The global number of vehicles is expected to increase to 2,9 billion in 2050, with the transportation sector being responsible for approximately two-thirds of emissions increase from all sources in 2030 [1]. One of the main sources of greenhouse gas emissions and other air pollutants is the transportation sector and in particular road transport [2]. Litman [3] in his research states that transport is associated with issues not only of environmental sustainability but also of the economy and society.To this end, ensuring the sustainability of this sector has become a crucial issue in today's world, as indicated by an increasing number of global initiatives [4]. For example, reducing emissions from transport is crucial to achieving the EU's climate neutrality targets [5] which has been supported by a multitude of policies in recent years such as the European Green Deal [6] andthe European Strategy for low-emission mobility [7]. The development and adoption of innovative technologies with an improved environmental performance than conventional ones are important to reach sustainability in transportation [8]. This is particularly the case in the transportation domain [9].
Electric vehicles (EVs) have attracted increasing interest in recent years from both the industry and many countries as an environmentally friendly and energy-efficient way of transport. [10]. It is now imperative that the increase in EV usage is being supported at the global level by using best management practices and by integrating these vehicles smoothly into contemporary reality. This transition is being accelerated by a number of targeted regulations around the world such as the Emission Performance regulation in Europe [5], the Zero-Emission Vehicle mandate in California [11] and the New Energy Vehicles mandate in China [12] As governments attempt to increase the adoption of electric vehicles, manufacturer and driver integration of mobile apps will become an increasingly important source of information, control, and motivation taking advantage of the fact that EV drivers are particularly well suited with mobile apps due to the high adoption rate of smartphones [13]. In this way, the integration of advanced digital technologies is achieved, supporting users with additional services, and increasing their level of satisfaction [14]. Furthermore, both in the academic and business spheres, innovative services are a predominant area of interest [15]. In order to assist EV drivers and to increase the diffusion of EVs, several applications have been developed in previous works, including applications that provide: (a) information to drivers on their way to the nearest charging station (CS) and assistance on route planning [16], (b) information to drivers regarding the status of charging stations [17], (c) optimum route based on data collected from the EV users [18], (d) cybersecurity defence mechanisms [19], (e) prediction of mileage capacity [20] and payment mechanisms [21]. Many works have considered different problems related to EV charging scheduling to minimize waiting time as well as traveling time for the vehicles [22] or considering the actions of other EVs [23].
Charging stations are likened to multi-server queues in [24], a theoretical lower bound to the charging time is derived, but it does not take into account deviations of EVs from their initially scheduled route in order to reach a preferable charging station. A multi-objective decision-making model based on personalized preferences is presented in [25], with the aim to optimize both travel time and charging cost, but without considering the effect of such individual decisions on each other. In [18], the authors develop a model to support EV drivers find the nearest charging station through an interactive application developed via SQL and PHP platforms to assign EVs their charging locations. The authors in [26] propose a charging assistance application based on the history of demand for energy, the location of EVs, and the time of connection. A booking application based on specific preferences provided by the EV driver to identify the optimal route to the CS is implemented in [27]. A framework that provides a real time connection between EVs and CSs is proposed in [28]. In this work, information regarding the peak/off-peak pricing of chargers and average charging times are provided by CSs, while EV users can select the CS with the aid of a weighting cost of charging and the distance to the charging station. Other works focus on minimizing the waiting and queuing time for EV charging, which is one of the most important features in the context of smart cities [22]. Similarly, the authors in [29] propose a time optimizing navigation system to provide users with charging options where the time parameter includes the driving time, waiting time, and charging time.
From the network side, electric vehicles play an important role, as they require large amounts of energy and, in case of irrational or not optimum management of the charging process, can cause large fluctuations in energy consumption. This can cause network overloading and the necessity to increase non-renewable energy production rates to meet the demand with a negative impact on the environment. The extent to which electric vehicles are charged by the electric grid depends on the number of vehicles connected at each point of the electric grid, as well as on the available power during the charging period.
This paper develops an algorithmic deterministic sequence of actions (processes), which, through multicriteria analysis, identifies the best charging station selection for each electric vehicle within a smart power grid. The algorithm is based on both the data entered by the respective EV owner and those of all charging points within the network. It examines all possible (and feasible) alternatives and suggests the best one among them. The examined alternatives are not confined to the comparison among stations at a specific time but consider different possible starting points of charge. The multi-criteria analysis of different time frames in combination with the dynamic pricing data could lead users to new alternatives to reduce the overall cost of charging, especially outside peak hours. The main parameters that are taken into account are the cost of energy supply, the distance and the expected time of the electric vehicle to reach the potential charging station at the time of request, the vehicle specifications (battery capacity, current level and maximum energy level required), the status of the charging stations and specific user preferences. Impracticable or inefficient solutions are discarded, for example when the user criteria are not met, or the EV energy reserve is not sufficient to approach the CS.
The main contribution of this research is a methodological approach for an EV user recommendation system that uses an algorithmic multi-criteria analysis to evaluate a variety of different parameters that influence the user's choice of charging station. This algorithm not only increases user satisfaction and reduces costs, but also improves urban mobility and reduces emissions during charging routes. The results can be exploited by both the academic and entrepreneurial community, enhancing the growth of electromobility Αs presented in the framework introduced by [30], digital innovations are an important pillar of digital transformation [31]. Thus, such digital innovations can accelerate digital transformation in the transport sector, improving the sustainability of businesses that support electromobility.
The paper is structured as follows. The first section refers to the materials and methods used for the optimization process in order to find the best solution for each individual EV user. The next section introduces in detail the methodology used as well as the parts of the algorithm written in pseudocode. A simulated case study is developed in the next section, concerning the interaction between users and charging stations and the evaluation of the pilot results is identified. Finally, the results and conclusions are presented and some future extensions which may be applied are mentioned.
The multi-criteria analysis is carried out in accordance with the internationally accepted PROMETHEE II and Analytic Hierarchy Process (AHP) methodologies. AHP method [32] provides a proven and effective way to handle complex decision problems and can help analyze the data collected and determine the importance of the criteria, while it is used to extract scales of proportion for both discrete and continuous coupled comparisons. These comparisons can be obtained from real measurements or from a fundamental scale which reflects the relative strength of preferences. It has been extensively used in a number of applications, such as decision making, resource planning and allocation, and conflict resolution. In its general form, AHP is a nonlinear framework which considers several factors all together. PROMETHEE II (Preference Ranking Organization METHod for Enrichment Evaluations) is a popular method in the area of multicriteria evaluation or multiple criteria decision aid (MCDA) [33]. It provides a decision support system that deals with evaluating and classifying a range of options, based on various criteria, for finding the best decisions. The combination of the above methods [34] is used in this work to provide a comprehensive framework for classifying EVs charging options.
The key elements of the optimization process are the algorithm, the numerical simulator, and the representation of the physical processes. The best solutions for each individual user in terms of charging station selection results from a defined and executable sequence of actions (processes) which analyze a variety of criteria and factors as well as preferences introduced by the driver of the electric vehicle. The algorithmic process is fed with geographical, cost, and user preference data. Then, it assesses the offered charging alternatives and provides a sorted range of optimum solutions to the user.
The primary piece of information by each charging station refers to the plug types that it supports in order to assure the suitability of the station to the user. A station must provide at least one plug fitting to the user's options. Another input from the station is the available ways in which a customer can pay the charging transaction (by Cash, POS, Blockchain Crypt). The type of charging station (public, commercial, or residential station) is further provided as an available information to the users as well as the amenities that can be available to users nearby (restaurants, shopping malls, supermarkets). Another important factor in selecting a charging station is its availability at the preferred time of charging. The time discretization for vehicle charging is set to one quarter of an hour in order to avoid long-time gaps during which the station is unused. Shorter time periods might be considered but at an increased computational burden.
The algorithm takes into account that the energy is charged under dynamic pricing conditions, which means that the charging price is not fixed within the day, primarily depending on demand fluctuations and network availability (to cope with the algorithm discretization period, it is considered that the energy price may be altered per quarter of an hour). For example, at times when a station runs at high energy demand, the price could increase. Instead, at times with low demand, the price can drop providing an incentive for prospective EV drivers to charge their vehicles at these periods and also protecting the grid from peaks. The way in which a station can determine the energy pricing policy in a dynamic manner is another major field of study. Following the algorithm discretization period, the energy price may change every quarter of an hour while the energy availability is also calculated at the same timeframe.
The second data subset required for the algorithm realization refers to the EV user preferences and constraints. The driver selects among several options for the plugs that are compatible with his or her vehicle, sets the required energy level to be bought, and chooses the type of station, the payment method, the amenities of interest as well as the preferable time to charge the EV. In addition, the algorithm is fed via GPS with the distance between the vehicle and the station (in km) and the estimated optimum travel time (in minutes). The model could eventually integrate intelligent algorithms for a more accurate prediction of range estimation. For example, drivers profile (i.e. aggressiveness level) as well as environmental and seasonal dynamic parameters could feed the model by applying machine learning algorithms to estimate the distance that can be travelled with the remaining amount of energy [35].
Following charging station and user data setting up, specific criteria are checked for each alternative station. These are the basic criteria which if not all met, the specific selection becomes unacceptable. The basic criteria are the plug type, the availability and sufficiency of the required energy, the station type, the available amenities, the payment method and the range estimation of the EV in correlation with the distance of stations. After the key tests are completed, a table of all possible alternatives is developed.
The available alternatives for EV charging developed in the previous step are then classified and prioritized through a multicriteria analysis. The decision parameters that are considered in this process correspond to the main concerns of a typical EV energy consumer, in particular:
Energy PRICE (cost);
Trip duration;
Charging station distance;
Waiting time.
The relative importance of these factors is represented be corresponding weights that are derived via the Analytic Hierarchy Process (AHP) methodology (Figure 1). The weights are then used in PROMETHEE II to classify alternatives charging solutions. In this way, not only one optimal solution is proposed, but a hierarchical list of all possible alternatives. The user is provided with the best five (5) of them, to choose the one that best suits the needs.
AHP schematic representation
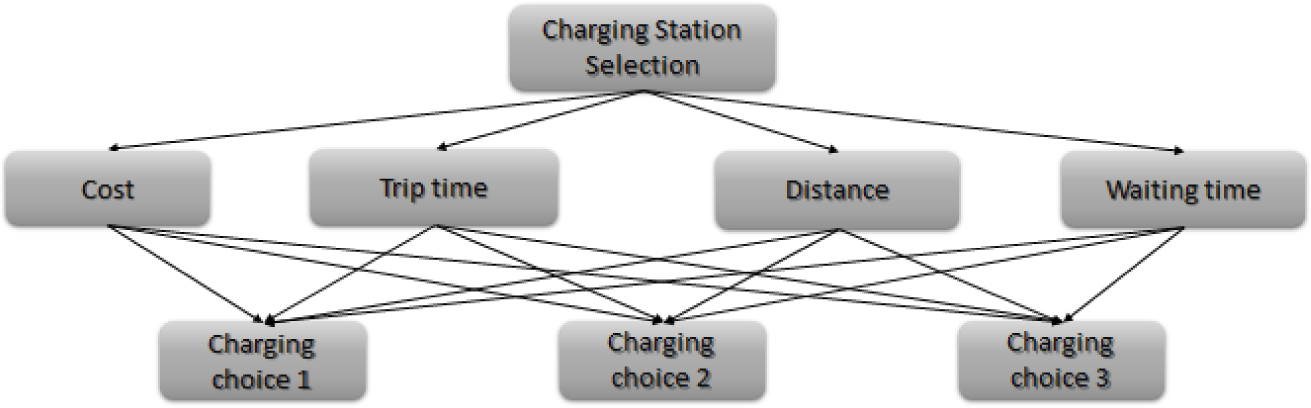
The application of the method starts with the problem breaking down into a hierarchy of criteria so that they are easier to analyze and compare independently. After building this logical hierarchy, alternatives can be systematically evaluated by making comparisons for each of the selected criteria. This comparison may use specific data from alternative solutions or human judgment as a way of entering information [36]. The comparison between two components using the AHP method can be done in different ways. However, comparing the relative importance of two alternatives, as suggested by Saaty [37], is the most popular one. Considering values ranging from 1 to 9, the scale determines the relative importance of one alternative compared to another, as it can be seen in Table 1. It is common to use exclusively odd numbers when referring to relative importance in order to have a reasonable modal split between the measurement points. Even numbers should only be used if there is a strong issue of trading between ratings.
AHP criteria assessment levels
Scale |
Assessment |
Reversed value |
---|---|---|
Significantly outperforming |
9 |
1/9 |
8 |
1/8 |
|
Far outperforming |
7 |
1/7 |
6 |
1/6 |
|
Outperforming to a great extent |
5 |
1/5 |
4 |
1/4 |
|
Slightly outperforming |
3 |
1/3 |
2 |
1/2 |
|
Equal |
1 |
1 |
The criteria comparison for any specific problem is then constructed by pair-wise comparisons of the criteria involved and the values above for setting up the relative importance between criteria pairs (an example for a three-criteria case is presented in Table 2). The value in row i and column j(aij) represents the importance of Criterion i in comparison to Criterion j. A normalization process takes place in which first the sum of each column values is calculated, then the values within the elements are normalized (divided) by the column sum and, finally, the average value in each row is calculated to provide the respective criterion weight Wi (Table 3). The Wi reflects the importance of the i criterion in a scale of 0 to 1.
AHP criteria comparison
Criterion 1 |
Criterion 2 |
Criterion 3 |
|
---|---|---|---|
Criterion 1 |
1 |
Assessment 1-2 |
Assessment 1-3 |
Criterion 2 |
1/Assessment 1-2 |
1 |
1/Assessment 2-3 |
Criterion 3 |
1/Assessment 1-3 |
1/Assessment 2-3 |
1 |
Normalization of criteria comparison and criteria weight assessment
Criterion 1 |
Criterion 2 |
Criterion 3 |
Weights W |
|
---|---|---|---|---|
Criterion 1 |
1/sum(i,1) |
a12 |
a13 |
W1 |
Criterion 2 |
a21 |
1/sum(i,2) |
a23 |
W2 |
Criterion 3 |
a31 |
a32 |
1/sum(i,3) |
W3 |
The next step of the process is to check for the consistency of the calculated weights. First, the value of the maximum eigenvalue, the cohesion factor CI, and the Random Index (RI) are calculated. The equations for calculating these values are shown below:
(1)
The Consistency Index (CI) is defined as:
(2)
Finally, the CI is compared to the Random Index (RI) for the appropriate value n (Table 4). If CI/RI < 0.10, the degree of consistency is considered as satisfactory.
Random Index (RI) indicator for different n values
n |
2 |
3 |
4 |
5 |
6 |
7 |
8 |
9 |
10 |
---|---|---|---|---|---|---|---|---|---|
RI |
0 |
0,58 |
0,9 |
1,12 |
1,24 |
1,32 |
1,41 |
1,45 |
1,49 |
The methodology followed by the multicriteria assessment method of PROMETHEE II [38]–[40] includes:
The definition of criteria j=1, ..., n and of the set of possible alternative solutions for the decision problem;
The determination of the weights (Wj) of the criteria, which indicate the relative importance of each of the criteria, where:
(3)
PROMETHEE II does not provide formal guidelines on how weights can be calculated. In this research work, weight calculation results from the AHP methodology;
Normalization of the decision matrix to range 0-1 using the following equation:
(4)
where Xij is evaluation values provided by decision makers i = 1,…, n, and numbers of criteria j = 1,..,m;
Definition of the preference function:
(5)
dj (a, b) denotes the difference between the evaluations of a and b on each criterion;
Determination of the deviation by pairwise comparison, i.e., the difference between a and b ratings for each criterion:
(6)
the evaluations of alternative a regarding alternative b on each criterion into a degree ranging from 0 to 1. The smaller number of the functions denotes the indifference of the decision maker. On the contrary, the closer to 1 indicates greater the preference.
Computation of the multi criteria preference index:
(7)
where Wj is the weight associated with criterion j. The symbol π (a, b) shows that the degree of a is preferred to b over all the criteria.
Determining the order of preference by ranking the values based on PROMETHE II method:
(8)
(9)
Φ+a represents positive outranking flow (showing how a dominates all the other alternatives) while Φ-a represents the negative outranking flow (showing how a is dominated by all the other alternatives). The alternative with the highest value φ+(α) and the lowest value of φ-(α) is the best. All alternatives can be compared in terms of the φ(α) values, with the highest φ(α) to indicate the most preferred alternative. In the current application, the list of the top five most preferred alternatives is generated and displayed to the user who can (optionally) make his or her own selection.
(10)
The application programming was implemented within Excel using the VB-IDE (Visual Basic - integrated development environment) [41]. However, the below presented methodology can be implemented in SQL server environment integrating Python programming language or any other programming language as well. This way current work can realistically be used as part of a real-world solution, such as a mobile app or an online service, avoiding large volume data limitations and eliminating data movements. For this reason, below the methodology used is presented in detail as well as the parts of the algorithm written in pseudocode. The prototype pieces of code written in VBA are attached in the appendix.
Each station has a computational datasheet which can be fed by the relevant data and also provide available plugs (Table 5).
Station datasheet template
Payment |
Operation Type |
Amenities |
||||||||
---|---|---|---|---|---|---|---|---|---|---|
cash |
pos |
crypto |
Public |
Commercial |
Residential |
restaurant |
shopping |
supermarket |
mall |
|
yes |
yes |
yes |
no |
yes |
no |
yes |
yes |
yes |
yes |
|
1 |
1 |
1 |
0 |
1 |
0 |
1 |
1 |
1 |
1 |
|
Plugs |
||||||||||
Supercharger |
CCS/SAE |
CHAdeMO |
J-1772 |
Tesla |
Tesla (Roadster) |
Type 2 |
Type 3 |
Three Phase |
Wall |
Caravans main socket |
yes |
no |
yes |
yes |
yes |
yes |
no |
no |
no |
no |
no |
1 |
0 |
1 |
1 |
1 |
1 |
0 |
0 |
0 |
0 |
0 |
In the station datasheet, each charging point has been allocated a corresponding column in which the time frames that the position is occupied for EV charging are indicated by a 0 value; otherwise, a value of 1 indicates a charging point free for use. For an enhanced visual representation, all zeros are automatically displayed in red while ones are in green (Table 6). The number of the available charging points of the station is calculated in a column (SUM) at each time interval. Each station inputs the energy price of the kilowatt-hour derived from dynamic pricing algorithms as well as the available energy per time frame. For example, with reference to Table 6, in the interval 00:15 to 00:30, two positions (H3 and H4) are free, while H1, H2 and H5 are occupied. The price of the kilowatt-hour is 2,25 € and the rest of available energy is 5,165 kWh.
Charging point station and individual point data
![]() |
A similar to the charging station template is also provided for gathering EV user's information, some of which is required (plug types, required energy) and some other is optional (payment method, desired station type, amenities and time of charge). More specifically, the required energy is automatically calculated based on the information provided by the user regarding the EV battery capacity, the current charging level and the expected one after charging.
The next step is the development of a central spreadsheet called Evaluation which automatically receives all the information from all station and user sheets. Within the global sheet, all computations are made, either through functions within the scheduled cells or through code execution, the latter also being in a macro format. Table 7 provides an indicative part of the evaluation sheet for station 1. Within the sheet, there are n such tables, where n is the total number of charging stations.
Indicative Evaluation Table for Station 1 (for two time slots)
Station 1 |
||||||||
---|---|---|---|---|---|---|---|---|
Time |
Charging Points |
Energy Cost (€) |
Available Energy (kW) |
Availability |
Price (€) |
Waiting time (mim) |
Distance (m) |
Travel Time (min) |
2000 |
10 |
|||||||
0:00 |
4 |
0,90 |
1500 |
1000000 |
2,727E+09 |
1000000 |
2000 |
10 |
0:15 |
4 |
1,10 |
1450 |
1 |
2,448E+09 |
20 |
2000 |
10 |
Max power |
kWh/15min |
Times |
Last quarter |
Payment |
Op. Type |
Amenities |
Plugs |
|
11 |
2,2 |
14 |
20 |
1 |
0 |
1 |
1 |
For each station, the maximum charging rate is calculated, taking into account the rates of all plugs with which the vehicle is compatible. Then the algorithmic process finds the time (number of quarters) required for charging completion. This is calculated by dividing the total amount of energy required by the charging rate, using a factor of 4 to convert time to a quarter of an hour and 0.9 as a charging efficiency correction factor [42], [43] (eq. (11)). Further, the energy transferred to the EV at each quarter is also calculated by eq. (12).
(11)
(12)
The assessment of the basic criteria is completed with the check of user’s preferences (payment method, plug type and amenities). If these are covered by stations, the condition for potential charging is satisfied and a respective parameter is translated into a value of 1 (otherwise 0).
After completing the basic criteria checks, the following ones are conducted:
check station energy availability;
check charging position availability.
To successfully complete these checks, an algorithmic process is used (see actual source code in annex), which calculates for each station and for all selected starting hours if the conditions for free positions and energy availability are satisfied.
Inefficient solutions are discarded by applying a penalization approach. Penalty functions in a form of huge values (billion euros for price and one million (mins) for Waiting Time parameter) are set to penalize infeasible solutions by reducing their fitness values in proportion to their degrees of constraint violation. In case that both conditions are met, then for the specific start time availability attains the value of 1, while in the opposite case, a high value (eg., 1000000) is set as a way of discarding the specific choices. The flow process of the logical concept is shown in Figure 2.
Energy and charging point availability checking process
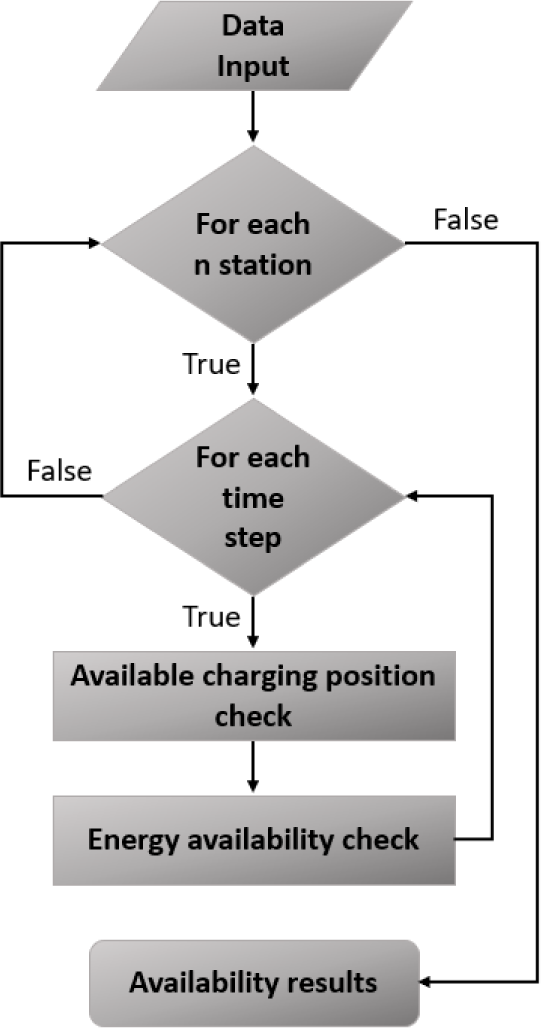
Following the availability assessment of stations and charging points at different time slots, the total price (cost) paid by the user is computed for each possible charging time and station via Algorithm 2 (see actual source code in Appendix), using an iterative computational process written in pseudocode. The calculation of this value is based on the results from Algorithm 1 and these two pieces of code form a single section that runs as a macro command. The process used for the price calculation is shown in Figure 3.
Price calculation process
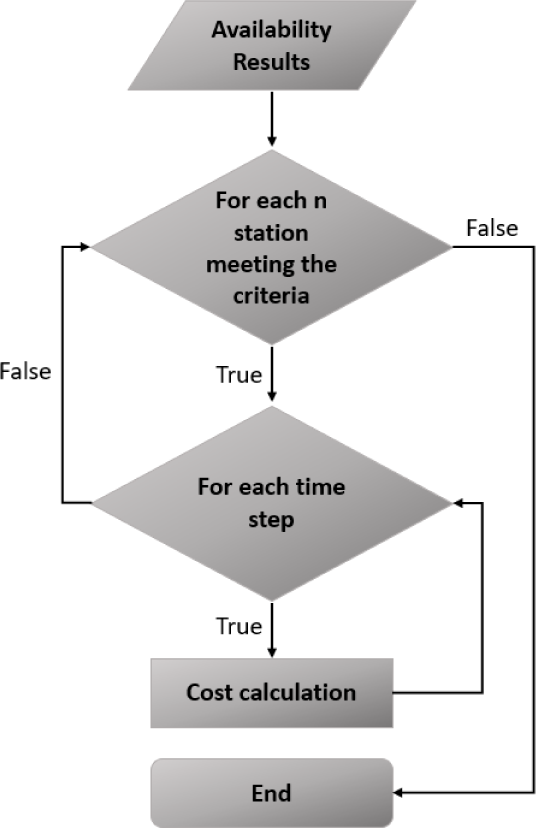
Next, a table is developed and informed for each station, consisting of the four parameters of concern by the user, which forms the base for the multi criteria analysis in order to provide to the user the best charging options (Table 8).
Parameters involved in the multi criteria analysis
|
Price (€) |
Waiting time (min) |
Distance (m) |
Travel time (min) |
---|---|---|---|---|
13:00 |
1,662E+0 |
1000000 |
2000 |
10 |
13:15 |
1,352E+09 |
1000000 |
2000 |
10 |
13:30 |
1,402E+09 |
1000000 |
2000 |
10 |
13:45 |
1,524E+09 |
1000000 |
2000 |
10 |
14:00 |
26,0569 |
0 |
2000 |
10 |
14:15 |
19,1845 |
15 |
2000 |
10 |
14:30 |
20,1438 |
30 |
2000 |
10 |
14:45 |
22,3475 |
45 |
2000 |
10 |
15:00 |
21,978 |
60 |
2000 |
10 |
15:15 |
21,5042 |
75 |
2000 |
10 |
The choices with excessively large values, derived from the penalties that have been applied when the conditions are not met, are removed using the Algorithm 3 (see actual source code in Appendix).
The result is an updated table (Table 9), which contains all feasible choices for the user.
Feasible alternatives
Station |
Time |
Price (€) |
Waiting time (min) |
Distance (m) |
Travel time (min) |
---|---|---|---|---|---|
2 |
14:00 |
25,74 |
0 |
1800 |
8 |
2 |
14:15 |
25,82 |
15 |
1800 |
8 |
2 |
14:30 |
26,86 |
30 |
1800 |
8 |
2 |
18:15 |
24 |
255 |
1800 |
8 |
2 |
18:30 |
23,5 |
270 |
1800 |
8 |
2 |
18:45 |
23,72 |
285 |
1800 |
8 |
2 |
19:00 |
22,56 |
300 |
1800 |
8 |
2 |
19:15 |
22,74 |
315 |
1800 |
8 |
2 |
19:30 |
23,02 |
330 |
1800 |
8 |
2 |
19:45 |
22,12 |
345 |
1800 |
8 |
2 |
20:00 |
22,36 |
360 |
1800 |
8 |
2 |
20:15 |
23 |
375 |
1800 |
8 |
2 |
20:30 |
22,32 |
390 |
1800 |
8 |
3 |
14:00 |
22,34 |
0 |
1700 |
9 |
3 |
14:15 |
21,38 |
15 |
1700 |
9 |
3 |
14:30 |
21,66 |
30 |
1700 |
9 |
The next stage is the implementation of the AHP for defining weights and PROMETHEE II 's methodology for the multicriteria analysis, to result in a sorted list of available options.
The calculation of the weights for all four parameters of the previous table is executed as follows.
First, the values that determine the relative importance of the decision parameters in pairwise comparisons are inserted in Table 10. Further, the sum of the values in each column is calculated. Based on these figures, the table values are normalized (divided) by the corresponding column sum (Table 11). The average of the values in each row provides the weight of the corresponding parameter.
Pairwise comparison values
Distance |
Waiting time |
Price |
Travel time |
|
---|---|---|---|---|
Distance |
1 |
3 |
0,13 |
3 |
Waiting time |
1/3 |
1 |
0,10 |
1 |
Price |
5 |
7 |
0,67 |
7 |
Travel time |
1/3 |
1 |
1 |
|
sum |
6,67 |
12,00 |
0,10 |
12,00 |
Weight calculation II
Distance |
Waiting time |
Price |
Travel time |
Weights |
|
---|---|---|---|---|---|
Distance |
0,15 |
0,25 |
0,13 |
0,25 |
0,1962 |
Waiting time |
0,05 |
0,08 |
0,10 |
0,08 |
0,0782 |
Price |
0,75 |
0,58 |
0,67 |
0,58 |
0,6474 |
Travel time |
0,05 |
0,08 |
0,10 |
0,08 |
0,0782 |
To check these values for convergence, after a series of calculations based on Equations (1) and (2) as well as the data from Table 3, the check results are presented in Table 12.
Weight consistency checks.
λmax |
4,073904 |
|
---|---|---|
CI |
0,024635 |
|
CR |
0,027372 |
<0,1 |
The multicriteria analysis is performed by using the Algorithm 4 (see actual source code in Appendix).
The variables k1, k2, k3, k4 are the weights derived from AHP. In particular, k1 refers to the price parameter, k2 to the waiting time, k3 to the trip time and k3 to the distance. The algorithm takes the normalized parameter values as input and returns the values φ+(x), φ-(x). Then, the alternative solutions are sorted based on the difference between the above values and the five most favourable ones are presented.
For the needs of the pilot application, a simulated environment is developed for the interaction between users and charging stations.
The positioning of 20 stations (commercial, residential, and public) and a fleet of 7 EVs are represented through Google's My Maps, which allows direct calculation of distance between points on the map as well as the estimated time of each route. The application is run in the City of Patras (Greece) and the station distribution within the city is depicted in Figure 4, with station id numbers allocated to them to facilitate computation. The stations are classified to commercial, residential and public ones.
Case study station map with station type indicate
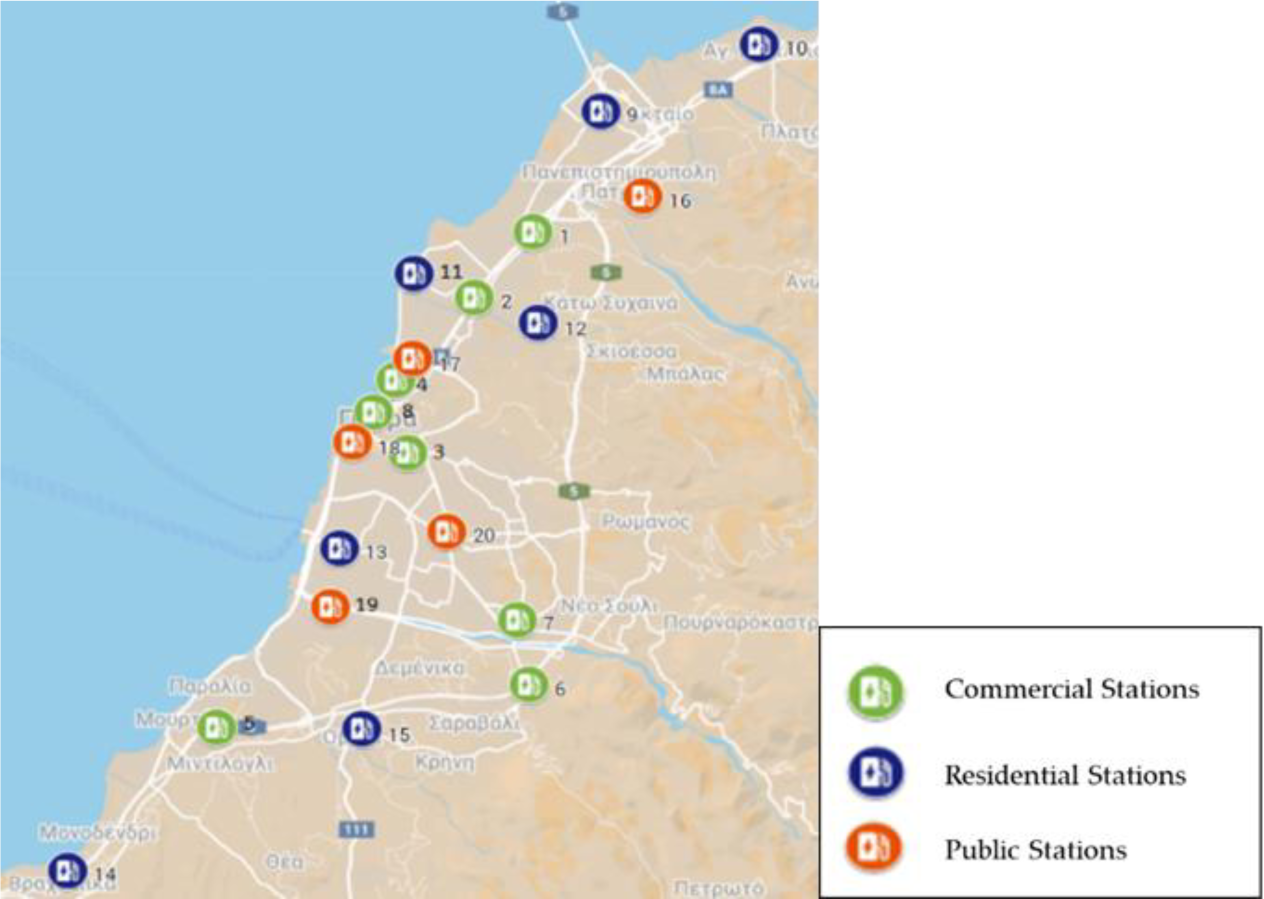
The electric vehicles that need to access a charging point and their drivers are interested to make the best charging station selection are distributed in the city as shown in Figure 5.
Vehicle starting point map
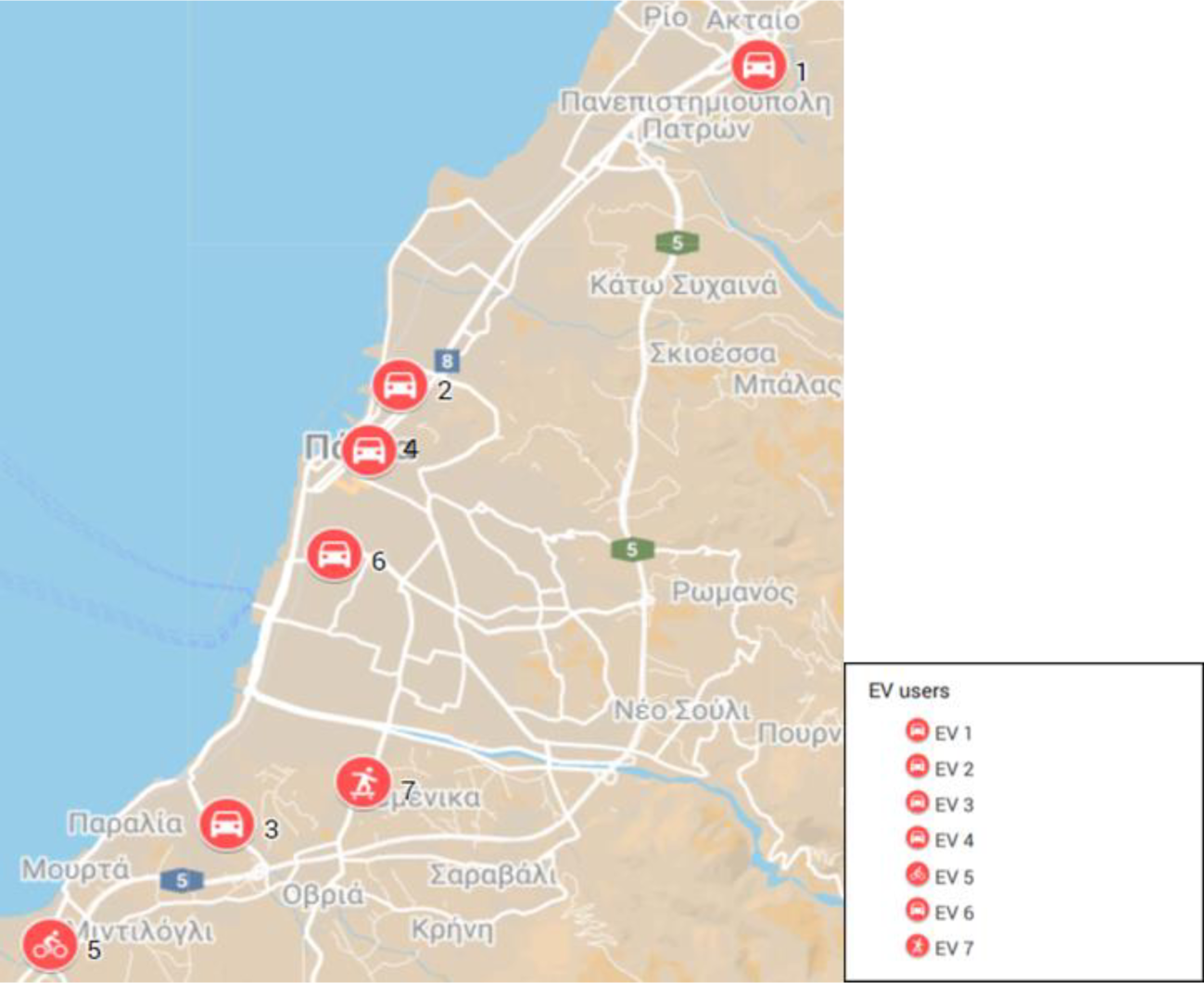
All station and vehicle data were randomly set within specified limits. In particular:
the price of kilowatt-hour [0,4 – 1.2 €/kWh];
the station power availability [0 - 1500 kWh];
the free or reserved charging points with equal probability, 1 and 0 respectively;
the electric vehicle battery size [17,6 to 100 kWh] for a Tesla model;
the charge rate in KW takes the maximum value for each type of plug, as shown in Table 13.
Maximum plug rate (kW)
Plugs |
||||||||||
---|---|---|---|---|---|---|---|---|---|---|
Supercharger |
CCS/SAE |
CHAdeMO |
J-1772 |
Tesla |
Tesla (Roadster) |
Type 2 |
Type 3 |
Three Phase |
Wall |
Caravans main socket |
250 |
150 |
62,5 |
6,6 |
16,5 |
16,8 |
44 |
7,4 |
22 |
2 |
3,7 |
The data for EV input tables are derived from Google map applications (indicatively shown in Table 14) for each specific station.
Data from GPS
EV1 |
Station 1 |
Station 2 |
Station 3 |
Station 4 |
Station 5 |
Station 6 |
Station 7 |
Station 8 |
Station 9 |
Station 10 |
|
---|---|---|---|---|---|---|---|---|---|---|---|
D (m) |
4000 |
5000 |
10000 |
8000 |
20000 |
15000 |
14000 |
10000 |
2000 |
3000 |
|
T (s) |
7 |
9 |
18 |
15 |
20 |
15 |
14 |
18 |
5 |
5 |
It is considered that the driver of a Nissan Leaf S 2017 (EV1) inputs the following data (shown in Table 15) in order to find the optimal charging station. Setting the vehicle’s battery capacity, the current and desirable charge percentage, the required energy is acquired.
Nissan Leaf S data
Battery Size (kWh) |
Charge from (x %) |
To (x %) |
Power Calc |
|||||||
---|---|---|---|---|---|---|---|---|---|---|
30 |
30 |
100 |
21 |
|||||||
Time |
Payment |
Operation Type |
Amenities |
|||||||
14:00 |
cash |
POS |
crypto |
Public |
Professional |
Residential |
restaurant |
shopping |
supermarket |
mall |
yes |
yes |
no |
no |
yes |
yes |
no |
no |
yes |
yes |
no |
1 |
1 |
0 |
0 |
1 |
1 |
0 |
0 |
1 |
1 |
0 |
Plugs |
||||||||||
0 |
0 |
0 |
0 |
0 |
0 |
6,6 |
0 |
0 |
2 |
0 |
Supercharger |
CCS/SAE |
CHAdeMO |
J-1772 |
Tesla |
Tesla (Roadster) |
Type 2 |
Type 3 |
Three Phase |
Wall |
Caravans main socket |
no |
no |
no |
no |
no |
no |
yes |
no |
no |
yes |
no |
0 |
0 |
0 |
0 |
0 |
0 |
1 |
0 |
0 |
1 |
0 |
A second EV2 vehicle (Tesla Model S) needs to charge, and its driver enters the following data (Table 16).
Tesla Model S data
Battery Size (kWh) |
Charge from (x %) |
To (x %) |
Power Calc |
|||||||
---|---|---|---|---|---|---|---|---|---|---|
100 |
0 |
100 |
100 |
|||||||
Time |
Payment |
Operation Type |
Amenities |
|||||||
14:00 |
cash |
POS |
crypto |
Public |
Professional |
Residential |
restaurant |
shopping |
supermarket |
mall |
no |
yes |
no |
no |
no |
yes |
no |
no |
yes |
yes |
no |
0 |
1 |
0 |
0 |
0 |
1 |
0 |
0 |
1 |
1 |
0 |
Plugs |
||||||||||
50 |
0 |
0 |
0 |
0 |
0 |
19,2 |
0 |
0 |
2 |
0 |
Supercharger |
CCS/SAE |
CHAdeMO |
J-1772 |
Tesla |
Tesla (Roadster) |
Type 2 |
Type 3 |
Three Phase |
Wall |
Caravans main socket |
yes |
no |
no |
no |
no |
no |
yes |
no |
no |
yes |
no |
1 |
0 |
0 |
0 |
0 |
0 |
1 |
0 |
0 |
1 |
0 |
The process of evaluating all possible options for Nissan Leaf consists of:
The whole set of options equals to 20 stations × 96 possible start-up times = 1920 solutions;
The set of feasible options, considering station and EV characteristics as well as user preferences and constraints, drops down to 109;
From the related data, it is found that the stations that meet the basic user requirements are those with numbers 1, 2, 3, 4, 20;
By sorting the φ values, the algorithm develops the list of the five best choices for the user (Table 17).
Nissan Leaf S optimal solutions
Station |
Time |
Price |
Waiting time |
Distance |
Travel time |
φ |
---|---|---|---|---|---|---|
1 |
14:00 |
13,338 |
0 |
4000 |
7 |
0,5501 |
1 |
14:15 |
14,214 |
15 |
4000 |
7 |
0,4482 |
3 |
14:00 |
13,644 |
0 |
10000 |
18 |
0,3466 |
1 |
14:30 |
15,204 |
30 |
4000 |
7 |
0,3343 |
2 |
19:00 |
13,164 |
300 |
5000 |
9 |
0,3286 |
It appears that the algorithm sensitivity to the various changes in all parameters is quite satisfactory, thus it can provide the set of optimal choices sufficiently. Eventually, the EV driver makes the first choice out of five and automatically commits that location to station 1. Accordingly, the process of evaluating all possible options for the Tesla EV includes:
The whole set of options equals to 20 stations × 96 possible start-up times =1920;
Ultimately, the set of feasible options is 42;
From the data in Table 16, it is found that the stations that meet the basic user requirements are those with numbers 2 and 3;
By sorting the φ values, the algorithm suggests the list of the five best choices for the user. In this case, apart from the basic selection criteria that are “on” – “off”, the user has set as the only parameter of interest the charging price. As a result, all five best alternatives are associated to station 2 (Table 18).
Optimal choices for the Tesla Model S
Station |
Time |
Price |
Waiting time |
Distance |
Travel time |
φ |
---|---|---|---|---|---|---|
2 |
19:30 |
6,996 |
0 |
3000 |
7 |
-6,9960 |
2 |
18:30 |
7,128 |
0 |
3000 |
7 |
-7,1280 |
2 |
19:15 |
7,128 |
0 |
3000 |
7 |
-7,1280 |
2 |
19:45 |
7,128 |
0 |
3000 |
7 |
-7,1280 |
2 |
19:00 |
7,26 |
0 |
3000 |
7 |
-7,2600 |
From the users’ perspective, the primary concerns in EV charging are the long recharge time, the proximity of the charging stations and the availability of charging spots where EV drivers can recharge their vehicles. The application presented in this paper is able to greatly mitigate the doubts about the CS positioning and availability and reduce driver’s anxiety through reservation services. In addition, the proposed methodology is not limited to comparing alternative charging options at a specific time, but it considers the cost of energy as a time parameter within a dynamic pricing context. This means that multiple possible starting times of charging are processed and optimized. In this way, the proposed algorithm can lead users to charging alternatives outside of peak hours, letting them enjoy reduced generalized total cost of charging and respecting the principles of sustainability.
In addition, the results indicate that it is possible to:
Address the problem of intermediate charging of EVs, without requiring the costly creation of new charging stations but optimizing the management of the existing infrastructure;
Enhance the use of electric vehicles, contributing to the reduction of conventional vehicles and thereby reducing the environmental impact which they cause;
Minimize large energy demand fluctuations in the energy generation and transmission network since the deployment of EVs;
Shift large volumes of EV charging from peak hours to periods when the network is at rest;
Encourage the use of renewable energy sources by supporting participation in a free market, with a view to making the produced energy available to the users;
Benefit financially the EV owners from the formation of an open energy market and the proposed best alternative charging options, based on their preferences.
Finally, some future extensions which may be applied are mentioned. The online application can provide a dynamic pricing algorithm, which, depending on the availability of energy, free positions, and other parameters related to network characteristics, can calculate a proposed energy sale price. Another extension could cover the uncertainties of computing the trip time. For example, for a charge starting five hours after the algorithm running, the EV will probably face different traffic conditions at that time. The proposed model is based on a general multi-criteria analysis methodology and is designed to be easily modifiable, thus it can serve as the basis for any future extension and can be also applied to other problems of similar structure. In particular, it can generate a hierarchical list of best alternatives to any decision problem that contains a number of noncomparable direct parameters (e.g., cost, time, distance, etc.) as, for example, for bicycles and skates charging.
This paper contributes to academic knowledge through a methodological framework of an algorithmic multi-criteria analysis process for the evaluation of different parameters that influence the user's choice of charging station. The innovative approach of the paper takes into account four main different parameters of cost per kilowatt-hour, waiting time, travel time and distance, while considering costs under dynamic pricing conditions. In this way, the user can actually choose the optimal option and avoid hidden costs that may arise from delays at charging stations or due to traffic congestion. At the same time the user can avoid a choice that seems to be cheaper but ends up costing more due to the long distance to the station, causing problems for urban mobility and sustainability by emitting unnecessary emissions and causing congestion on the roads. The methodology also takes into account a number of other key parameters such as the method of payment, the availability of stations, the compatibility of stations and the amenities they provide. The methodology also considers a number of other key parameters such as the method of payment, the availability of stations, the compatibility of stations and the amenities they offer, thus eliminating in good time the wrong choices which are a burden on traffic, the environment and the user. The methodology developed through this research can be exploited in different avenues by both the academic and the entrepreneurial community, which addresses its extensibility towards other challenges and needs of implementation. Also, operators of electric vehicle information applications worldwide can be guided to create a new service by utilizing the results of this work by contributing to increase user satisfaction, enhancing electromobility, urban mobility and sustainability of cities. In other words, the introduction of digital innovations in society such as the one proposed by this paper can ensure the diffusion of electromobility and sustainable transportation by accelerating the digital transformation of companies in the sector and providing incentives to the users of electric vehicles.
Author Contributions: S.K. P.F. and A.C.; methodology, S.K and P.F.; software, S.K, P.F. and T.F; validation, S.K, P.F. and T.F; formal analysis, S.K, P.F. and T.F.; investigation, S.K, P.F. and T.F.; writing—original draft preparation, S.K and T.F.; writing—review and editing, S.K. and A.C.; visualization, S.K and T.F.; supervision, A.C and Z.C.; project administration, A.C.; funding acquisition, A.C, S.K and P.F All authors have read and agreed to the published version of the manuscript.
Funding: This research is co-financed by Greece and the European Union (European Social Fund-ESF) through the Operational Programme «Human Resources Development, Education and Lifelong Learning 2014-2020» in the context of the project “Development of Dynamic Pricing and Autonomous Trading Application for Electric Vehicle Charging in the Digital Energy Market context” (MIS 5047183).

Abbreviations |
|
---|---|
AHP |
Analytic Hierarchy Process |
CI |
Consistency Index |
CS |
Charging Station |
CCS |
Combined Charging System |
CHAdeMO |
DC fast charging protocol |
DC |
Direct Current |
ESF |
European Social Fund |
EV |
Electric Vehicle |
GPS |
Global Positioning System |
MCDA |
Multiple Criteria Decision Aid |
RI |
Random Index |
POS |
Point of sale |
PROMETHEE II |
Preference Ranking Organization METHod for Enrichment Evaluations |
SAE |
Society of Automotive Engineers |
SQL |
Structured Query Language |
VBA |
Visual Basic for Applications |
Climate change, enhanced greenhouse gas emissions and passenger transport – What can we do to make a difference? ,Transp. Res. Part Transp. Environ. , Vol. 13 ,pp 95–111 , 2008, https://doi.org/https://doi.org/10.1016/j.trd.2007.12.003
, A review on air emissions assessment: Transportation ,J. Clean. Prod. , Vol. 194 ,pp 673–684 , 2018, https://doi.org/https://doi.org/10.1016/j.jclepro.2018.05.151
, Issues in sustainable transportation ,Int. J. Glob. Environ. Issues. 6 , Vol. 331 , 2006, https://doi.org/https://doi.org/10.1504/IJGENVI.2006.010889
, Addressing Sustainability in Transportation Systems: Definitions, Indicators, and Metrics. J. Infrastruct. Syst. , Vol. 11 ,pp 31–50 , 2005, https://doi.org/https://doi.org/10.1061/(ASCE)1076-0342(2005)11:1(31)
, - New registrations of electric vehicles in Europe, https://www.eea.europa.eu/ims/new-registrations-of-electric-vehicles, [Accessed: 5 August 2022]
- A European Green Deal, https://ec.europa.eu/info/strategy/priorities-2019-2024/european-green-deal_en, [Accessed: 5 August 2022]
- A European Strategy for low-emission mobility, https://ec.europa.eu/commission/presscorner/detail/et/MEMO_16_2497, [Accessed: 5 August 2022]
Green innovation in technology and innovation management - an exploratory literature review: Green innovation in technology and innovation management ,RD Manag. , Vol. 42 ,pp 180–192 , 2012, https://doi.org/https://doi.org/10.1111/j.1467-9310.2011.00672.x
, New business models for electric cars—A holistic approach ,Energy Policy , Vol. 39 ,pp 3392–3403 , 2011, https://doi.org/https://doi.org/10.1016/j.enpol.2011.03.036
, - Global EV Outlook: Understanding the Electric Vehicle Landscape to 2020, https://www.ourenergypolicy.org/wp-content/uploads/2013/09/GlobalEVOutlook_2013.pdf, [Accessed: 5 August 2022]
- Zero-Emission Vehicle Program | California Air Resources Board, https://ww2.arb.ca.gov/our-work/programs/zero-emission-vehicle-program/about, [Accessed 5 August 2022]
- How China raised the stakes for electric vehicles, https://www.weforum.org/agenda/2018/12/how-china-raised-the-stakes-for-electric-vehicles, [Accessed: 5 August 2022]
- , Mobile App Support for Electric Vehicle Drivers: A Review of Today’s Marketplace and Future Directions, In: Kurosu, M. (ed.) Human-Computer Interaction. Applications and Services, 2013
Understanding customer satisfaction and loyalty: An empirical study of mobile instant messages in China ,Int. J. Inf. Manag. , Vol. 30 ,pp 289–300 , 2010, https://doi.org/https://doi.org/10.1016/j.ijinfomgt.2009.10.001
, - , Developing Innovative Services: The Case of the Airport Environment, In: Douligeris, C., Polemi, N., Karantjias, A., and Lamersdorf, W. (eds.) Collaborative, Trusted and Privacy-Aware e/m-Services
- , Recommender system for drivers of electric vehicles, In: 2011 3rd International Conference on Electronics Computer Technology, 2011
- , Electric Vehicle Charging Process and Parking Guidance App., Energies, 2019
Real-Time Forecasting of EV Charging Station Scheduling for Smart Energy Systems ,Energies , Vol. 10 (377), 2017, https://doi.org/https://doi.org/10.3390/en10030377
, Investigating the Prospect of Leveraging Blockchain and Machine Learning to Secure Vehicular Networks: A Survey ,IEEE Trans. Intell. Transp. Syst. , Vol. 23 ,pp 683–700 , 2022, https://doi.org/https://doi.org/10.1109/TITS.2020.3019101
, - , Android-Based Monitoring System for Electric Vehicle with Notification Services (AMOSEVENS), SSRN Electron. J., 2018
Coordination and payment mechanisms for electric vehicle aggregators ,Appl. Energy. , Vol. 212 ,pp 185–195 , 2018, https://doi.org/https://doi.org/10.1016/j.apenergy.2017.12.036
, - , Framework for Optimizing the Charging Time of Electric Vehicles in Public Supply Station Deployed in Smart Cities, In: 2018 IEEE 5th International Congress on Information Science and Technology (CiSt), 2018
Optimal Day-Ahead Charging Scheduling of Electric Vehicles Through an Aggregative Game Model ,IEEE Trans. Smart Grid. , Vol. 9 ,pp 5173–5184 , 2018, https://doi.org/https://doi.org/10.1109/TSG.2017.2682340
, - , Charging scheduling with minimal waiting in a network of electric vehicles and charging stations, In: Proceedings of the Eighth ACM international workshop on Vehicular inter-networking - VANET ’11, 2011
- , Research on Gas Station Selection Support System with Given Refueling Volume, Adv. Mater. Res. 424–425, 2012
Scenario generation for electric vehicles’ uncertain behavior in a smart city environment ,Energy , Vol. 111 ,pp 664–675 , 2016, https://doi.org/https://doi.org/10.1016/j.energy.2016.06.011
, - , A Route Planner Service with Recharging Reservation: Electric Itinerary with a Click, IEEE Intell. Transp. Syst. Mag. 8, 2016
A Framework for Electric Vehicle (EV) Charging in Singapore ,Energy Procedia , Vol. 143 ,pp 15–20 , 2017, https://doi.org/https://doi.org/10.1016/j.egypro.2017.12.641
, - , Rapid-Charging Navigation of Electric Vehicles Based on Real-Time Power Systems and Traffic Data, IEEE Trans. Smart Grid. 5, 2014
Digital Transformation - A Primer for Practitioners ,IEEE Softw. , Vol. 37 ,pp 13–21 , 2020, https://doi.org/https://doi.org/10.1109/MS.2020.2999969
, Understanding digital transformation: A review and a research agenda ,J. Strateg. Inf. Syst. , Vol. 28 ,pp 118–144 , 2019, https://doi.org/https://doi.org/10.1016/j.jsis.2019.01.003
, How to select and how to rank projects: The Promethee method ,Eur. J. Oper. Res. , Vol. 24 ,pp 228–238 , 1986, https://doi.org/https://doi.org/10.1016/0377-2217(86)90044-5
, Note—A Preference Ranking Organisation Method: (The PROMETHEE Method for Multiple Criteria Decision-Making) ,Manag. Sci. , Vol. 31 ,pp 647–656 , 1985, https://doi.org/https://doi.org/10.1287/mnsc.31.6.647
, Analytical Hierarchy Process and PROMETHEE as Decision Making Tool: A Review ,IOP Conf. Ser. Mater. Sci. Eng. , Vol. 505 ,pp 012085 , 2019, https://doi.org/https://doi.org/10.1088/1757-899X/505/1/012085
, Implementation of machine learning based real time range estimation method without destination knowledge for BEVs ,Energy , Vol. 172 ,pp 1179–1186 , 2019, https://doi.org/https://doi.org/10.1016/j.energy.2019.02.032
, Relative measurement and its generalization in decision making why pairwise comparisons are central in mathematics for the measurement of intangible factors the analytic hierarchy/network process ,Rev. Real Acad. Cienc. Exactas Fis. Nat. Ser. Mat. , Vol. 102 ,pp 251–318 , 2008, https://doi.org/https://doi.org/10.1007/BF03191825
, - , The Analytic Network Process. In: Decision Making with the Analytic Network Process, Springer US, 2006
Application of PROMETHEE method for green supplier selection: a comparative result based on preference functions ,J. Ind. Eng. Int. , Vol. 15 ,pp 271–285 , 2019, https://doi.org/https://doi.org/10.1007/s40092-018-0289-z
, A combined AHP-PROMETHEE approach for selecting the most appropriate policy scenario to stimulate a clean vehicle fleet ,Procedia - Soc. Behav. Sci. , Vol. 20 ,pp 954–965 , 2011, https://doi.org/https://doi.org/10.1016/j.sbspro.2011.08.104
, - , Using the PROMETHEE multi-criteria decision making method to define new exploration strategies for rescue robots, In: 2011 IEEE International Symposium on Safety, Security, and Rescue Robotics, 2011
- , Information Security Management Systems: A Novel Framework and Software as a Tool for Compliance with Information Security Standards, Apple Academic Press, 2018
Optimized Electric Vehicle Charging With Intermittent Renewable Energy Sources ,IEEE J. Sel. Top. Signal Process , Vol. 8 ,pp 1063–1072 , 2014, https://doi.org/https://doi.org/10.1109/JSTSP.2014.2336624
, Scheduling strategy of electric vehicle charging considering different requirements of grid and users ,Energy , Vol. 232 ,pp 121118 , 2021, https://doi.org/https://doi.org/10.1016/j.energy.2021.121118
,