Transportation is among the biggest emission-intensive sectors in Europe, with greenhouse gas emissions from transportation continuing to rise, thereby challenging the overall EU climate targets [1]. Since the energy consumption for transportation heavily depends on fossil fuels, using Electric Vehicles (EVs) can help decrease emission levels [2]. Moreover, with the trends encouraged by governments and political parties, strong commitments have been made on an international level to increase the penetration of Renewable Energy Sources (RES) [3]. Several papers in the literature address the possibility of using electric vehicles as a solution to fully take advantage of RES by shaping the load demand curve [4], [5].
Statistically, passenger vehicles stay parked for up to 96% of the day and their idle time is much longer than the time required to fully recharge their batteries [6]. Hence, acting as an environmentally and economically friendly choice for transportation and energy management, EVs can be used both as mobile energy storage units and as generators to support building energy demand [7].
Furthermore, with 30% of the end-use energy-related carbon emission coming from building energy consumption, including commercial and residential buildings, a reduction of the energy drawn from the grid by 39% compared to unoptimised energy management schemes as showcased in [8] is considered of great importance. As such, EVs can help increase power supply reliability and reduce energy peaks in demand from the grid via vehicle-to-building (V2B) operation mode [9]. For this purpose, EVs must be equipped with vehicle-to-grid (V2G) communication systems [10]. One can study the management of EV charging as an optimization problem, where the objective is to maximize the usage of energy produced by renewable sources or to minimize the environmental impact in terms of CO2 emission.
However, there are some constraints to be considered, such as battery capacity, maximum charging/discharging power, state of charge at arrival time, desired state of charge at departure, user preferences (priority charge, eco-friendly charge, and economical charge), and amount of power available from the grid or RES.
The purposes of this work are (a) to assess the potential of Electric Vehicle technology adaption in countries with relatively low EV penetration levels, (b) to investigate the energy efficiency potential of microgrids (which include EVs with V2G capability, photovoltaic and storage systems, and charging stations), and (c) to set the basis for the development of an integrated energy management system governing the whole spectrum of the processes. In particular, this research aims to design and develop an algorithm that optimizes the integration of electric vehicles into a general energy management system while calibrating relevant parameters to avoid any discomfort and annoyance caused in people's everyday living and the grid's operation.
According to the European Parliament official briefing [11], the European Union has set new targets for renewable energy use. The targets are expected to be partially met by both regular energy consumers and consumers who produce energy (introduction of the term "prosumers"). Since 2015, the European Commission has set a vision for an Energy Union with citizens lying at the center, taking advantage of new technologies to reduce their bills [12].
Prosumers investing in renewable energy technologies could help the EU finance the transition to the energy union, increase the share of renewable energy (RES) and, under certain conditions, reduce energy costs, dramatically changing the energy system. At the same time, by generating their own electricity, prosumers could reduce their demand from the grid and so their bills, benefit from a sense of empowerment, and help introduce elements of "energy democracy" [13]. This reduced demand and the ability to sell surplus electricity from energy storage devices create added value in the market, including the electricity system's balance enhancement for which customers could be compensated.
This decision-making capability of selling surplus energy puts prosumers at the center of the research interest. Some other projects propose using electric vehicle batteries as energy storage. One could charge vehicles at lower demand (and lower energy pricing) periods, with the energy being sold back to the grid at higher demand (and energy pricing) periods. This vehicle-grid (V2G) idea is already operating on a small scale in the United Kingdom, as presented below [14].
Other research works have proposed and integrated EV charging stations with PV generation to help lower costs and reduce the carbon footprint. Many optimization or control algorithms, including ordinal optimization [15], genetic algorithm [16], and model predictive control approaches [17], have been proposed to deal with the inelasticity of demand and performance variability of renewable energy sources during the day. For example, EV charging cost, as a convex function of load demand, is minimized by Tang and Zhang [18]. Although a lot of work aims at reducing the complexity of the optimization algorithms for the EV charging process, e.g., by sacrificing the minimum performance gap, substantial time is still required to compute and obtain the optimal results.
The increasing complexity of power grids due to the introduction of renewable energy sources, the flourishing energy demand due to the introduction of EVs, and the greater distribution of power elements justify the need for a shift from conventional to smart grids. The main objectives of smart grids are to provide (a) operational efficiency with distributed energy generation, network optimization, and improved assets utilization, (b) energy efficiency, (c) customer satisfaction, and (d) CO2 emission reduction. As a supply network, the grid can be considered a complex system of systems that aims to ensure a reliable power supply to all its customers. Nowadays, the dependency on the centralized power system is changing through progressive replacement by smaller and more distributed generations closer to loads to meet their requirements effectively [19].
Microgrids are small-scale supply networks designed to provide electrical and heat loads in a small community, such as a housing estate, a suburban locality, an academic or a public community, an industrial site, or a commercial area. A theoretical framework has been established and applied in a microgrid layout case scenario to analyse the energy scheme proposed in this work.
Energy management at a microgrid level appears to be a subject at a high level of complexity due to the integration of several systems, i.e., PV and other renewable energy sources, EVs, and varying needs and requirements of the users. The proposed method considers these interrelationships and aims to maximize energy efficiency and microgrid sustainability. More specifically, it integrates optimization algorithms (genetic algorithms) with multicriteria decision-making techniques (Analytic Hierarchy Process − AHP) to develop a robust optimization tool to unravel multiple and conflicting objectives.
The proposed process consists of four stages: (1) microgrid operational assessment, (2) AHP decision criteria weighting, (3) Genetic Algorithm optimization, and (4) Energy management scheme selection. Figure 1 provides a schematic representation of the proposed approach. In the first stage, the microgrid characteristics (energy demand, demand fluctuation during the day) and assets (ESS, PVs, EVs) are analysed, and alternative microgrid operation schemes are presented. Further, the AHP parameters/criteria are determined, and a decision-making hierarchy is developed. In particular, factors of "relative importance" are assigned to the decision-making parameters (based on pairwise comparisons), and weights are calculated for the decision parameters to make up the objective function. A set of constraints are identified to supplement the optimization model.
The next step includes the iterative processes proposed by the genetic algorithms' methodology. The initial population is selected, the problem limitations are set, and the genetic operators are quantified. In the last step, the selected energy scheme is checked and proposed for adoption by each user. A user can approve the proposed energy management scheme based on his/her schedule and regardless of the choice of other users. If approved, the system will be automatically authorized to manage the microgrid. While if any of the users reject the proposed scheme, then the system returns to stage 3 of the procedure, recalculates the scheme for the users that didn't approve it, and runs the process through the last stage for approval.
The proposed approach focuses on integrating EVs as a viable alternative energy resource in a microgrid that can support other existing resources, such as PV installations and batteries installed in situ, to provide sustainable and efficient energy management within the microgrid.
Schematic representation of the proposed Energy Management Scheme selection
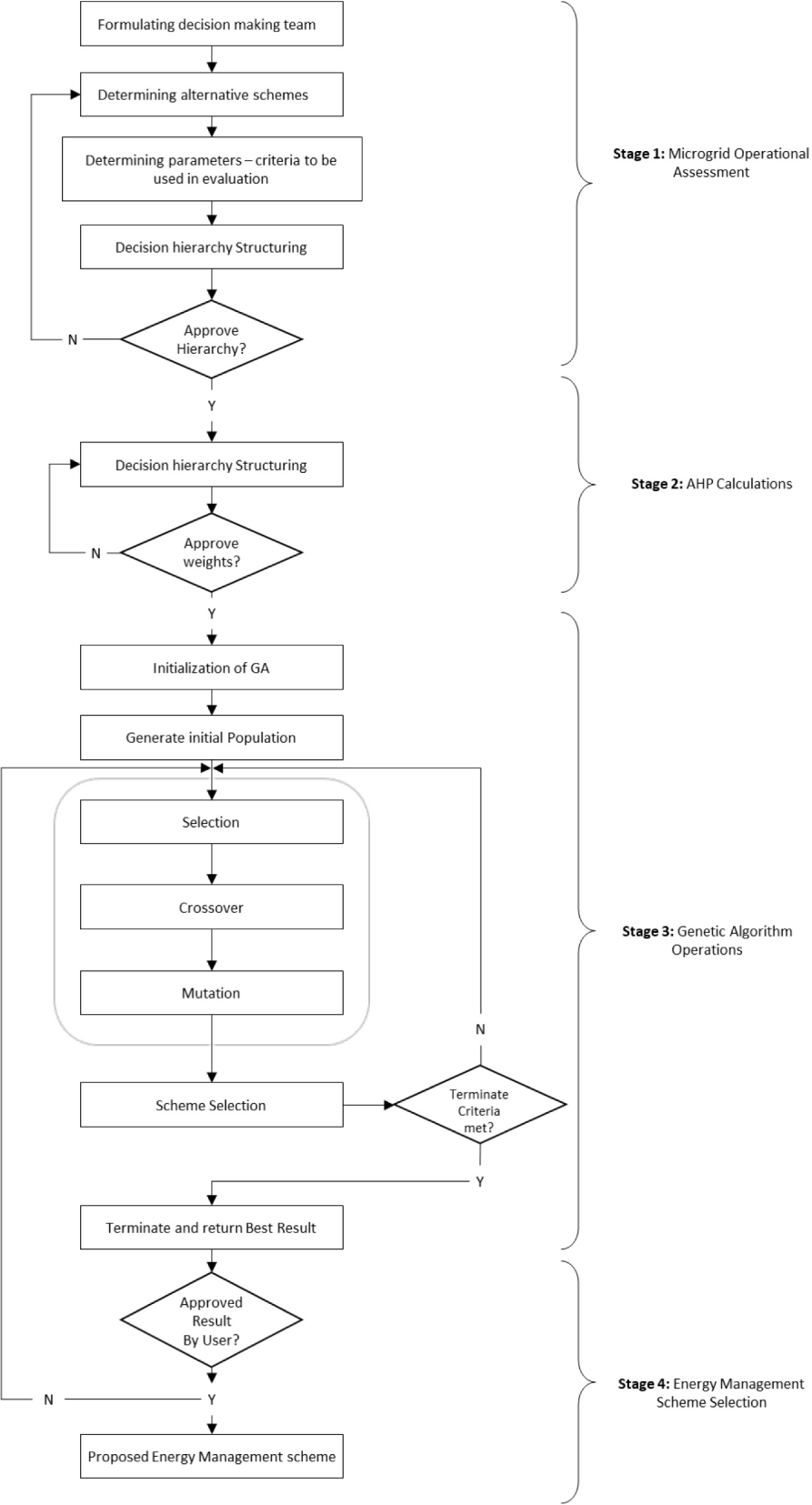
Key microgrid features include its assets and architecture, the available or desirable resources it may have, and the specifications concerning Electric Mobility and Vehicle-to-Grid (V2G) technology. Based on the overview of these features and the assessment of the field research analysis results, three alternative energy schemes (Alternative Energy Scheme − AES) are developed:
AES-1: Grid – Battery Installation (home & work),
AES-2: Grid – Battery Installation (home & work) – PV Installation (Work),
AES-3: Grid – Battery Installation (home & work) – PV Installation (Work) – Electric Vehicles (V2G).
These alternatives represent the current state (AES-1), a future state with the installation of RES systems (AES-2), and a future state based on the integration of both RES systems and EVs (AES-3).
The main aim of the analysis is to assess the energy scheme that results in resource exploitation in a sustainable and economically viable way. In this direction, four parameters (criteria) are considered to contribute toward these objectives: grid energy cost, utilization of renewable energy sources, use of energy storage elements, and balanced energy demand. The decision-making process is based on a hierarchy structure consisting of four levels, as presented in Figure 2, that depicts the interconnections between the different elements of each layer. The overall goal of the proposed approach lies at the top level. The decision criteria and sub-criteria are visible in the second and third levels. The level of sub-criteria can be recalibrated and include the corresponding data of each case study. The alternative energy management schemes lie on the fourth level of the hierarchy.
Decision-making hierarchy structure
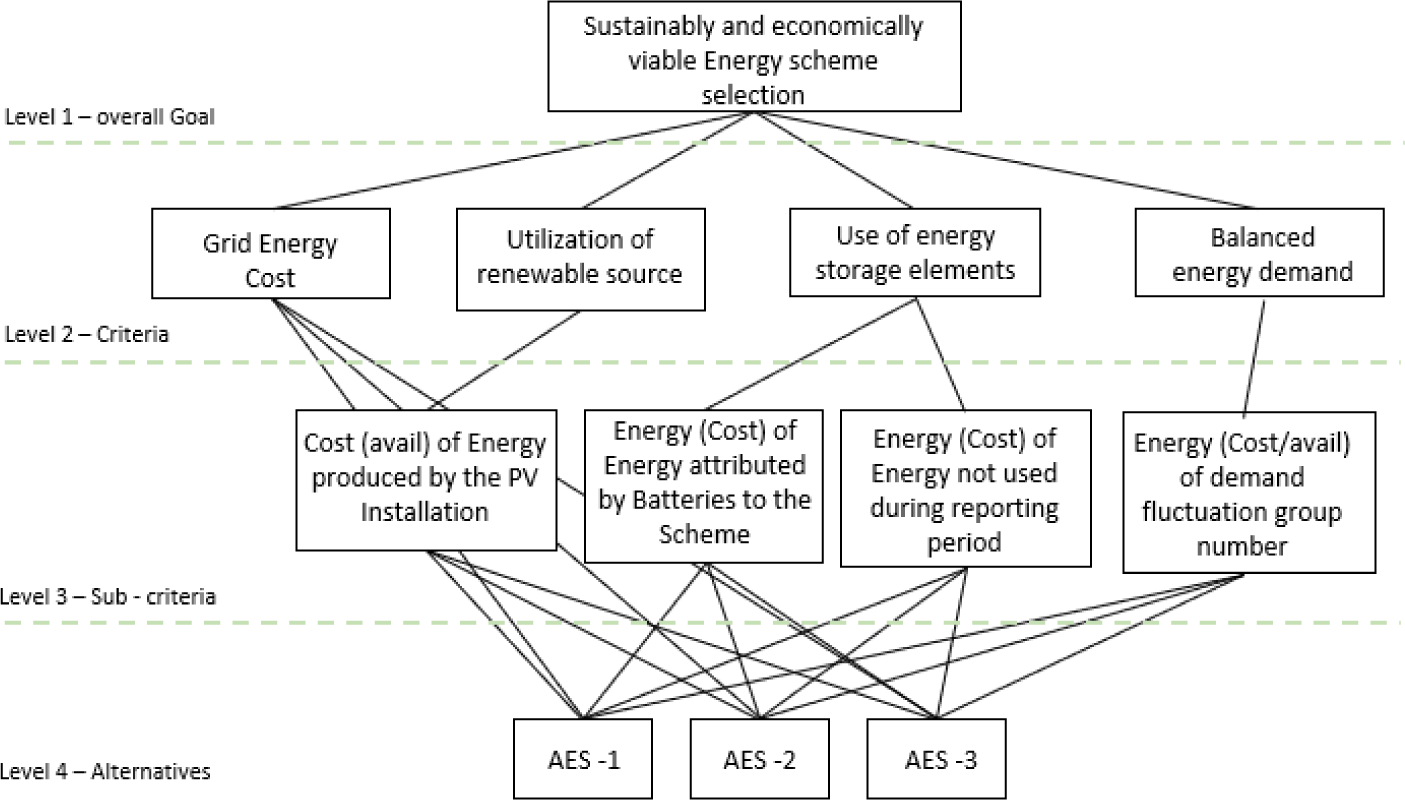
The former hierarchy provides AHP with input for assigning weights toward shaping the objective function, which will govern the Energy scheme selection in the following stages. The AHP is developed with the use of a pairwise comparison matrix. Table 1 presents the results from the criteria comparison based on a scale of importance and the values geometric means.
Pairwise comparison matrix and criteria Weights
Grid Energy Cost |
Utilization of renewable sources |
Use of energy Storage elements |
Balanced energy demand |
Weight |
|
---|---|---|---|---|---|
Grid Energy Cost |
0.12 |
0.09 |
0.25 |
0.12 |
14.4% |
Utilization of renewable sources |
0.35 |
0.26 |
0.25 |
0.24 |
27.6% |
Use of energy Storage elements |
0.06 |
0.13 |
0.13 |
0.16 |
11.9% |
Balanced energy demand |
0.47 |
0.52 |
0.38 |
0.48 |
46.2% |
The weights resulting from the AHP calculation are presented in Table 2, along with the calculations related to the consistency ratio (CR), which is 0.065 < 0.1. One can safely conclude that the assigned weights are consistent and can be approved and proceed to the next stages of the process. Based on the assessment, the balanced energy demand and the utilization of renewable sources appear to be the most important criteria, which complies with the logic supporting the approach and increases the likelihood of achieving the overall goal.
Results from AHP Calculations
Criterion |
Weight |
λmax, CI, RI |
CR |
---|---|---|---|
Grid Energy Cost |
0.144 |
λmax = 4.157 |
CR = 0.065 |
Utilization of renewable sources |
0.276 |
CI = 0.0525 |
|
Use of energy storage elements |
0.119 |
RI = 0.9 |
|
Balanced energy demand |
0.462 |
A set of constraints should supplement the objective function to make the system economically viable and promote sustainability. Because of this problem's complexity and multilevel nature, the objective function is of a polynomial form. It consists of a set of variables considering the different EVs operational scenarios, indicating the state and rate of charge/discharge (−1 for a minimum to −10 for maximum discharging rate, while 1 for a minimum to 10 for maximum charging rate). Although the system is dynamic, it could work on a continuous operation, the algorithm configuration process charging/discharging time is set at a steady time frame. This time frame is selected to ensure the orderly operation of the bidirectional energy transfer process between the vehicle and the building installation network. Therefore, the optimization algorithm uses a 30 min time frame (period duration) of reference for configuring the power management program.
Time of use, with on-peak cost, vs. conventional energy pricing (PPC pricing modelling)
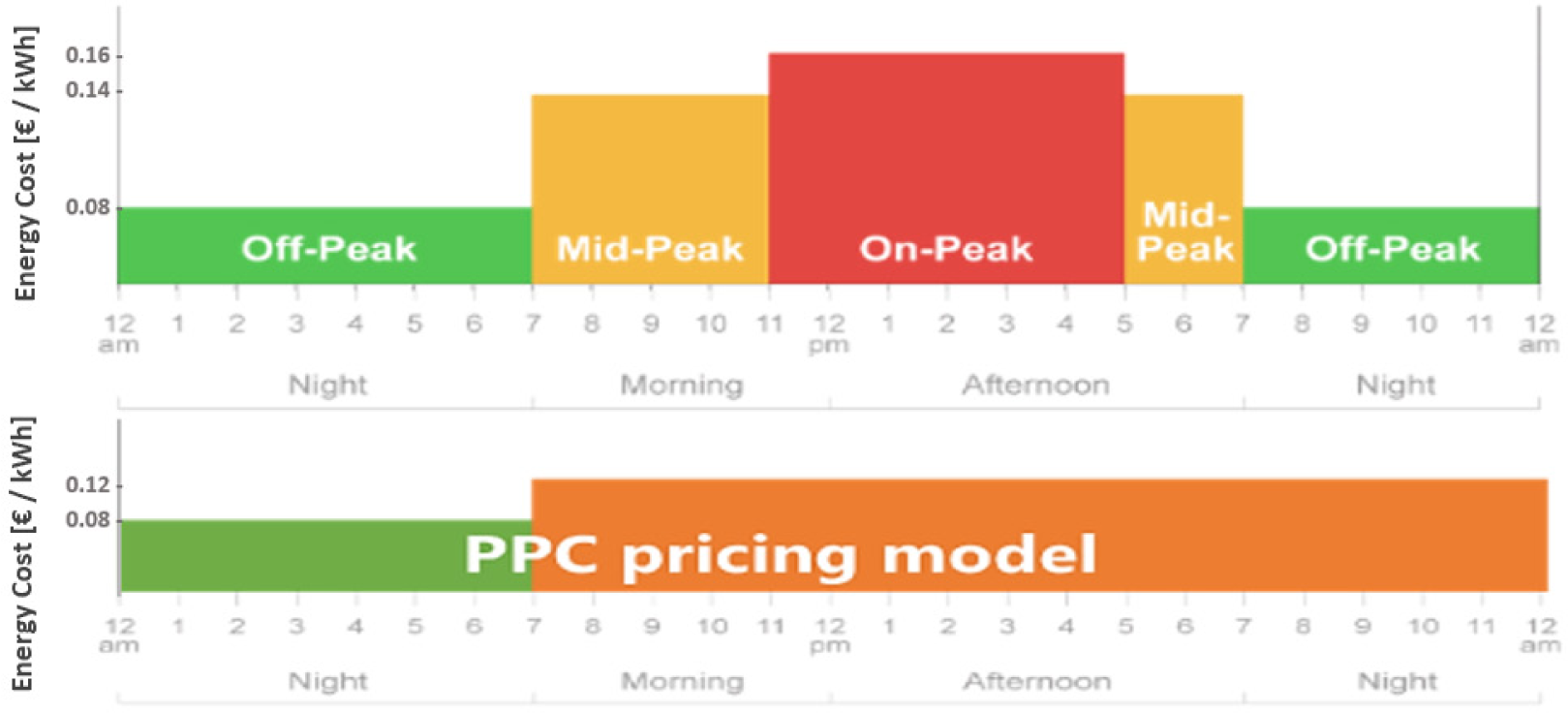
The optimization procedure starts with setting up the decision-making parameters (design) and developing the objective function based on these parameters. The problem constraints are then formed to represent functional relationships among the design variables and other parameters describing physics and resource-based limitations [20]. The proposed optimization tool focuses primarily on sustainability performance. In this respect, it is not only the economic parameters, such as the energy cost per kWh, that solely determine the optimization output, but also the level of demand fluctuation during the day, expressed in periods (which show about the same demand profile) and the energy produced by RES. Although a common measurement unit is required, all parameters are converted into costs (expressed in Euro) based on information gathered from the current energy market.
As mentioned above, the criteria used for AHP calculations represent the objective function decision-making parameters. In each reporting period, calculations are made on energy requirements related to the cost per kWh provided by the Time-of-Use strategy. All parameters of the objective function are being expressed in cost units (based on energy market cost values) to facilitate the following stages of the process. Another reason for choosing a cost unit as an overall measurement unit is the ability to follow the dynamic changes in the energy market, expressed by the cost price of a kilowatt-hour. Based on the AHP calculations, the objective function of the system is described by the following equation:
(1)
Where: CGrid is the grid energy cost [€], BRes is the price benefit from renewable sources utilization [€], BBat is the benefit from managing energy stored in batteries in and out at low and high energy pricing levels from the grid [€], CBat is the cost of the energy attributed by the batteries [€], and the last term is determined using the formula:
(2)
where DNgroups denotes the difference in the number of energy demand groups (groups of periods with common energy demand profile) between the initial state and the state proposed by the energy management scheme. Each of the respected criteria and sub-criteria presented above is further analysed and correlated with energy unit cost.
Grid Energy cost is a straightforward matching of Energy cost based on ToU and the energy demand according to the following formula:
(3)
where Et(i) is the energy demand at period t(i) based on time of use [kWh] and Ct(i) is the energy price at period t(i) [€/kWh]. The utilization of renewable sources applies to the energy surplus created by the photovoltaic installation. This parameter is calculated according to the formula:
(4)
where EPVt(i) is the energy production from PV installation at period t(i) [kWh], and CRes is the price per kWh for energy production (due to lack of dynamic connection with the Energy Market, it is considered equal to the lowest energy cost price CRes =0.08 [€/kWh]). In real life, this value will be linked with the corresponding value provided by the Energy Regulating Authority, based on the period of the day and the type of renewable sources.
The use of storage elements includes energy from either the storage units of an office building (BatESS) or the batteries installed in houses (ΣHBat, corresponding to the house units included for this application) and the EV batteries (ΣEVBat). This criterion is subdivided into two equally weighted sub-criteria, so a weight of 0.500 is assigned to each of them. The equations describing the above are the following:
(5)
(6)
where cdstate(i) is the state of charge or discharge of each battery installed in the scheme, and Ct(i) is the energy price at period t(i)based on time of use [€/kWh].
The second sub-criterion is the cost of the energy stored in the storage elements at the end of the optimization (EBat (t=48) – end of the day as indicated based on the 48 periods of reference and EMS selection period). This energy is considered a cost in the objective function; it will be available for use beyond the examined period of reference. Therefore, the microgrid will not benefit from the unused resources. The said cost corresponds to the following equation:
(7)
where Ct(i) is the energy cost at period t(i) based on time of use [€]
Balanced energy demand and minimized demand fluctuations are important aspects of a sustainable microgrid. Based on the standard deviation in each period of reference, the spread of the Grid Energy Cost is calculated and grouped into respective periods, with energy demand deviating up to 15% from the average value of the respective consumption. The goal is to have fewer groups than the original state and a stable demand profile related to the grid. This parameter is expected to be greatly valued in a fully integrated and dynamic energy market soon. The current research adopts a trade-off of 1/10 of the mean energy cost as the benefit for each reduction of the groups calculated by the proposed procedure.
After selecting the proposed energy management scheme, the next and final stage of user approval follows. Each user, in a real-life scenario, will have to approve the proposed scheme to be adopted by the microgrid. If there is no unanimous approval of the proposed scheme, the procedure must run again. Suppose the users do not approve the proposed scheme unanimously after the second effort. Then, the system continues with the charging/discharging schedules for the users who have approved it and rerun the procedure for those who have not, to meet their needs in the best possible way. If no approval is provided, these users are excluded from the procedure.
A simulation paradigm has been designed to test the proposed method at a first level. Nowadays, there are many countries in Europe where Electric Vehicles already occupy a significant proportion of vehicles on the road, while each country is developing the necessary infrastructure related to the vehicle charging process. Great Britain is one of the model countries in terms of Electric Vehicle integration and the development, support, and adoption of Vehicle-to-Grid (V2G) technology. Many projects have been designed and implemented in the United Kingdom (V2G-Britain, Sciurus, EV-elocity, etc.) to test V2G's efficiency. Among the most important challenges the scientific community faces in this field are the availability of data on the costs and the potential of utilizing and adopting this technology.
Based on research conducted by Cenex, an independent non-governmental organization in the UK [21], a conclusion was that the ECO professionals are among the first people to join a new market, such as the EV market, and adopt new technologies such as V2G. ECO professionals are considered as highly motivated by environmental benefits and likely have PV panels installed on their homes. This archetype is most likely to be met among people of high education, working in academia, or technology companies.
The simulation has been selected to run for a reference territory to achieve a more realistic outcome. It included the city of Patras in Greece for the houses of reference and the University of Patras for the offices/workplaces of reference. The assumption was that each house has a vehicle for daily usage as a second car, a 10 kW photovoltaic system, and a tesla battery as an energy storage system for providing power to recharge the EVs. The allocation of a specific reference area helps provide the simulation with realistic data regarding the daily home-work-home driving distances, drivers' preferences, and microgrid layout.
The University of Patras reference microgrid in this work consists of the following components: a 10-office building with a 10 kW photovoltaic installation, an energy storage system (ESS), four electric vehicle charging stations as well as users' electric vehicles, and the houses, equipped with the appropriate equipment for bidirectional energy transfer and EV charging. Figure 4 displays the microgrid of reference and its components. The specific characteristics used for simulation purposes will be presented, along with the limitations and special preferences proposed for application in the framework.
Application's microgrid of reference layout
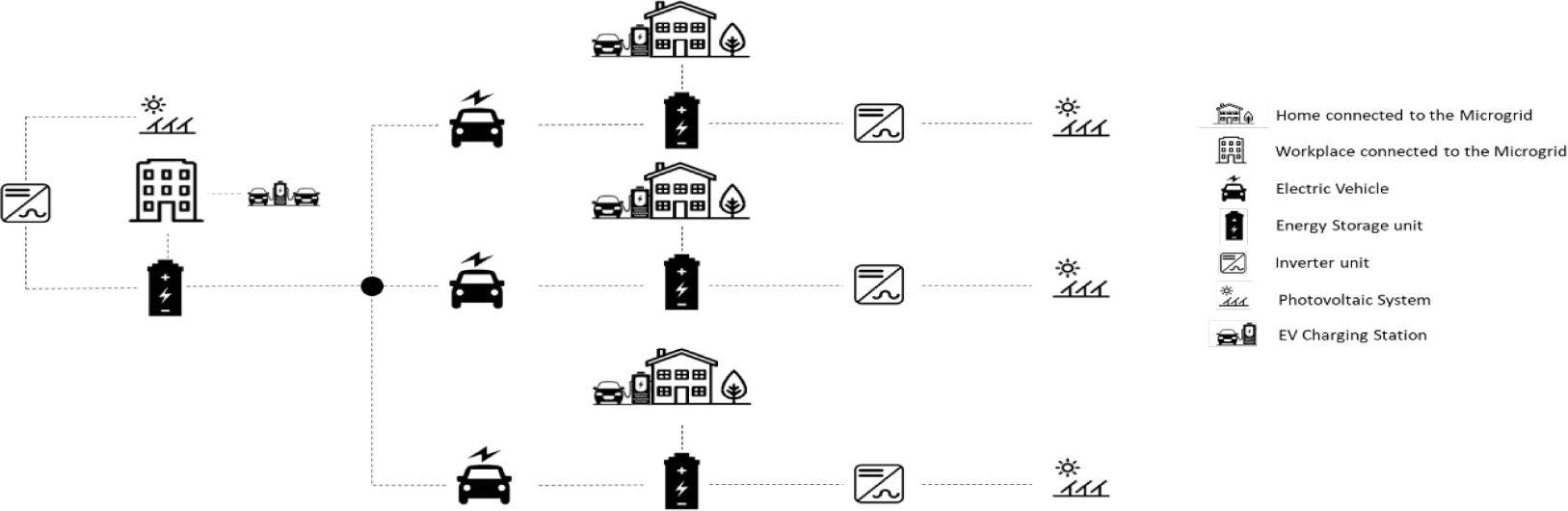
Following the methodological approach presented above, the first stage considers the citizens' opinions regarding the development of the energy management scheme. In 2020, the research team organised and conducted a questionnaire-based survey in the reference area and analysed the responses. The survey targeted 104 citizens of the City of Patras. An overview of the demographic characteristics of the respondents is given in Table 3. Figure 5 presents the most important reasons for users to buy an EV. Based on the responses, environmental concerns, energy price, cost, and the utilization of EV batteries lie among the most important motives. More specifically, there is an alignment between the survey responses and the architecture and operation of the proposed system, as the above-mentioned most popular choices have been considered for the design and development of the decision-making process.
Electric Mobility selection parameters specified by future users
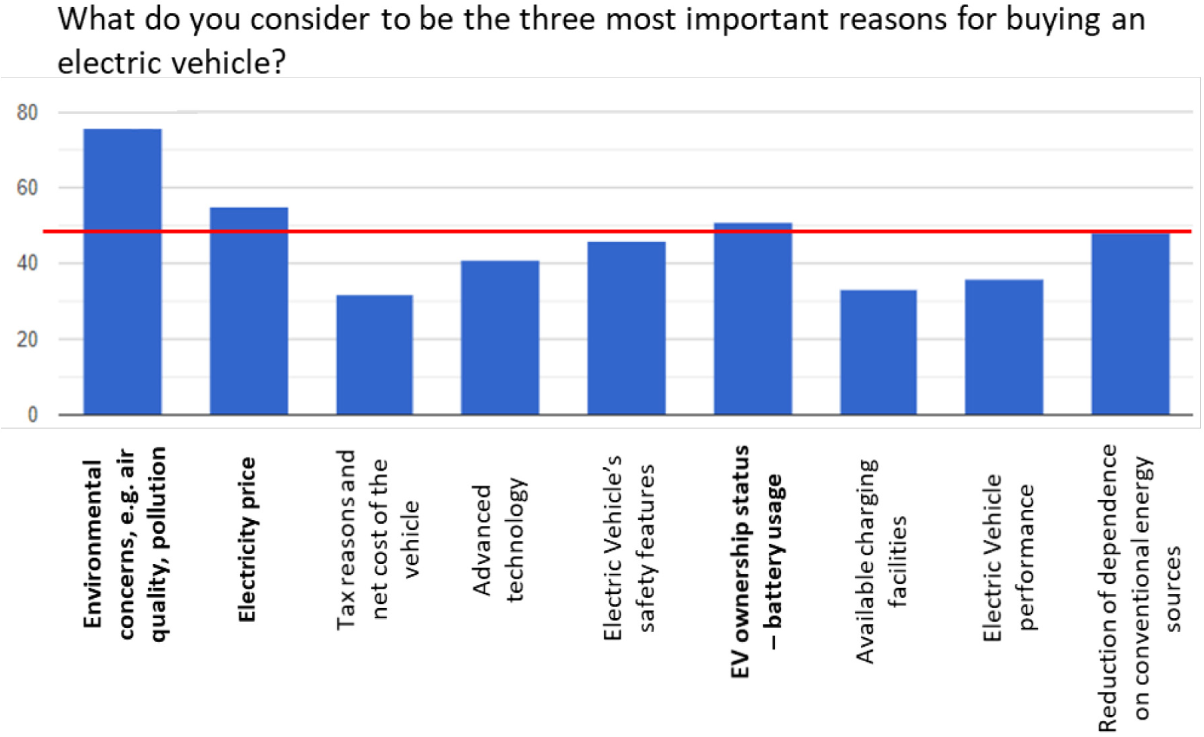
Demographics of survey respondents
Demographic parameter |
Number |
Percentage [%] |
|
---|---|---|---|
Gender |
Male |
51 |
49 |
Female |
53 |
51 |
|
Age |
18–25 years |
28 |
26.9 |
26–35 years |
41 |
39.4 |
|
36–45 years |
19 |
18.3 |
|
46–55 years |
14 |
13.5 |
|
56 years and above |
2 |
1.9 |
|
Education |
Compulsory |
1 |
1 |
Secondary |
18 |
17.3 |
|
Univ./Tech. degree |
41 |
39.4 |
|
University diploma |
30 |
28.8 |
|
Postgraduate diploma |
14 |
13.5 |
|
PhD |
1 |
1 |
|
Relationship status |
Unmarried |
75 |
72.1 |
Married |
30 |
27.9 |
|
Permanent residence in Patras |
Yes |
103 |
98.5 |
No |
2 |
1.5 |
|
Driving license |
Yes |
88 |
84.8 |
No |
17 |
15.2 |
|
Car ownership |
Yes |
68 |
65 |
No |
37 |
35 |
|
Vehicles at the disposal of the family |
none |
21 |
20.2 |
1 |
17 |
16.3 |
|
2 |
51 |
49 |
|
3 and above |
15 |
14.4 |
|
Preferred means of transport |
Motorcycle |
8 |
7.7 |
Car |
53 |
51 |
|
Suburban railway |
10 |
9.6 |
|
City bus |
33 |
31.7 |
|
Taxi |
0 |
0 |
The user (EV owner) profile should be created within the system. This profile includes the average route distance (home to work commute) and other parameters, such as expected daily travelling time and EV systems' energy consumption needs (e.g., AC and media player). Based on this dataset, the system will estimate the energy required for each EV. One can then calculate the energy amount available to be supplied to the microgrid over any reference period by using the following equation:
(8)
where is the total energy required for a trip by an EV [kWh], DS is the round-trip distance [km], and is the energy required for a trip accounting for the desired comfort level at specific meteorological conditions [kWh]. A safety factor ni = 1.3 is introduced to ensure that in all cases there will be sufficient energy available for the users to complete their everyday commute, regardless of unplanned needs that might require additional energy.
An important goal within the energy management system is to ensure that the EV owner's comfort level is not disrupted even in cases that the microgrid requires to absorb the maximum of the available energy provided by the EV. The available EV energy for exploitation by the microgrid is:
(9)
where is the available battery capacity at the start of the scheduling [kWh]. Nowadays, EVs have higher battery capacity than previous generations and faster or even supercharging capability. The increasing EV mass production and competition among the world's biggest car manufacturers have helped decrease purchase costs to levels close to conventional vehicles. These are some important factors that have led to increasing EV penetration in the market, with a further increase anticipated in the future. Based on current trends in the case study, a 40% EV penetration rate has been set.
The microgrid consists of the following components: the 10-office building, the photovoltaic plant, the energy storage system (ESS), the electric vehicle charging station, and the electric vehicles connected to the charging station. The microgrid is designed with four charging sites (one at each building), considering the suggested penetration rate. Apart from the number of available EVs connected to the system at any time, there are some constraints regarding the EV batteries. The battery is among the most expensive EV components that determine EV usability and viability as a product. The battery life cycle and performance are the main parameters considered in the proposed method.
EV batteries nowadays provide a capacity higher than what daily usage would demand. A battery of a 75 kWh capacity is a standard for vehicle manufacturers, while statistically, the average daily demand is not more than 10 kWh. It is possible to use the available excess energy for alleviating building energy demand peaks during the day.
EV batteries' life span and charging cycles are also important parameters. Tesla batteries present about 5,000 to 6,000 cycles before losing their capacity. Studies argue that the lifespan loss is minimised if the battery is used to 80% of its capacity [22]. Accordingly, eq. (10) is:
(10)
where is EV battery energy that may be available for use by the system. Based on the average EV life charging cycles, a soft constraint is set in the optimization process to allow no more than four cycles of EV charge/discharge daily. The limit is set according to the results of a survey reporting an 89% EV owner sample that would follow the constraint (see Table 4):
(11)
where is the acceptable daily number of EV battery charging and discharging cycles.
The user preferences and willingness to participate in the Energy Management Scheme are also studied by introducing a value to show the degree of desired participation on a scale from 1 to 10 (with 1 indicating the lowest and 10 the highest potential participation). This input, along with the number of available cycles of the EV battery, corresponds to two important indications of availability, which have an impact on the energy that the system can use for the energy management scheme. The energy of EV battery that can be available for use by the system is assumed and shown in Table 4.
Participation willingness effect on EV Energy availability parameters
Availability choice (Scale 1 to 10) |
[%] |
|
---|---|---|
1 |
1 |
20 |
2 |
1 |
30 |
3 |
1 |
40 |
4 |
2 |
50 |
5 |
2 |
60 |
6 |
2 |
70 |
7 |
3 |
70 |
8 |
3 |
80 |
9 |
3 |
100 |
10 |
4 |
100 |
The last constraint regarding the EV battery is the level of charging and discharging provided by the charging station. There are three levels of charging, starting from level 1, provided by the national grid simple outlet, to level 3, provided by superchargers. In this work, the intermediate level 2 chargers are considered, which are 5.6% more efficient than level 1 and way less expensive than superchargers. Level 2 chargers typically range from 16 A to 40 A and may be referred to as 3.3 kW and 7.2 kW, respectively. Superchargers installed in a specific microgrid solution would not affect the national grid much. However, having more systems (including more EVs and batteries that need to be charged simultaneously) would affect the national grid as they are 480 V and require installing special equipment. The possibility of their common use in homes would create risks of more frequent demand peaks.
The optimization process is designed and executed using the methodology of genetic algorithms and the support of the computer package Evolver of the Palisade company. This software provides the ability to work with Microsoft Excel, where the problem and all the parameters have been formed to evaluate the algorithm's effectiveness. The software enables changing the three basic parameters of the genetic algorithm methodology, namely the initial population size, the crossover rate, and the mutation rate.
The purpose of the presented application is to efficiently manage the microgrid energy resources and plan the charging and discharging cycles of electric vehicles. Based on the results, the scheme appears to have a positive performance by using the proposed Energy Management Scheme − including photovoltaic system installations and utilising the energy available from electric vehicle batteries.
For the purposes of this application, a total of 3 EV users have been specified, with the same car and specifications, although with different travel requirements and energy availability. The EV's preferences are presented in Table 5.
Electric Vehicles' energy-related parameters
EV1 |
EV2 |
EV3 |
|
---|---|---|---|
Initial battery state of charge |
100% |
50% |
70% |
Travel requirement |
5.19 kWh |
2.29 kWh |
19.11 kWh |
Usable battery capacity |
60 kWh |
60 kWh |
60 kWh |
Energy available for the system |
54.41 kWh |
27 kWh |
22.89 kWh |
Participation level (scale 1 to 10) |
7 |
10 |
10 |
Number of battery cycles available |
3 |
4 |
4 |
Figure 6 presents the proposed schedule for charging and discharging Electric Vehicles. All constraints set above have been taken into account. The system has responded well to the expectations, assisting the microgrid in achieving its environmental and economic goals. Of decisive importance is scheduling the charge of the EV when the energy price is low and discharge when it is high, as provided by the ToU model (Figure 3). Another indication of efficient functionality is the concentration of scheduled discharges between 12:00 to 14:00 when peak demand and a high energy cost may occur.
EV charging and discharging schedule

Figure 7 shows an overview of energy consumption, the use of the EV batteries and PV installation, and the savings resulting from the proposed Energy Management scheme. More specifically, the demand during the reference day is visible, where the initial state (original state) is compared with the one proposed by the energy management scheme. In the demand profile proposed when EMS is enabled, 17 demand groups reduce to 15.
Comparison of initial and final energy demand curves of the Microgrid of Reference

There is a distinct reduction in demand during the peak hours indicated by the ToU, which is also combined with the maximisation of energy storage utilization, towards reducing peak demand on the grid. The scheme follows the users' preferences regarding the energy availability and the number of charging/discharging cycles of the EV batteries scheduled for the day of reference. These observations indicate the scheme's proper operation and the adaptation capabilities to the energy market needs, based on the case-by-case ToU policy.
There are some metrics based on the adopted criteria set that supplementary confirm this conclusion. The energy grid cost for the initial state was 55.19 € (for the day the scheme was proposed) and has been reduced to 42.63 €, presenting economic efficiency equal to 22.75%. From this reduction, a part is attributed to the utilization of renewable sources, which provided a 9.22% cost reduction equal to 5.09 €. It becomes evident that the use of storage elements, such as the batteries installed in the office building and houses, along with the EV batteries, are responsible for the major part of the cost savings achieved by using the proposed Energy Management Scheme.
The modern lifestyle combined with the increased energy requirements for the service of human activities is among the main factors that negatively affect the environment and are associated with phenomena such as the increase in average temperature and the greenhouse effect. In addition, providing for the installation of the required infrastructure to ensure a smooth transition to electrification, as well as encouraging the use of electric vehicles by consumers, can have some positive effects on the environment and everyday life in the cities.
It has been shown that the development of charging infrastructure combined with the implementation of appropriate strategies to reverse the behaviour of citizens (users of electric vehicles) in the workplace can reduce the burden on the power system during peak hours. A part of the daily energy demand of electric vehicles is the battery energy consumed to drive from home to work. This part can be recovered in the morning at work, when system demand is still relatively low, or can be treated by charging again at night, with energy produced by photovoltaic systems and stored in batteries.
This paper used a hybrid approach combining AHP methodology for parameter weight definition and genetic algorithm optimization techniques to integrate RES and electric vehicles in a smart microgrid. The proposed model, combined with a dynamic pricing policy (Time of Use), provides the necessary incentives to attract the user's interest and delivers better results against uncontrolled charging.
The proposed solution was examined by simulation using case scenarios in a specified area in Greece. Upon problem parameterization, a model was formed that serves energy management and optimization in its use. The proposed scheme is configured so that small modifications could be implemented in real pilot applications in the future.
B |
economic benefit |
[€] |
Bat |
battery parameter (capacity, available energy, number of charging/discharging cycles) |
[kWh], [-] |
C |
energy cost, energy price |
[€], [€/kWh] |
CI |
consistency index |
[-] |
CR |
consistency rate |
[-] |
E |
energy amount |
[kWh] |
EPV |
energy production from photovoltaics |
[kWh] |
RI |
random consistency index |
[-] |
Greek letter |
||
λ |
eigenvalue in AHP calculation |
[-] |
Subscripts and superscripts |
||
av |
available |
|
Bat |
battery |
|
cap |
capacity |
|
cycles |
charging/discharging cycles |
|
fact |
factor |
|
i |
i-th time period |
|
max |
maximum |
|
min |
minimum |
|
Res |
renewable energy sources |
|
sys |
system |
|
t(i) |
at the i-th time period |
|
Abbreviations |
||
AHP |
Analytic Hierarchy Process |
|
EMS |
Energy Management System |
|
ESS |
Energy Storage System |
|
EU |
European Union |
|
EV |
Electric Vehicles |
|
PV |
Photovoltaic |
|
RES |
Renewable Energy Sources |
|
ToU |
Time of Use |
|
UK |
United Kingdom |
|
V2B |
Vehicle to Building |
|
V2G |
Vehicle to Grid |
The work presented in this paper received funding by the Horizon 2020 Programme, TwinERGY Project, Grant Agreement Nr. 957736
Decarbonizing transport in the European Union: Emission performance standards and the perspectives for a European green deal ,Sustainability (Switzerland) , Vol. 12 (20), 2020, https://doi.org/https://doi.org/10.3390/su12208381
, - European Commission, 2015, https://ec.europa.eu/energy/sites/ener/files/documents/1_EN_autre_document_travail_service_part1_v6.pdf, [Accessed: Aug. 07, 2020]
The role of supreme audit institutions in energy accountability in EU countries ,Energy Policy , Vol. 156 , 2021, https://doi.org/https://doi.org/10.1016/j.enpol.2021.112413
, Upscaling smart local energy systems: A review of technical barriers ,Renewable and Sustainable Energy Reviews , Vol. 131 , 2020, https://doi.org/https://doi.org/10.1016/j.rser.2020.110020
, Incentives and concerns on vehicle-to-grid technology expressed by Australian employees and employers ,Transportation Research Part D: Transport and Environment , Vol. 98 , 2021, https://doi.org/https://doi.org/10.1016/j.trd.2021.102986
, Stochastic Coordination of Plug-In Electric Vehicles and Wind Turbines in Microgrid: A Model Predictive Control Approach ,IEEE Transactions on Smart Grid , Vol. 7 (3),pp 1537-1551 , 2016, https://doi.org/https://doi.org/10.1109/TSG.2015.2475316
, - , The demand response support under weather impacts using PV generation and EV energy storage, 2016
Energy Management Systems for Residential Buildings with Electric Vehicles and Distributed Energy Resources ,IEEE Access , Vol. 9 , 2021, https://doi.org/https://doi.org/10.1109/ACCESS.2021.3067950
, Review of positive and negative impacts of electric vehicles charging on electric power systems ,Energies , Vol. 13 (18), 2020, https://doi.org/https://doi.org/10.3390/en13184675
, Integration of renewable energy into the transport and electricity sectors through V2G ,Energy Policy , Vol. 36 (9),pp 3578-3587 , 2008, https://doi.org/https://doi.org/10.1016/j.enpol.2008.06.007
, - European Parliament briefing: Understanding electricity markets in the EU, 2016, http://www.easyres-project.eu/wp-content/uploads/2019/02/understanding-elecricity-markets-in-the-eu.pdf, [Accessed: Aug. 07, 2020]
- Cooperation between Horizon 2020 Projects in the field of Smart Grids and Energy Storage Main findings and recommendations Data Management Working Group, https://www.h2020-bridge.eu/wp-content/uploads/2018/06/BRIDGE-Data-Management-WG-Findings-and-Reco-July-2019.pdf, Jul. 2019[Accessed: Aug. 07, 2020]
- Strategies of energy democracy, 2016, https://www.rosalux.de/fileadmin/rls_uploads/pdfs/sonst_publikationen/strategies_of_energy_democracy_Angel_engl.pdf, [Accessed Dec. 25, 2020]
Vehicle-to-Grid in the UK fleet market: An analysis of upscaling potential in a changing environment ,Journal of Cleaner Production , Vol. 290 , 2021, https://doi.org/https://doi.org/10.1016/j.jclepro.2020.125203
, - , Day-ahead battery scheduling in microgrid considering wind power uncertainty using ordinal optimization, 2014
- , The optimal economic dispatch of smart Microgrid including Distributed Generation, ISNE 2013 - IEEE International Symposium on Next-Generation Electronics 2013, 2013
Two-Stage Economic Operation of Microgrid-Like Electric Vehicle Parking Deck ,IEEE Transactions on Smart Grid , Vol. 7 (3),pp 1703-1712 , 2016, https://doi.org/https://doi.org/10.1109/TSG.2015.2424912
, A Model Predictive Control Approach for Low-Complexity Electric Vehicle Charging Scheduling: Optimality and Scalability ,IEEE Transactions on Power Systems , Vol. 32 (2),pp 1050-1063 , 2017, https://doi.org/https://doi.org/10.1109/TPWRS.2016.2585202
, - , Microgrid Integration, Special Topics in Renewable Energy Systems, 2018
Towards improved understanding of the applicability of uncertainty forecasts in the electric power industry ,Energies , Vol. 10 (9), 2017, https://doi.org/https://doi.org/10.3390/en10091402
, - Understanding the True Value of V2G, 2019, https://www.cenex.co.uk/app/uploads/2019/10/True-Value-of-V2G-Report.pdf, [Accessed: Sep. 09, 2020]
- , Dynamic EV battery health recommendations, 2018