polygeneration system in a residential building complex in northern Italy.
Uche et al. [10] showed experimental tests of a polygeneration pilot plant, for power, freshwater and domestic hot water production, designed for a typical family home (four residents) isolated from the power and water networks. Buonomano et al. simulated a small trigeneration plant supplied by geothermal and solar energies for a hotel in Ischia, in South Italy. Results showed that the system performance is more dependent on the availability of the geothermal energy than the solar one [11]. Considering a small residential building, Calise et al. designed and simulated a system combining solar cooling, solar-assisted heat pump and photovoltaic/thermal collector technologies. System performance presented to be highly sensitive to the PVT field area [12]. Angrisani et al. studied different solar panel technologies, tilt angles and collecting areas in a simulation of a solar heating and cooling system in an office building located in Southern Italy. The results showed a primary energy saving and equivalent dioxide carbon emission reduction while compared with conventional system [13]. Calise et al. evaluated energy and economic feasibility of a solar-assisted heating and cooling system for different types of school buildings and Italian climates. Despite the potential of energy saving, the author concluded that the economic profitability can be achieved only in case of public funding policies [14].
However, in order to promote reliable polygeneration systems simulations, detailed demand profiles of the analyzed building are required. Energy demand can be defined as the energy required by a system to provide energy services to the final users. The main residential demands can be divided into cooling, heating, domestic hot water and electricity (lighting and appliances). It is important to note that the thermal energy (heating and cooling) is the highest consumer and represents 45% of the energy consumption in the EU residential sector [15] and depends on the thermal characteristics of the building, ventilation, indoor and outdoor climatic conditions and energy end-uses [16].
Thus, different methods of building energy simulation (BES) for energy demand estimation can be defined. The models can be classified as diagnoses or prognosis and law or data [17]. Coakley et al. [18] describe that models applied to BES are, basically, prognostics. Although, they can be driven by both law or data. The prognostic law-driven model (computational simulation) is used to predict the behavior of a complex system given a set of well-defined laws (e.g., energy balance, mass balance, conductivity, heat transfer, etc.). Whereas, the prognostic data-driven approach (statistical analysis) uses monitored data from the building to predict accurately the energy demand behavior. The study conducted by Yoshino et al. [19] highlighted the main research methods, findings and outcomes regarding the robust prediction of total energy use in buildings.
A few examples of detailed BES for energy demand estimation can be found in the literature. In particular, a study performed by Karlsson et al. [20] used three different tools to simulate a low energy terraced house in the south of Sweden. The measured values for total electricity demand ranged from about 6,000 kWh to over 12,000 kWh. Richardson et al. [21] presented a high-resolution model to estimate the demand for electricity in a dwelling. This model was validated through samples of 22 houses located in East Midlands, United Kingdom. The model can be modified according to specific requirements and be incorporated into other models. Carvalho et al. [22] carried out a study along a period of 1-year to evaluate the demand for a medium-sized hospital located in the city of Zaragoza in Spain. The demand outcomes showed a maximum heating demand of 50,007.0 kWh/day in January and a maximum cooling demand of 20,170.0 kWh/day in July. Electricity demand achieved a maximum and minimum demand of 9,411.0 kWh/day and 7,802.0 kWh/day, respectively.
Thus, there is a lack of energy demand information regarding the residential sector. This data is indispensable for the simulation of reliable polygeneration systems. For that reason, the aim of this paper is to estimate an hourly generic pattern for all main energy demand required by the residential sector. As a reference case, a single-family dwelling located at the Mediterranean European side is considered. The results can be useful for improving the knowledge about energy demand estimation and providing basis for polygeneration systems simulations applied to the residential sector.
The reference case is a single-family townhouse located in the Mediterranean European side. The energy demand patterns estimated are electricity, heating, cooling, domestic hot water (DHW) and freshwater along 1-year simulation with 1-hour time step.
The reliable tool TRansient SYstem Simulation (TRNSYS) [27] was used to estimate the heating and cooling demands by a detailed energy performance evaluation of the residential building. TRNSYS simulation software evaluates the energy required to maintain a specified building condition (e.g., temperature and humidity). It performs a detailed heat-balance calculation under the influence of external and internal inputs (weather, infiltration, occupancy, lighting, equipment loads, etc.).
On the other hand, electricity, DHW and freshwater demands were obtained by statistical analysis reported at the literature based on studies previously validated. The reference dwelling parameters were used as input for all approaches. Furthermore, a thermal comfort study and a primary energy saving (PES) analysis were performed to validate the assumptions and highlight the importance of the residential sector.
The city of AlmerÃa, in the Mediterranean European side of Spain, was selected due to its high summer climatic severity and, therefore, the high total annual irradiance, which accounts to 2,587 kWh/m². Meteonorm weather data of AlmerÃa (36°50′17″ N, 2°27′35″ W) was used for climatic simulation purpose. Figure 11 shows the hourly variation of available global solar radiation on the horizontal surface and the dry bulb temperature for the whole year in this location. The global solar irradiance encompasses the direct incident beam radiation at the surface, the diffuse radiation that has been scattered through the molecules of the atmosphere and the albedo, radiation reflected by the surroundings [23].
Hourly mean solar radiation and ambient temperature, AlmerÃa, Spain
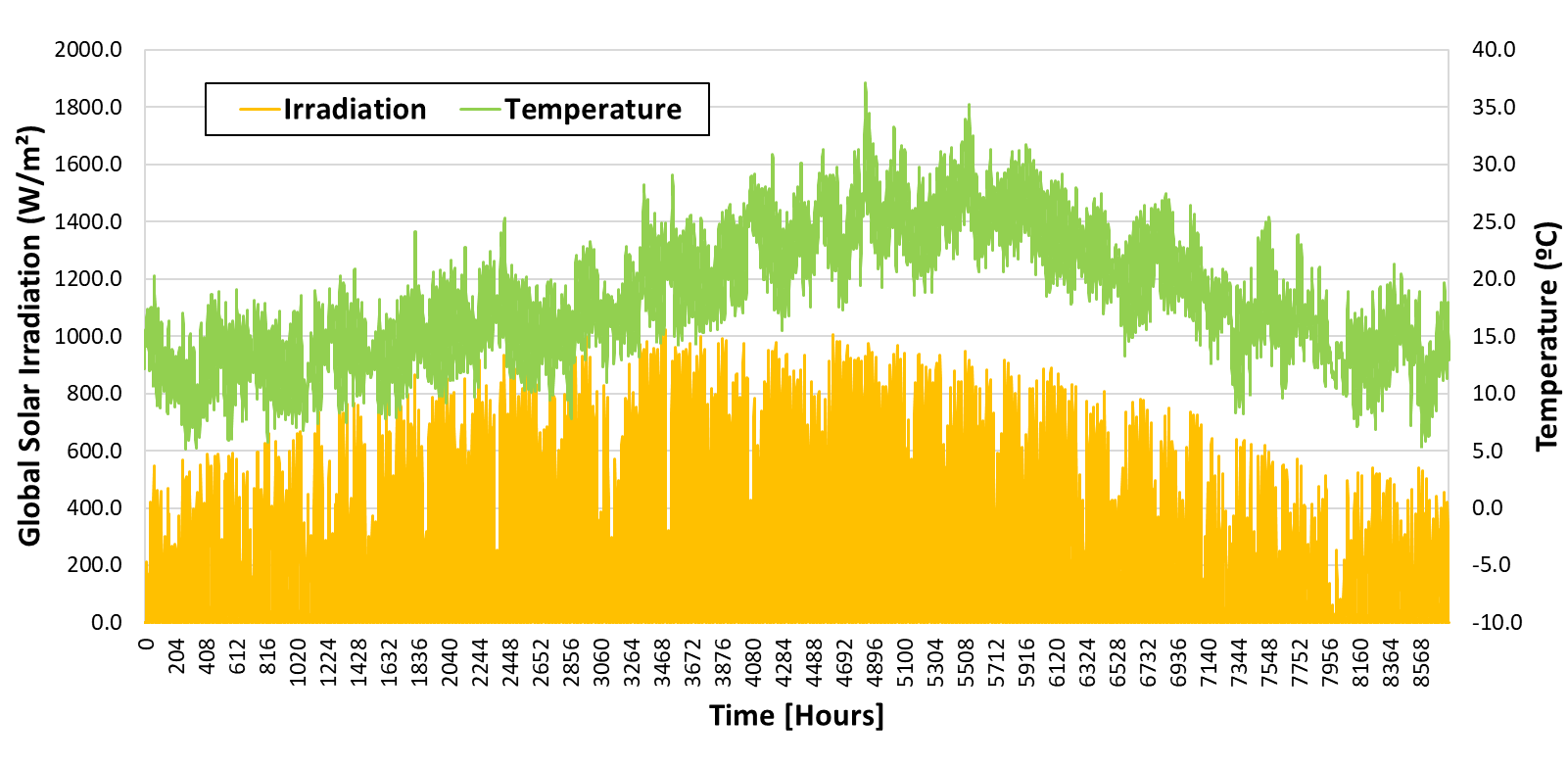
The space heating and cooling demand was dynamically simulated with TRNSYS in order to obtain the building thermal behavior. The model utilizes several components from the build-in component library considering both physical components (building, ground temperature, etc.) and controlling devices (schedules, calculators, unit converters, etc.). Moreover, the simulation tool also includes a number of additional components required to run the simulations and to process the results, such as weather data readers, integrators and plotters. All main types used in the proposed system model are listed in Table 1 and showed at Figure 2.
It is important to note that, the software built-in components are validated against experimental and/or performance manufacturers’ data. Thus, the results of the simulation of the proposed system are highly reliable. Furthermore, the model under investigation can be analyzed by means of instantaneous or integrated data on whatever time period (hours, days, weeks, months or years). A detailed description of all component models used in the simulation are omitted, however it is available in the TRNSYS component mathematical reference [24].
TRNSYS main types used in the model
Component |
Type |
Component |
Type |
Weather data reader |
15 |
Ground temperature |
77 |
Building |
56 |
Schedulers |
14 |
Unit converter |
57 |
Printer/plotter |
65 |
Regarding the main components, the TRNBuild (Type 56) is the building input description tool of TRNSYS studio and simulates the multi-zone building thermal behavior. TRNBuild is an interface for creating and editing all of the non-geometry information required by the TRNSYS BES model. It allows the user extensive flexibility in editing wall and layer material properties, creating ventilation and infiltration profiles, adding gains, defining radiant ceilings and floors, and positioning occupants for comfort calculations. Furthermore, Meteonorm weather data of AlmerÃa, Spain is given by Type 15 and Type 77 calculates the ground-coupled heat transfer according to the IEA Task 34/43 [25].
Pictorial view of the BES model in TRNSYS studio
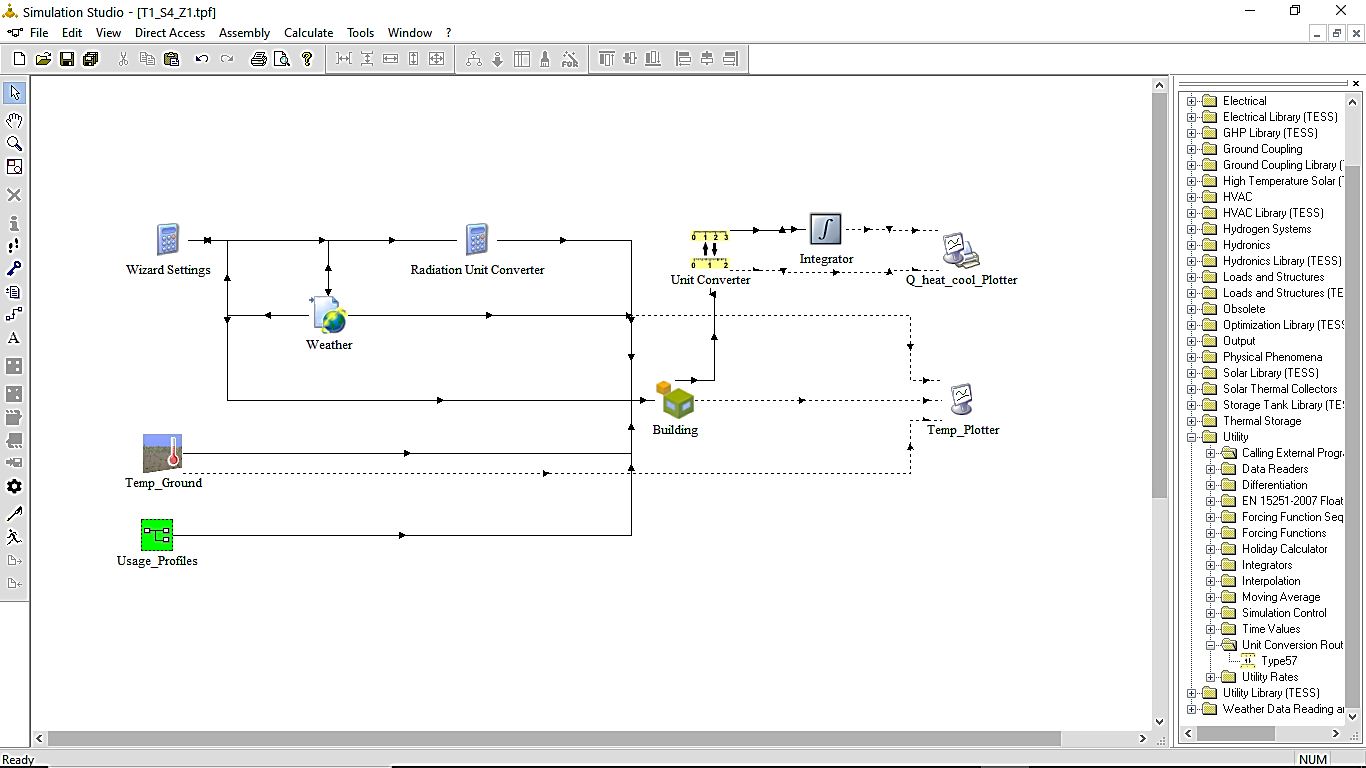
The usage profile encompasses in a macro all user defined information in order to simplify the TRNSYS main scheme. Figure 3 presents the usage profile macro breakdown. It presents all the inputs required by Type 56 to perform the BES model. In particular, the space heating and cooling thermostat setpoint control, the ventilation and infiltration airflow profiles and the lighting, equipment load and occupancy gains.
Pictorial view of the usage profile macro
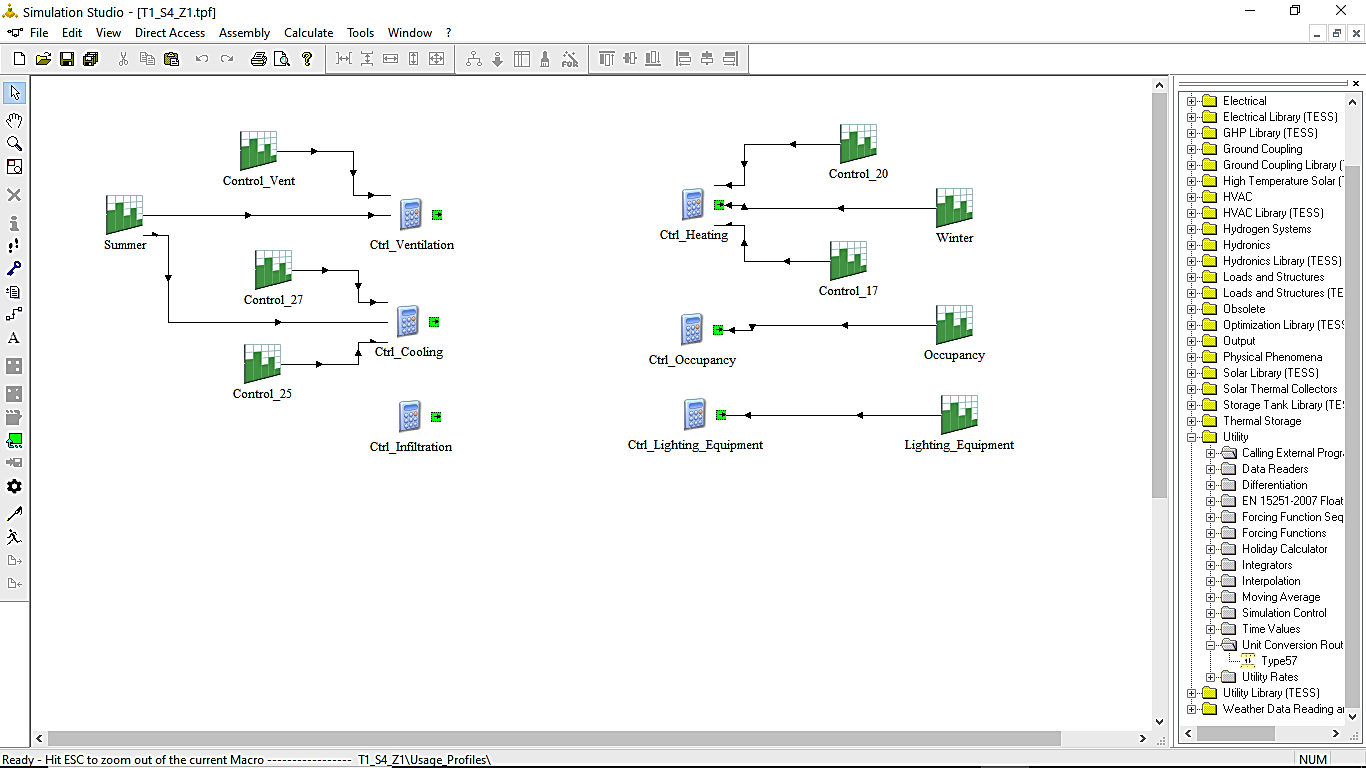
The thermal energy demand of a residential building is a function of the building type, size, materials used for construction, occupancy and location [26]. In particular, a typical single-family townhouse of 110 m2 with four occupants, as shown in Figure 4, was designed. It consists of 2 floors and an attic with a total height of 7.5 m. The side walls are adiabatic. Fenestration comprehends 10% and 15% of the north and south façade area respectively. Trnsys3d plugin [27] for Google SketchUP [28] was used to build the 3D dwelling geometry divided in thermal zones for the proper building thermal evaluation. Furthermore, it provides a direct link between SketchUp and TRNBuild for BES.
3D of the reference dwelling
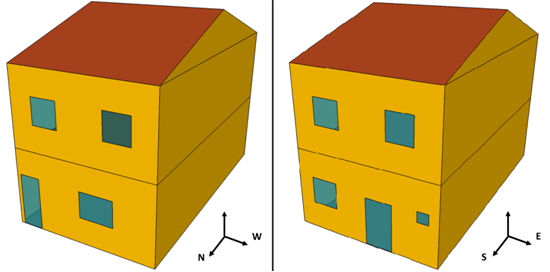
The house envelope comprehends the external walls and roof, the floor and the external doors and windows. The dwelling meets the transmittance thresholds defined by the basic document of energy saving of the Spanish technical building code: BD-ES [29], summarized in Table 2. However, no envelope components layers (material, thickness, density, etc.) were specified. The convective coefficients of the interior and exterior surfaces of each envelop component are calculated using a specific dynamic algorithm defined in TRNSYS engine. It is worthy to note that no thermal bridges were considered in this study, nor internal walls and doors.
Transmittances of building envelope components
Element |
Value [W/ (m2.K)] |
External wall |
0.50 |
External roof |
0.47 |
Floor |
0.50 |
Door |
2.20 |
Window |
2.60 |
Regarding the building usage profile, Table 3 presents the space heating and cooling setpoint temperature operation, the occupancy, lighting and equipment load profiles and the ventilation and infiltration air rate. Ventilation is the controlled air change of the building interior air to ensure its quality, whereas infiltration consists of an uncontrolled air renovation through various elements of the building envelope.
As shown in Table 3, an addition of 4 air changes per hour in ventilation airflow rate is considered during the cooling season, which represents the opening of windows proposed by the Ref. [29]. During the whole year, a constant ventilation rate is defined according to the procedure described in the basic document of hygiene, salubrity and environmental protection of the Spanish technical building code: BD-HS [30].
An annual mean infiltration rate was defined according to the fenestration permeability. It is important to note that infiltration depends on the thermal and pressure gradient between the building interior and exterior, the wind and the air permeability of the building envelope. However, for reason of brevity, a constant infiltration rate along the year was defined based on the results of Trejo [31] for an average fenestration tightness.
Building usage profile
Setpoint temperature |
Heating season |
17 ºC |
00:00 – 08:00 |
|
20 ºC |
08:00 – 24:00 |
|||
Cooling season |
27 ºC |
00:00 – 08:00 |
||
– |
08:00 – 16:00 |
|||
25 ºC |
16:00 – 24:00 |
|||
Occupancy load |
3.51 W/m² |
Weekday |
100% |
00:00 – 08:00 |
25% |
08:00 – 16:00 |
|||
50% |
16:00 – 24:00 |
|||
Weekend |
100% |
00:00 – 24:00 |
||
Lighting load/ |
4.40 W/m² |
50% |
00:00 – 01:00 |
|
Equipment load |
10% |
01:00 – 08:00 |
||
30% |
08:00 – 19:00 |
|||
50% |
19:00 – 20:00 |
|||
100% |
20:00 – 24:00 |
|||
Ventilation rate |
Heating season |
0.4 1/h |
00:00 – 24:00 |
|
Cooling season |
4 1/h |
01:00 – 09:00 |
||
0.4 1/h |
09:00 – 01:00 |
|||
Infiltration rate |
0.45 1/h |
00:00 – 24:00 |
An electrical demand curve was generated using a high-resolution stochastic model of domestic electricity demand developed by the Centre for Renewable Energy Systems Technology (CREST). The model produces 1-hour resolution demand data, disaggregated by end-use, using a bottom-up modelling approach based on patterns of active occupancy and daily activity profiles derived from time-use survey data. This model was validated through samples of 22 houses located in East Midlands, United Kingdom. The user may configure the month of the year, the total number of residents and whether a weekday or a weekend day simulation is required [21]. The model was adapted to the reference case through the replacement of the climatic data, the appliances selection according to local characteristics and the lighting control calibration.
In order to estimate the domestic hot water (DHW) demand, a realistic daily time dependent simulation was performed using the DHWcalc software [32] developed for the IEA-SHC Task 26, which can generate realistic DHW profiles for European countries in several time steps. This software has been successfully used and validated in previous literature [33].
For a single-family house, the software considers flow rate distributions for small (washing hands) and medium (dishwasher) draw offs, shower and bath. A pre-defined probability distribution based on Gaussian and step functions assumes a constant probability for draw-offs for small and medium draw offs during daytime and nighttime, respectively. For shower and bath, Gaussian distributions with peaks in the morning and evening, respectively, are defined to describe the probabilities during the day. Each category-profile is generated separately and superposed afterwards [34].
In the particular case, the total mean daily water volume, duration and time step were set based on Ref. [29]. [29][35]The daily water volume was assumed to be 112.0 l/day considering an average consumption per person of 28.0 l/day and 4 people occupancy. Finally, the software distributes all draw offs throughout the year according to its probability function.
Furthermore, to estimate the DHW thermal demand, a typical outlet temperature of 60ºC was chosen in order to meet the legionella requirement [30] and an annual mean inlet temperature of 16ºC was calculated according to the monthly aqueduct water temperature from AlmerÃa reported at Ref. [29] and presented at Figure 5.
Monthly mean aqueduct water temperature of AlmerÃa
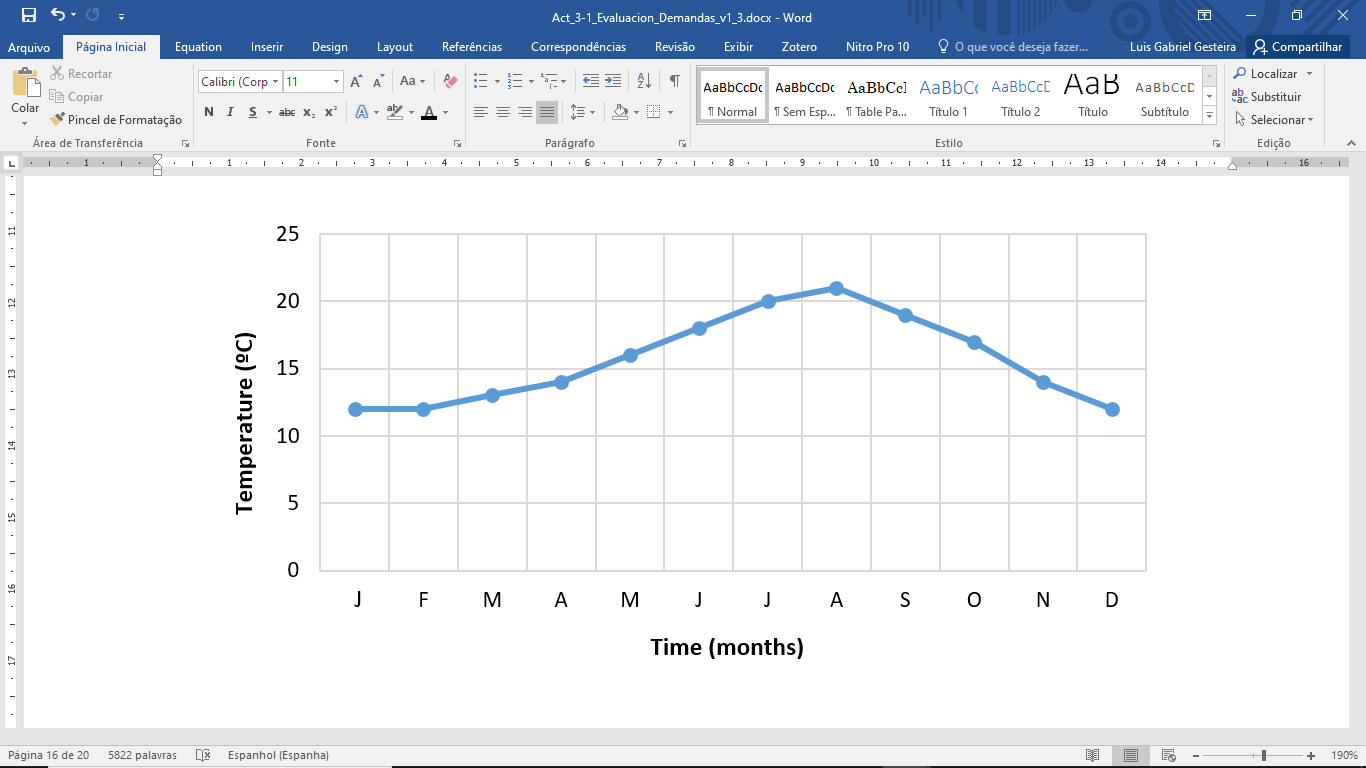
STREaM (STochastic REsidential wAter end use Model) is a stochastic simulation model for synthetically generating high resolution time series of residential water use at the end-use level developed and validated by Ref. [36]. Each water end-use fixture simulated is characterized by its typical consumption pattern, as well as its probability distributions of number of uses per day, single use durations, water demand contribution and time of use during the day. Therefore, the generated profiles represent the heterogeneity of the different fixtures’ signatures, with the toilet and the faucet characterized by an almost instantaneous pulse equally distributed during the day, while shower and clothes washers correspond to longer consumption events. The hourly distribution of the shower and the dishwasher consumption contributes to the morning and evening peak, respectively. Whereas the clothes washer is simulated overnight as this use occurs during off-peak times [37].
In this framework, the freshwater consumption of a single-family dwelling with 4 residents was simulated. The set of fixtures chosen consisted of toilet, faucet, clothes washer and dishwasher. No shower was selected here in order to avoid redundancy once it was considered in the DHW study.
The thermal comfort of building users is performed by a built-in subroutine included in TRNSYS to validate the assumptions taken from Refs. [29] and [30]. This model is developed according to the International Standard Organization (ISO) 7730 standard [38], described in details by Fanger [39]. The main calculation procedure, reported by Fanger, establishes a statistical correlation between the Predicted Mean Vote (PMV) thermal comfort index and the thermal load acting on the human body:
The PMV index is calculated according to the energy loss to the environment (L) and the metabolic rate (M) of the users. The PMV is an index that aims to predict the mean value of votes of a group of occupants on the ambient thermal comfort sensation. The index is translated as +3 for a too hot sensation, while -3 as too cold ambient. The 0 value represents a neutral comfort condition. To consider the satisfaction level of the occupants in an ambient, Fanger developed another equation to relate the PMV to the Predicted Percentage of Dissatisfied (PPD), defined as follows:
 |
(2) |
The TRNBULD comfort subroutine includes the procedure to calculate both PMV and PPD as a function of the ambient climatic condition and the person-specific parameters. Regarding the person-specific parameters, a metabolism rate, clothing insulation and air velocity were taken from ASHRAE Standard 55 [40] as it can be seen in Table 4.
Thermal comfort parameters
Parameter |
Description |
Factor |
Clothing factor |
Light summer clothing |
0.5 clo |
Metabolic rate |
Seated, light work |
1.2 met |
Air velocity |
Still air |
0.2 m/s |
A primary energy saving (PES) analysis was carried out to investigate the building efficiency potential regarding a suitable reference case (RS) considering the electricity, DHW, heating, cooling and freshwater production through conventional technologies. The RS consists of an electric vapor compression air-conditioning (AC) for space cooling characterized by an average coefficient of performance of COPCool,RS = 2.5 [41] and a natural gas boiler (GB) for DHW and space heating production with an average conversion thermal efficiency of ηth,RS = 0.92 [29]. The national grid for electric demand features an electrical efficiency ηel,RS of 0.46 [42]. The freshwater demand () is assumed to be provided by a reverse osmosis desalination plant with a specific consumption of ϵRS = 4 kWh/m³ [43]. The PES achieved by the RS is calculated as showed in Equation 3:
PES = |
(3) |
Where PEl,dem is the building electrical demand which is considered to be withdrawn from the national grid. QDHW,dem, QHeat,dem and QCool,dem are the thermal energy demand required to be supplied by a GB for DHW and space heating and by an AC for space cooling, respectively. Whereas, is the total freshwater demand provided by a conventional desalination system.
The outcomes of this work is mostly based on generic power and flow rate profiles for a single-family dwelling for each energy demand. Daily, weekly and yearly time bases were used for instantaneous and integrated results of electricity, heating, cooling, DHW and freshwater demands. All simulations were performed based on a 1-year time period (from 0 h to 8760 h) with 1-hour time step in order to decrease the simulation running time. Nevertheless, all data reported here can be generated in lower time step or mathematically interpolated. The building thermal comfort was analyzed for two scenarios: without an indoor temperature control and controlled by the setpoint temperature established by the BD-ES. Finally, a PES analysis was carried out in order to present the building efficiency potential and verify its BD-ES compliance.
In this section, the daily demand behavior for each demand are discussed. Figure 6 shows the heating and cooling demands. It is important to note that heating demand refers to a day in the winter season (February 1st) whereas the cooling daily demand was taken from the summer (August 1st). During the winter, heating is required in the mornings from 08:00 to 13:00. Users’ daily routine (work, school, etc.) and the ambient temperature decrease during the night are responsible for this demand. Conversely, during the summer, the cooling demand is required between 16:00 and 24:00. It also coincides with the users’ daily routine (after occupation) and a higher ambient temperature due to longer daylight during this season.
Daily heating and cooling demand
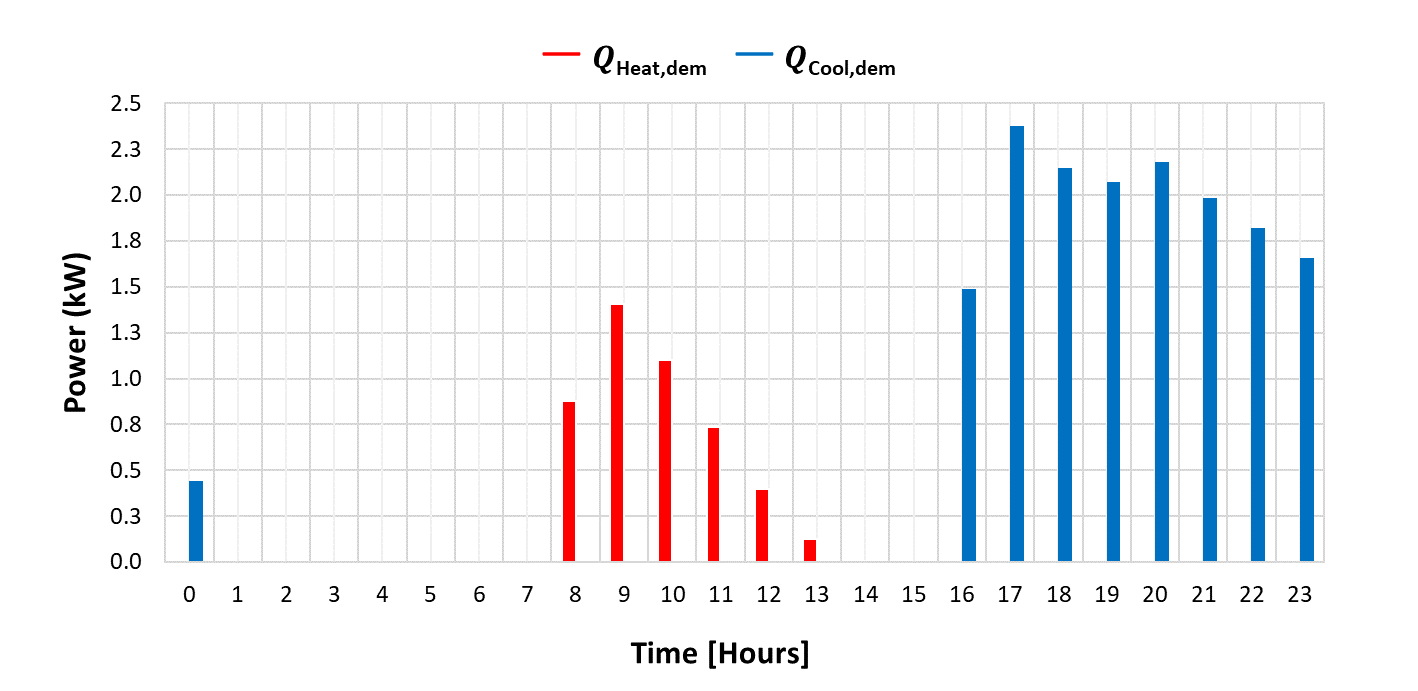
Figure 7 displays the electricity demand profile for January 1st during 24-hours long with 1-hour time step. The greater consumption is shown between 19:00 and 22:00 due to higher occupancy and lighting and appliance use. This period of time represents the daily electric power peak demand.
Daily electrical power demand
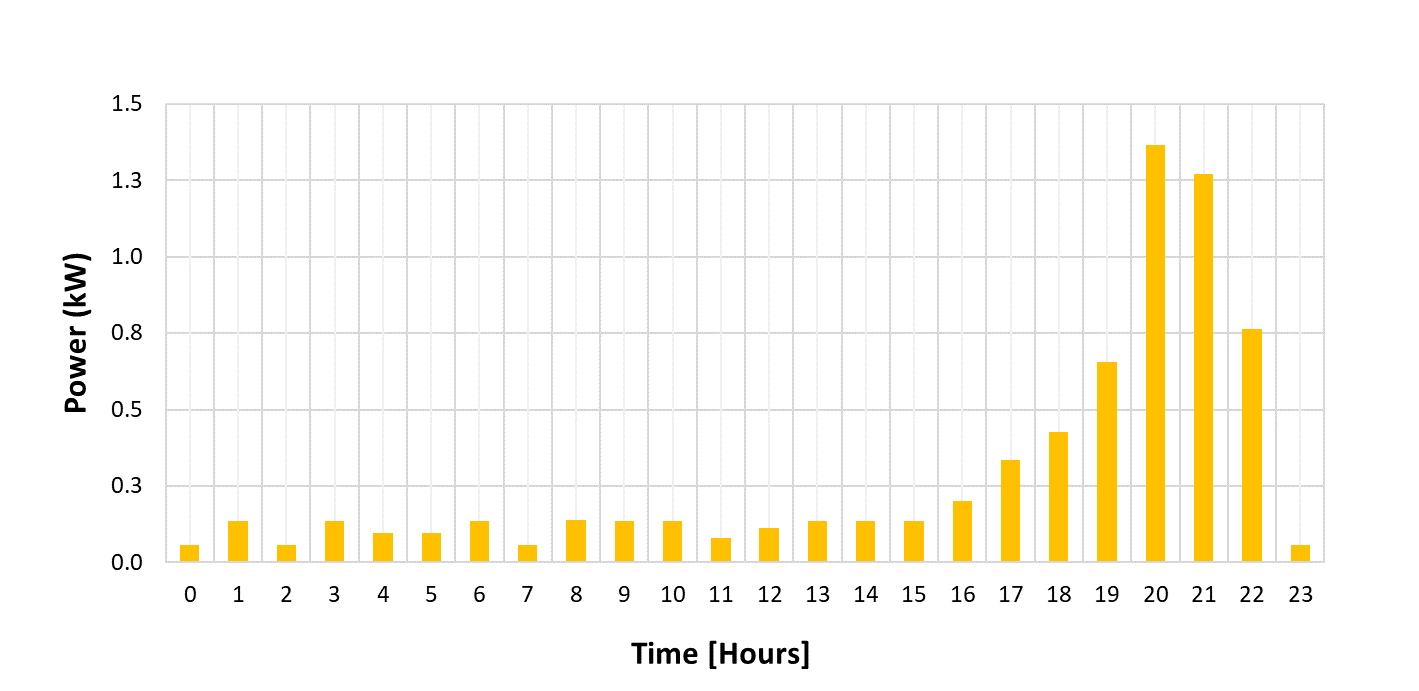
DHW and freshwater demand profiles were aggregated in Figure 8 based on the data estimated for January 1st. It is worth noting that, DHW has a very characteristic peak at 20:00 due to showering. On the other hand, freshwater is more scattered along the day with a peak at midday (from 11:00 to 12:00) caused mostly by cooking and laundry time.
Daily DHW and freshwater flow rate demand
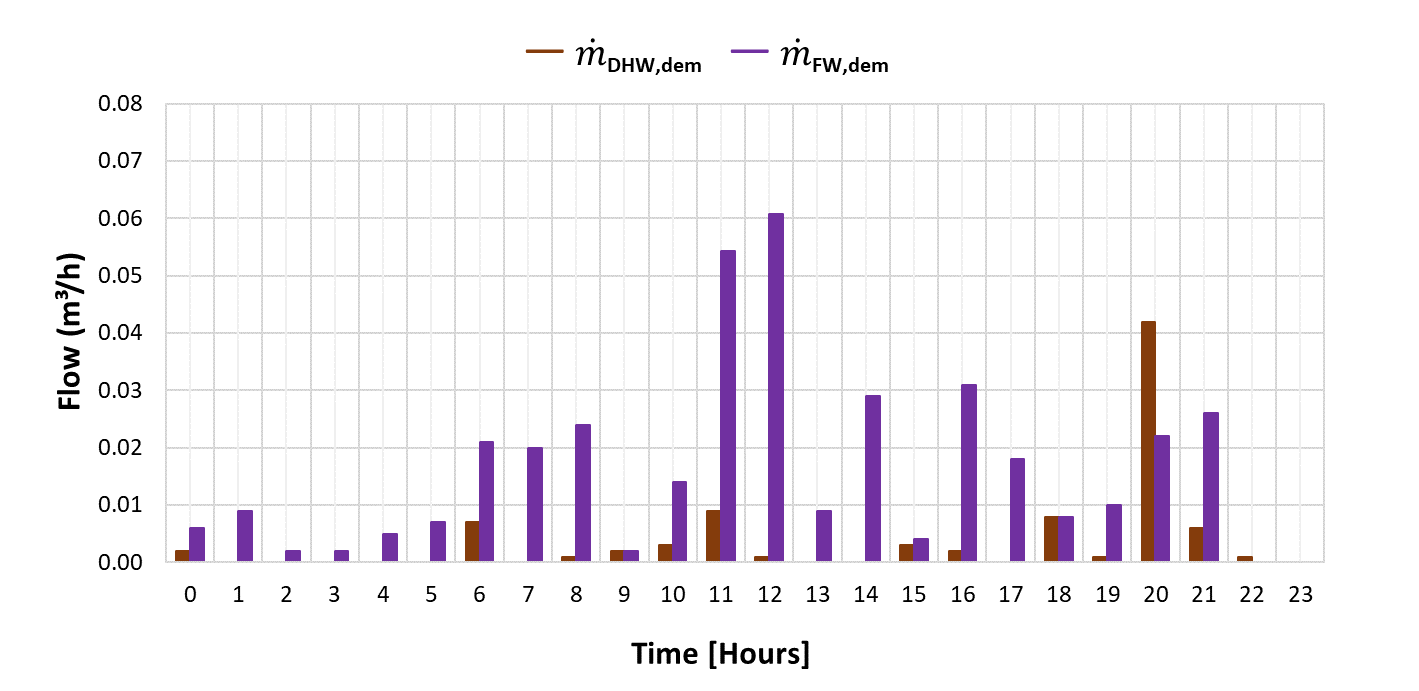
Regarding the weekly demand profile, Figure 9 shows the electrical power consumption for the first week of January. The electricity is the only demand highlighted in this section due to its dissimilar behavior between weekday and weekend. The week ranges from 0h to 120h and displays five similar demand patterns, one for each day of the week, as discussed in the previous section. Conversely, the weekend (121h to 168h) presents a very different consumption profile. The electricity is used more scattered during the day due to a higher dwelling occupancy and a greater peak is observed between 12:00 and 13:00 (cooking time).
Weekly electrical power demand
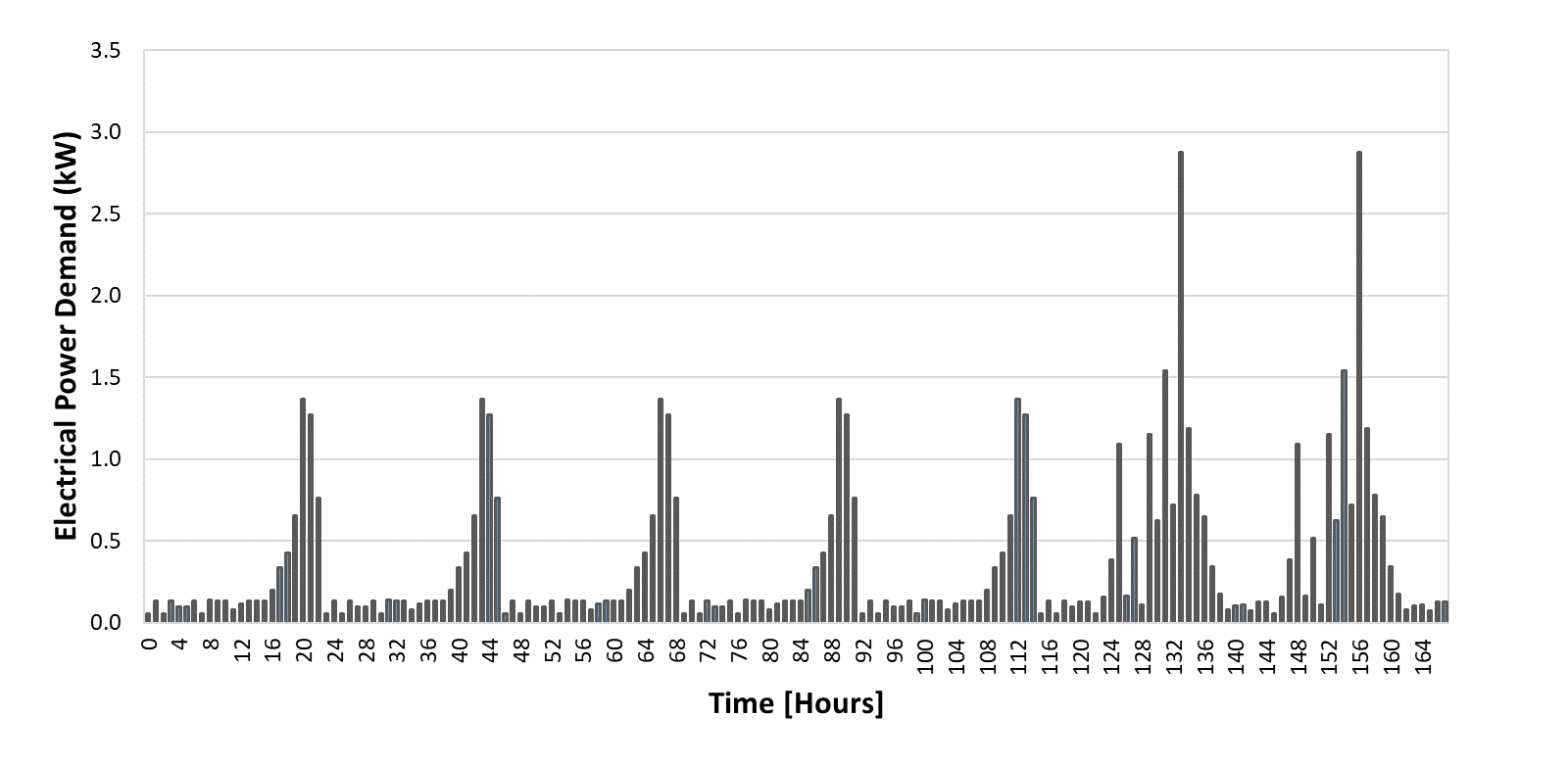
In this section, the yearly demand pattern of heating and cooling (Figure 10), electricity (), DHW (Figure 12) and freshwater (Figure 13) are displayed with 1-hour time step. In addition, the annual consumption is calculated through a 1-year integration period (form 0h to 8760h). The results of the overall amount of each demand are summarized in Table 5. All yearly demands have been previously validated based on the approach used to generate them.
Yearly results
Parameter |
Symbol |
Value |
Unit |
Electricity building demand |
PEl,dem |
3,866 |
kWh/yr |
DHW building flow demand |
DHW ,dem |
41 |
m3/yr |
DHW building thermal demand |
QDHW,dem |
2,090 |
kWh/yr |
Freshwater building demand |
FW,dem |
110 |
m3/yr |
Cooling building demand |
QCool,dem |
1,450 |
kWh/yr |
Heating building demand |
QHeat,dem |
941 |
kWh/yr |
Figure 10 shows the yearly profile of heating and cooling demand. In particular, the cooling season ranges from June to September and the heating season from October to May. The annual cooling peak (5.05 kW) happens at 18:00 on August 19th, whereas the annual heating peak (3.09 kW) occur at 09:00 on January 8th. The building energy demand of space cooling, QCool,dem, is 1,450 kWh/yr which is higher than the space heating demand QHeat,dem, (941 kWh/yr) due to the local weather condition and the setpoint temperature defined by the BD-ES. Nevertheless, the building heating and cooling demands satisfy the BD-ES threshold of 1,650 kWh/yr and 2,200 kWh/yr for heating and cooling demands, respectively.
Yearly heating and cooling demand
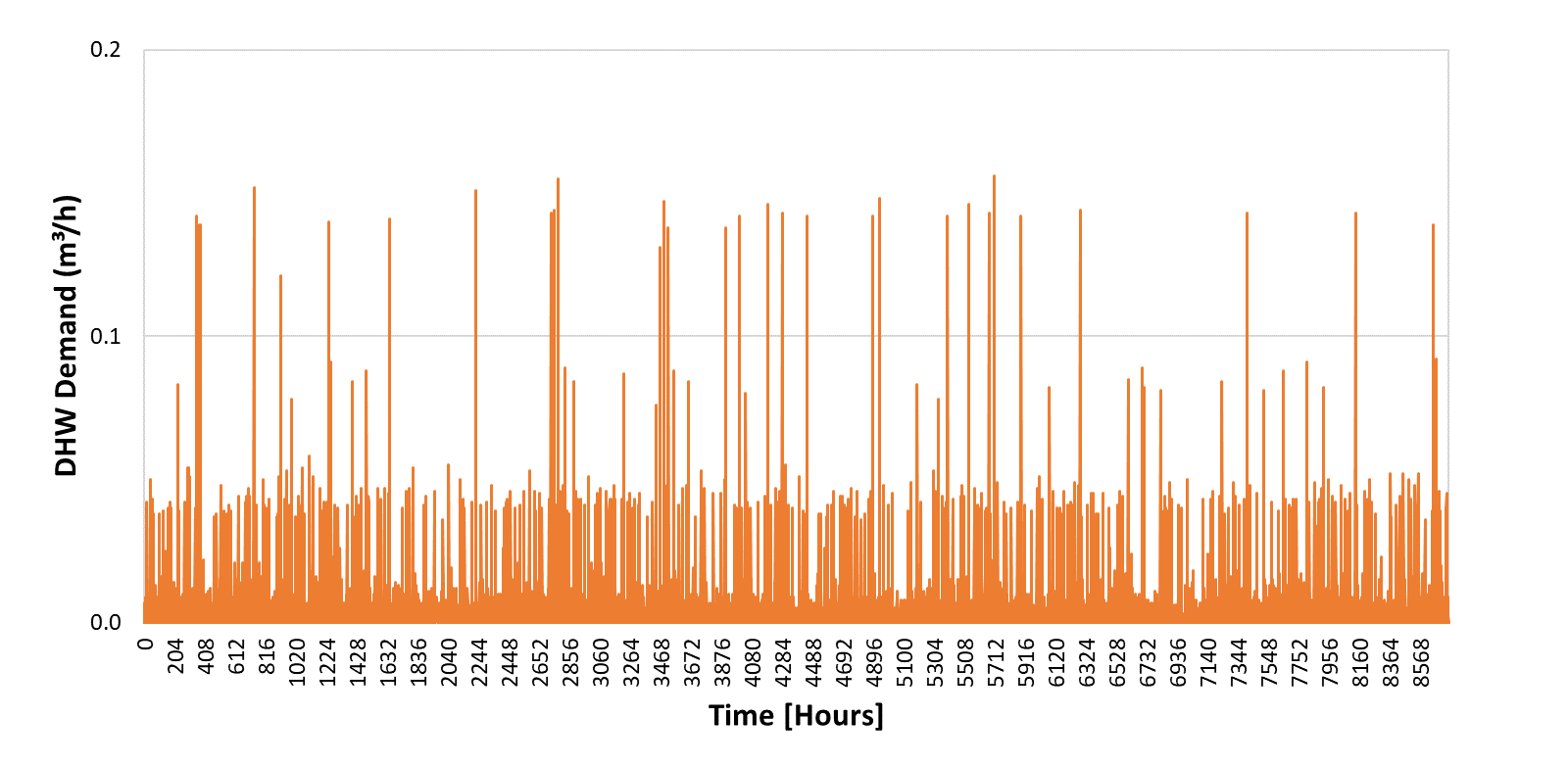
Yearly electrical power consumption can be seen at . It is important to note that weekly patterns remain constant into a specific month of the year. Spring and autumn seasons present lower difference between weekday and weekend profiles due to the mild temperature of the seasons and more outdoor activities. Summer and winter show higher consumption during the weekend due to higher occupancy and more appliances use. The greater power demands were registered in July and December and an electrical power peak of 3.16 kW was found. In this simulation, October showed the least power consumption along the year. The building electrical power load is due to appliances and lighting use exclusively.
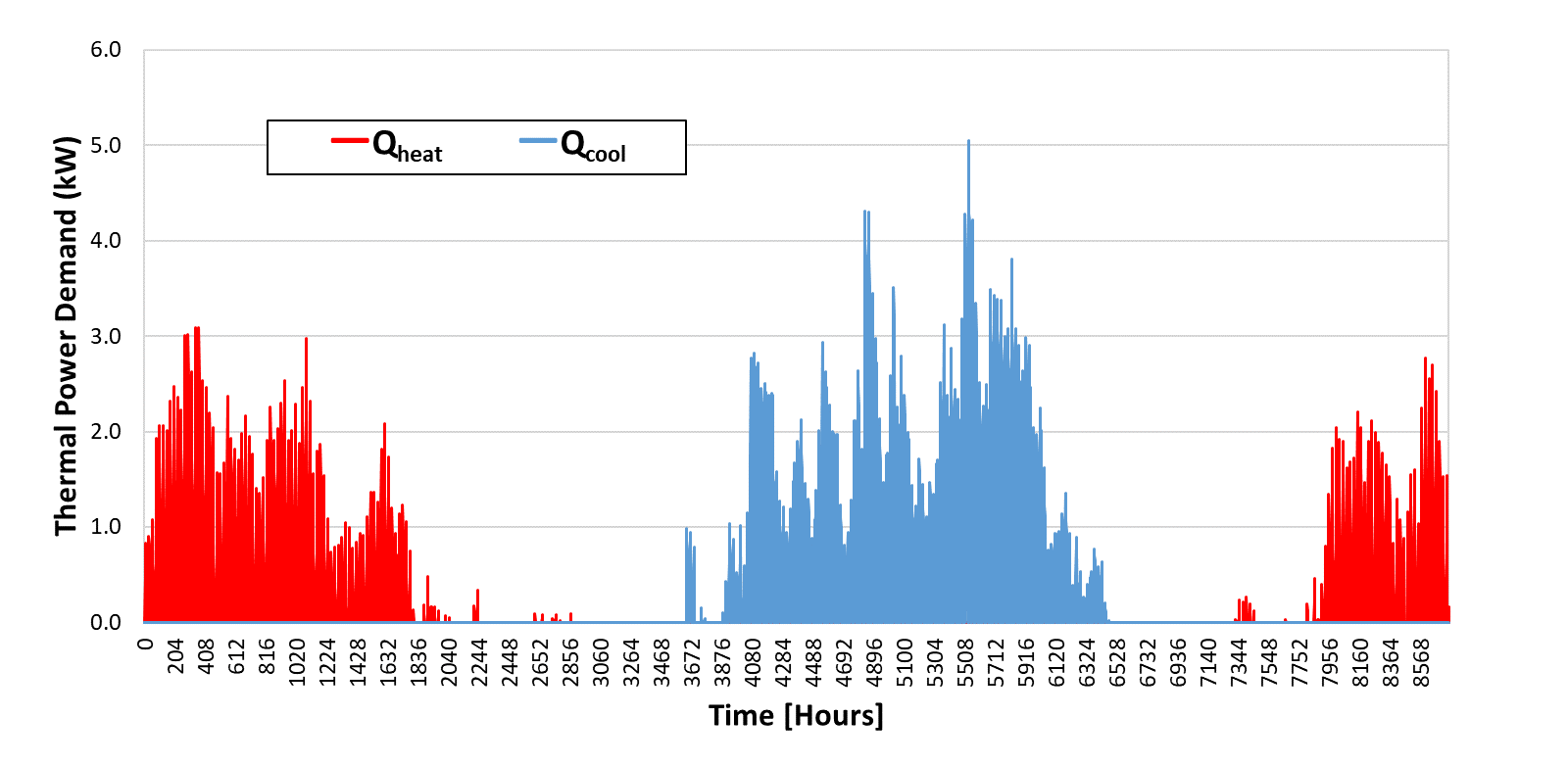
Figure Yearly electricity demand
and Figure 13 display the water consumption profile regarding DHW and freshwater, respectively. Both figures show a pattern with lesser season influence all year long because of the constant user need. DHW demand presents a peak of 0.16 m³/h once it considers only a shower. On the other hand, the freshwater consumption can achieve a peak of 0.23 m³/h due to all other building fixtures usage at the same time. Furthermore, the scattered nature of the figures coincide with the distribution of draw offs according to its probability function, duration and time step along the year.
Yearly DHW demand
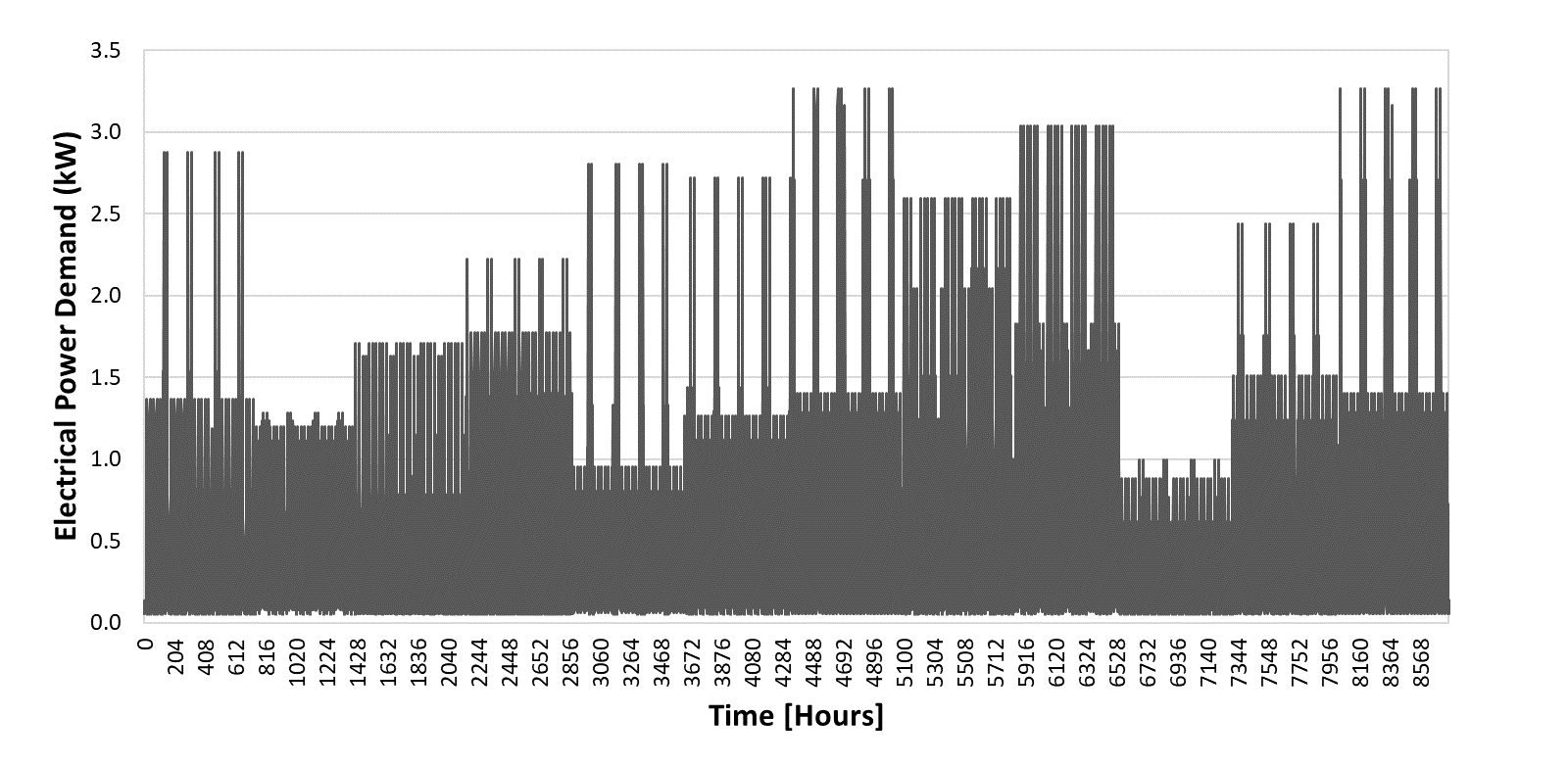
Yearly freshwater demand
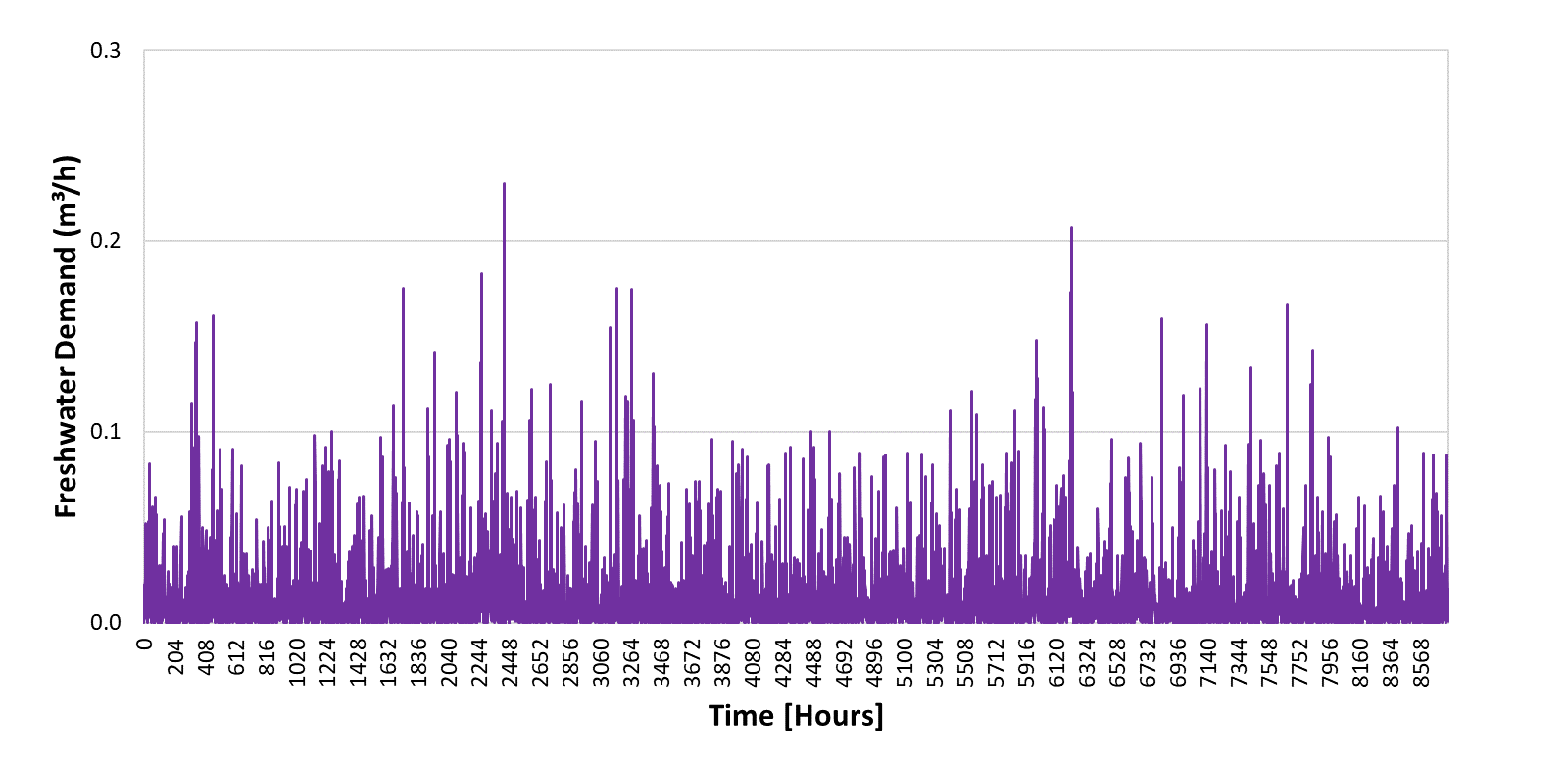
First, the building thermal comfort was analyzed without an indoor temperature control, as it can be seen at Figure 14. In this particular case, PMV index ranges from -2 to -3 in the winter season, which is associated to a too cold sensation. In contrast, during the summer, the same index varies up to +2, corresponding to a hot sensation. The PMV is directly related to the PPD index, which establishes the level of building users dissatisfaction. Thus, during the winter 100% of the users are dissatisfied in some occasions, whereas in the summer this dissatisfied percentage varies from 10% to 80%.
Thermal comfort analysis without temperature control
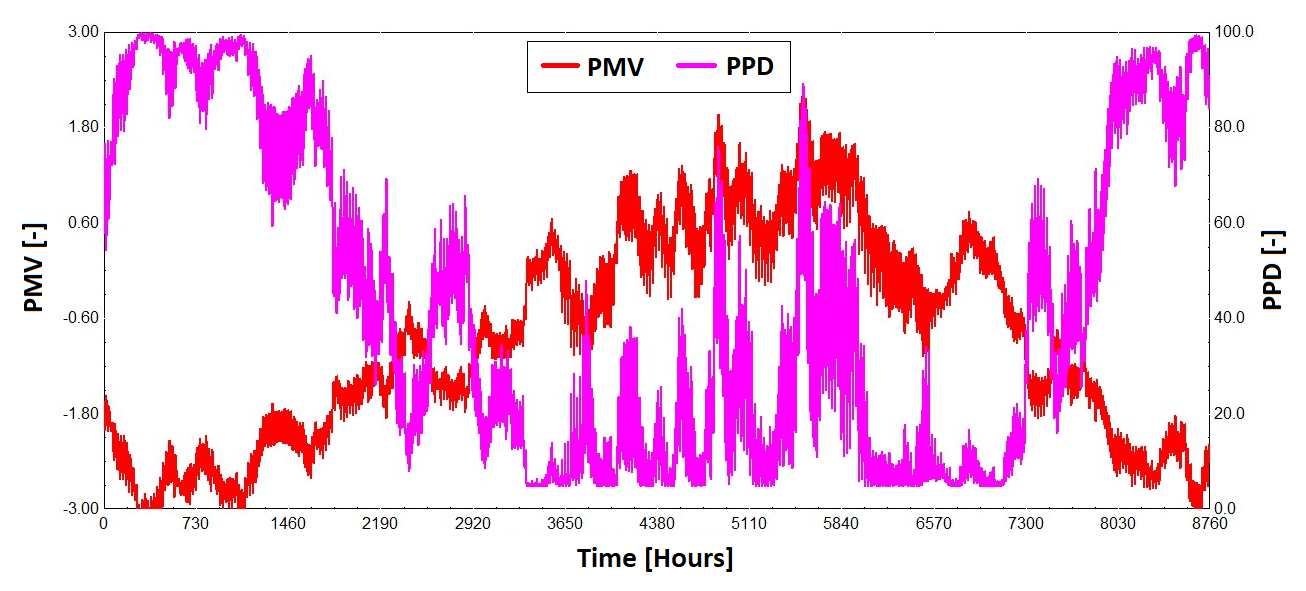
In order to validate the thermal comfort achieved by the setpoint temperature defined in the BD-ES, another analysis was performed. Figure 15 shows the PMV and PPD indexes. In particular, PMV was considerably improved during summer season, achieving a neutral sensation most of the time and, consequently, reaching up to 90% occupant satisfaction rate. Nevertheless, in the winter, a slightly improvement can be noticed, therefore a cold sensation remains and the level of dissatisfaction is around 80% of the building occupants. According to ASHRAE 55 [40], thermal comfort can be achieved based on 80% occupant satisfaction rate or more. Therefore, applying the BD-ES to the reference case presented in this work, the thermal comfort can only be achieved during the summer season.
Thermal comfort analysis with temperature control
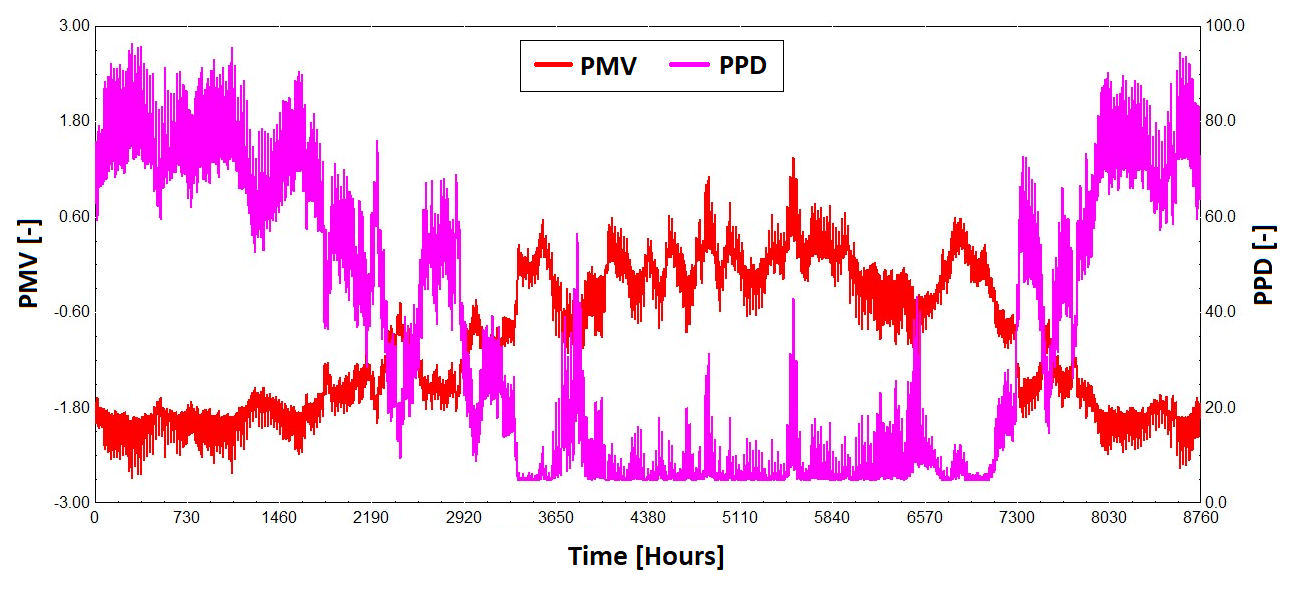
The PES applied to the RS was calculated according to Equation 3. shows the PESRS breakdown according to the thermal, electrical and freshwater primary energy consumption, as well as the total PESRS.
Table Primary Energy Saving breakdown
Demand |
Value [k Wh/ yr ] |
PECth |
4,556 |
PECel |
8,404 |
PECFW |
956 |
PES R S |
13,917 |
PESRS achieves up to 13,917 kWh/yr for the reference case. It represents a high potential for polygeneration systems application and renewable energy sources usage in the residential sector. Furthermore, the reference case was evaluated according to the maximum non-renewable primary energy consumption (PECth,max) defined by the BD-ES. For that reason, a thermal primary energy consumption (PECth) was calculated aggregating all thermal demands (heating, cooling and DHW). In particular, PECth amounts to 4,556 KWh/yr and meets the threshold of 5,400 KWh/yr established by the BD-ES.
Generic patterns for all energy demands required by the residential sector, in particular a single-family townhouse located at the Mediterranean European side, are presented. Electricity, domestic hot water and fresh water demands were estimated according to parametric algorithms reported in the literature. Whereas, heating and cooling profiles were obtained through a TRNSYS building simulation. All approaches have been previously validated based on statistical and/or experimental data.
Simulations provided reliable data with satisfactory resolution time step along 1-year period. The results were presented in terms of daily, weekly and yearly proï¬les. Moreover, an annual integration was adopted for all energy demands. The outcomes of the developed study fill a lack of information about residential sector demands found in the literature.
In addition, thermal comfort and primary energy saving analysis were performed to validate the reference case against the Spanish normative. The thermal comfort can only be achieved during the summer season. A high percentage of user dissatisfaction was found in the winter. It can be concluded that the BD-ES setpoint temperature assumed for the winter is inadequate to provide thermal comfort satisfaction. PES confirmed the high potential of the residential sector as one of the most significant energy consumers. Furthermore, the maximum non-renewable primary energy consumption defined by the BD-ES was accomplished.
This study can be useful for technicians and policy makers dealing with demand side management as well as polygeneration systems design. The generic energy demand profiles are fully reliable and suitable for realistic simulations in order to integrate and exploit the use of different polygeneration configurations applied to the residential sector. Furthermore, future developments of this work will include a comprehensive analysis of a polygeneration system to supply the reference case demands.
L |
Energy loss to the environment |
[W/m²] |
M |
Metabolic Rate |
[W/m²] |
Mass flow |
[Kg/s] |
|
Q |
Heat |
[KWh] |
|
||
ϵ |
Specific Consumption |
[kWh/m³] |
𜂠|
Efficiency |
- |
|
||
Cool |
Cooling |
|
Dem |
Demand |
|
El |
Electrical |
|
FW |
Freshwater |
|
Heat |
Heating |
|
Th |
Thermal |
|
Max |
Maximum |
|
|
||
AC |
Air-conditioning |
|
BES |
Building Energy Simulation |
|
CFD |
Computational Fluid Dynamics |
|
COP |
Coefficient of Performance |
|
CREST |
Centre for Renewable Energy Systems Technology |
|
CTE |
Technical Construction Code |
|
DB-SE |
Basic Document on Energy Savings |
|
DHW |
Domestic Hot Water |
|
EU |
European Union |
|
GB |
Gas Boiler |
|
IEA-SHC |
International Energy Agency - Solar Heating and Cooling |
|
ISO |
International Organization for Standardization |
|
PEC |
Primary Energy Consumption |
|
PES |
Primary Energy Saving |
|
PMV |
Predicted Mean Vote |
|
PPD |
Predicted Percentage of Dissatisfied |
|
RS |
Reference System |
|
STREAM |
Stochastic Residential Water and Use Model |
|
TRNSYS |
Transient Systems Simulation |
Building operation and energy performance: Monitoring, analysis and optimisation toolkit ,Applied Energy , Vol. 101 ,pp 310-316 , 2013, https://doi.org/10.1016/j.apenergy.2011.10.037
, Energy performance assessment of existing dwellings ,Energy and Buildings , Vol. 39 (4),pp 393-403 , 2007, https://doi.org/10.1016/j.enbuild.2006.08.008
, - , A strategy for smart, sustainable and inclusive growth, 2010
- ,
Chapter 5: Increasing Efficiency of Building Systems and Technologies ,pp 39 , Polygeneration and efficient use of natural resources ,Energy , Vol. 34 (5),pp 575-586 , 2009, https://doi.org/10.1016/j.energy.2008.08.013
, Simulation of polygeneration systems ,Energy , Vol. 163 ,pp 290-337 , 2018, https://doi.org/10.1016/j.energy.2018.08.052
, Integration of a photovoltaic system with an electric heat pump and electrical energy storage serving an office building , 2019
, Dynamic Simulation of a Trigeneration Scheme for Domestic Purposes Based on Hybrid Techniques ,Energies , Vol. 9 (12),pp 1013 , 2016, https://doi.org/10.3390/en9121013
, The choice of operating strategy for a complex polygeneration system: A case study for a residential building in Italy ,Energy Conversion and Management , Vol. 163 ,pp 278-291 , 2018, https://doi.org/10.1016/j.enconman.2018.02.066
, Analysis of a domestic trigeneration scheme with hybrid renewable energy sources and desalting techniques ,Journal of Cleaner Production , Vol. 212 ,pp 1409-1422 , 2019, https://doi.org/10.1016/j.jclepro.2018.12.006
, Energy and economic analysis of geothermal–solar trigeneration systems: A case study for a hotel building in Ischia ,Applied Energy , Vol. 138 ,pp 224-241 , 2015, https://doi.org/10.1016/j.apenergy.2014.10.076
, A novel solar-assisted heat pump driven by photovoltaic/thermal collectors: Dynamic simulation and thermoeconomic optimization ,Energy , Vol. 95 ,pp 346-366 , 2016, https://doi.org/10.1016/j.energy.2015.11.071
, Dynamic simulation of a solar heating and cooling system for an office building located in Southern Italy ,Applied Thermal Engineering , Vol. 103 ,pp 377-390 , 2016, https://doi.org/10.1016/j.applthermaleng.2016.04.094
, Thermoeconomic analysis and optimization of high efficiency solar heating and cooling systems for different Italian school buildings and climates ,Energy and Buildings , Vol. 42 (7),pp 992-1003 , 2010, https://doi.org/10.1016/j.enbuild.2010.01.011
, - Relatório sobre a estratégia da UE para o Aquecimento e Refrigeração
State of the art in building modelling and energy performances prediction: A review ,Renewable and Sustainable Energy Reviews , Vol. 23 ,pp 272-288 , 2013, https://doi.org/10.1016/j.rser.2013.03.004
, - , , Global sensitivity analysis: the primer, 2008
A review of methods to match building energy simulation models to measured data ,Renewable and Sustainable Energy Reviews , Vol. 37 ,pp 123-141 , 2014, https://doi.org/10.1016/j.rser.2014.05.007
, IEA EBC annex 53: Total energy use in buildings—Analysis and evaluation methods ,Energy and Buildings , Vol. 152 ,pp 124-136 , 2017, https://doi.org/10.1016/j.enbuild.2017.07.038
, Measured and predicted energy demand of a low energy building: important aspects when using Building Energy Simulation ,Building Services Engineering Research and Technology , Vol. 28 (3),pp 223-235 , 2007, https://doi.org/10.1177/0143624407077393
, Domestic electricity use: A high-resolution energy demand model ,Energy and Buildings , Vol. 42 (10),pp 1878-1887 , 2010, https://doi.org/10.1016/j.enbuild.2010.05.023
, - , Thermoeconomic and environmental analyses for the synthesis of polygeneration systems in the residential-commercial sector, 2011
Assessment of solar radiation components in Brazil using the BRL model ,Renewable Energy , Vol. 108 ,pp 569-580 , 2017, https://doi.org/10.1016/j.renene.2017.02.077
, - , , TRNSYS: A Transient System Simulation Program, 2006
TRNSYS – Features and functionalitity for building simulation 2009 ,Conference ,pp 5 , 2009
, A review of micro combined heat and power systems for residential applications ,Renewable and Sustainable Energy Reviews , Vol. 64 ,pp 144-162 , 2016, https://doi.org/10.1016/j.rser.2016.04.064
, - , , OpenStudio plugin for Google SketchUp3D, 2009
- , , Google SketchUp is a registered trademark, 2009
- , Basic Document Energy Saving, 2017
- , Basic Document of Health, 2019
- , Caracterización de la ventilación en la edificación residencial existente. Conciliación entre calidad del aire interior y eficiencia en la rehabilitación energética, 2016
Tool for the Generation of Domestic Hot Water (DHW) Profiles on a Statistical Basis Version 2.02b (March 2017) ,pp 22 ,
, Analysis of a Residential Photovoltaic-Thermal (PVT) System in Two Similar Climate Conditions ,Energies , Vol. 12 (19),pp 3595 , 2019, https://doi.org/10.3390/en12193595
, - ,
Influence of the DHW load profile on the fractional energy savings: a case study of a solar Combi-system with TRNSYS simulations ,Fuel and Energy Abstracts , Vol. 43 (3),pp 200 , 2002, https://doi.org/10.1016/S0140-6701(02)85844-1 - , Documento Básico de Ahorro de Energía, 2017
Implications of data sampling resolution on water use simulation, end-use disaggregation, and demand management ,Environmental Modelling & Software , Vol. 102 ,pp 199-212 , 2018, https://doi.org/10.1016/j.envsoft.2017.11.022
, Developing a stochastic simulation model for the generation of residential water end-use demand time series ,pp 9 ,
, - , Moderate thermal environments — Determination of the PMV and PPD indices and specification of the conditions for thermal comfort
- , , Thermal comfort. Analysis and applications in environmental engineering, 1970
- , Standard 55- Thermal Environmental Conditions for Human Occupancy, 2013
A novel tool for thermoeconomic analysis and optimization of trigeneration systems: A case study for a hospital building in Italy ,Energy , Vol. 126 ,pp 64-87 , 2017, https://doi.org/10.1016/j.energy.2017.03.010
, A novel solar-geothermal district heating, cooling and domestic hot water system: Dynamic simulation and energy-economic analysis ,Energy , Vol. 141 ,pp 2652-2669 , 2017, https://doi.org/10.1016/j.energy.2017.08.084
, Analyzing the Energy Consumption, GHG Emission, and Cost of Seawater Desalination in China ,Energies , Vol. 12 (3),pp 463 , 2019, https://doi.org/10.3390/en12030463
,