In many remote areas that are not electrified, the efficient use of renewable energy supply (RES), isolated systems have been developed that combine photovoltaic energy and wind energy and combining these in turn with diesel systems and storage systems such as batteries[1].
Some institutions are often characterized by peculiar energy requirements. In fact, they must be in operation continuously during the day, every day of the year [2]. Hence the need for systems that are permanently monitoring the state of the energy systems. In the case of renewable systems, it is the battery that must deliver the state of health in which it is found to project the acquisition of new ones.
Lead acid batteries are widely used as a primary source of energy in some stationary systems and electric vehicle due to their lower cost, and recycling efficiency and will remain in the near future. Despite this advantage, these batteries are prone to acute and chronic defects due to continuous use and may develop various faults during use, such as electrolyte depletion, sulfonation, etc. Therefore, it is expected to continuously monitor the state of health (SOH) and state of charge (SOC) of the battery [3]. Among the techniques used to determine the SOH are, among others, those indicated in Table 1.
State of the art of state of health measurement techniques
Method to determine the state of health | Method details |
---|---|
Two pulse load test | This experimentation was carried out by Colleman et al. 2008 [4] with 7 lead acid batteries. The battery is subjected to short pulses of 10 A during the discharge process for 17 Ah batteries and the voltage drop in V is measured, which increases as the battery is more discharged. |
Electrochemical impedance applying the cross-correlation technique | Electrochemical Impedance Spectroscopy (using the cross-correlation technique to previously trained data) was developed by Gücin and Ovacik [5] and it is a powerful test technique that is widely applied to electrochemical cells to determine the frequency response of the cell impedance. The electrochemical impedance meter is very expensive, although it has the advantage of being done online. |
Amp hour count | It is the most widespread technique to measure the battery charge and from this deduce the SOH, by counting amps, the amp count is reduced as the battery delivers charge-discharge cycles [6]. It was also experienced by Wetz et al. [7]. |
SMO- Slide mode observers | In [8], an estimation scheme is presented for the combined estimation of SOC and SOH of lithium-ion battery cells by applying the sliding mode theory in electrochemical models. |
X-Ray Analysis Scanning Electron Microscopy | It allows visualizing the changes suffered by the electrodes due to the use of the battery [9]. |
Several parameters contribute to battery degradation, such as temperature, charge/discharge profiles, and inherent battery characteristics [10]. In general, the SOH is related to the physical quantities that control the electrochemical process within the battery. Therefore, any parameter that varies with the use of the battery could be considered as an indication of the SOH of the battery. So battery models are generally electrochemical, circuit-based equivalent, performance-based, and analytical models [11]. The parameters that generally determine the quality of a battery are subject to its application, but in general terms, the most important and what researchers focus their studies on are: the relationship of life cycles to a depth of discharge, battery lifespan, The state of health [12], the state of charge (SOC) [13] and current density [14].
To measure or project these parameters, techniques such as spectroscopy used in biomedical and chemical analysis are used, such as the case of Chen et al. [15], who propose a 10-bit digital impedance converter with which it is possible to directly measure the magnitude and phase of the impedance to digital codes for a range between 0.1 mHZ and 100 kHz, a technique also used in Shabbir et al. [16] using frequencies between 50 mHz and 10 kHz, range in which it is possible to obtain answers related to the dielectric and electrochemical properties of the system, although the frequency range between 10 mHz and 100 kHz has been experimented with for synodal signals with an amplitude of 5 mV in Li-ion batteries [17].
Rada et al. [18] analyze the behavior of lead-acid batteries using the appropriate Kramers-Kronig relationships to verify whether the experimental impedance data using electrochemical impedance spectroscopy belong to a linear and real electrical equivalent circuit or not, they also verify components in the electrodes using diffraction X-rays and give an estimate of the final percentages of a worn battery in which almost 60% of the composition is lead sulfate.
Most of the research is focused on lithium batteries and uses techniques that could be explored in other types of batteries, some techniques are expensive, others require the disassembly of the material to analyze the composition of the electrodes, and their techniques are not as accurate, but more economical as is the case with direct terminal measurement of current voltage and temperature measurement.
The relation of the variation of the optical transmittance of the batteries as they lose capacity, which is verified from the ampere counting technique, has not been worked on in the consulted literature.
In a previous work, the experimental setup was developed and published in [19]. In this new project, it was sought to predict the state of health of batteries from experimental data by adjusting a portion to generate a classification.
A method of classifying the SOH is proposed from the transmittance values obtained from the electrolyte of each of the four central cells of the battery. A widely developed classifier in the literature is the Bayesian classifier that is a naturally probabilistic method that performs classification tasks based on the class membership probabilities [20] that uses a Gaussian distribution as an a priori distribution function of the training data [21].
The proposed classification is framed in the grouping of four different classes, which manage four SOH intervals from the transmittance measured for the electrolyte with different states of use. Therefore, four input features are presented in the training to generate the prior probability constituted by Gaussian distributions.
The optical method used and the forced electrical wear used are defined below, as well as the forms of prediction of the state of health of the batteries.
The embedded system performs permanent monitoring of the current to count the amps consumed until the voltage drops to a certain value where the electronic load cannot supply the programmed current or the active battery voltage reaches approximately 10.5 V as a deep discharge. And the charging process starts again to complete a complete cycle. The implemented scheme is shown in Figure 1 (previously developed and published in [19]). In this the electronic load control measures the voltage in a 100 W and 1-ohm resistor connected to the emitter output of the IGBT, the battery current is in proportion to the measured voltage, and when the battery is discharging the voltage between collector and emitter is reduced to keep the voltage in the resistor RL constant and therefore the current through it, also constant, the comparator AO serves as a control element on the voltage of the IGBT gate which at the same time increases or decreases, in the case of discharge, the voltage VCE. The embedded system captures the current and adjusts the op-amp voltages according to the desired current.
General scheme of the experimental setup [19]
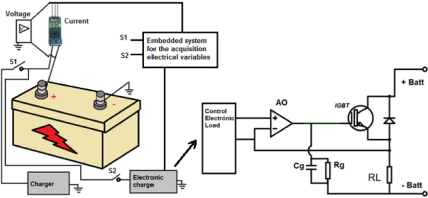
The electronic maintains an almost constant current of around 6 Ah (previously developed and published in [19]) and drops a little at the end of the charge when the measured voltage is approximately 10.5 V. The data is obtained from a data acquisition that consists of an embedded system based on Arduino Nano, with a micro SD storage system and by serial communication on the PC using Matlab®, in addition to a system of voltage divider isolated by operational amplifiers that allow measuring voltages higher than 5 volts and lower than 22 V.
The work that was developed, finally considered the analysis for 6 batteries and 1 additional destroyed new to analyze the initial structure of the electrodes using scanning electron microscopy (SEM), whose maximum initial capacity, when they were new, was 42 Ah, the data acquired through an embedded system and the electronic load designed from an IGBT as a power control element.
100% depth of discharge (DOD) cycles were performed based on 100% discharge when the battery drops to a voltage of 10.5 volts, discharge current was performed at rates of 14.2% of maximum rated capacity, and intercalated discharges of 5% of the nominal capacity to adjust the C20 standard, which is indicated as the maximum charge divided by 20 and which allows the value of the charge to be recorded in the previous charge and discharge cycle at a higher current.
As mentioned before, discharge tests are performed at C3 or 14.2% of the maximum battery capacity. In the case of 42 Ah batteries, the discharge was carried out at 6 A, it should be noted that this relationship is maintained regardless of the state of health (SOH) due to the discharges made in each cycle, the amp count is performed by ensuring of the current in the electronic load through the data acquisition system and using eqs. (1), (2) and (3), the SOC during the load, SOC during the discharge and the SOH are obtained, respectively:
Where SOC0 corresponds to the charge that the battery initially had whether it was in the process of charging or discharging, Qmax is the maximum charge of the new battery, and Qmax(k) is the maximum charge after the kth charge-discharge cycle.
Figure 2 (previously developed and published in [19]) shows the internal system of the camera implemented for measuring the transmittance that allows the electrolyte to pass through. This consists of an SHT10 sensor, which is a two-wire interface digital sensor that allows measuring temperature values in the range of 10 °C to 80 °C with a precision of ±0.5 °C, a UVC lamp with a narrow spectral range maximized at 254 nm, a quartz cuvette containing the electrolyte to be measured and a 254 nm UVC sensor. The chamber is closed during measurements. The distance between the lamp and the electrolyte is 0.5 cm and between the electrolyte and the sensor is 1 cm. The transmittance ratio is made by taking as reference the UVC reception of the sensor when it passes through the electrolyte, about the incident signal of the cuvette without the electrolyte.
Camera for the measurement of UVC 254 nm transmittance [19]
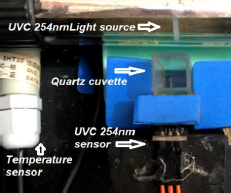
The values of % transmittance and %SOH for all the samples acquired for each of the four cells of the analysed battery (cells 2 to 5) can be seen in Figure 3a and the average of all the measurements of each cell for a SOH, in particular, can be seen in Figure 3b.
Transmittance % data for each SOH value: Acquired data (a); Averaged data of each cell (b)
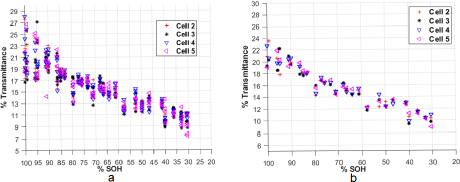
The independent variable during data acquisition is %SOH and the dependent variable is % transmittance. Since what is sought is to determine the %SOH from the % of transmittance acquired, a classification method must be sought for the forecast of the SOH that has been given transmittance data. So, grouping the points, without considering that they come from different cells, they are marked on the plane with the independent variable on the x-axis for the % transmittance as shown in Figure 4.
Experimental data with the change of axes for classification
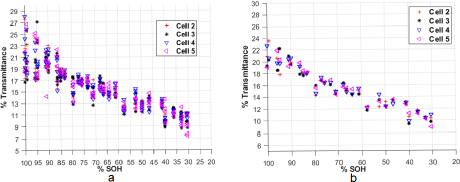
One of the pattern recognition methods is statistical classification in which the posterior probability (eq. (4) of a sample belonging to a certain class is estimated by evaluating the prior probability of this sample in the process of class segmentation and evaluated according to Bayes's theorem [22] which determines that:
The probability of each class defined by P(ωi|x where x (feature vector), P(x|ωi) is the prior probability for the data used in training. For example, if there are 2 classes, the total probability is obtained, which depends on the contribution of each class (eq. (5)).
The Bayesian classifier can be set for the two classes as:
if P(ω1|x)P(ω2|x), x is classified by ω1; if P(ω2|x)P(ω1|x), x is classified by ω2;
The method used for pattern recognition performs better if the appropriate features are chosen, so it is important to represent the data to be analyzed on a feature plane [23]. It implements a univariate and symmetric Gaussian distribution around zero (eq. (6)):
Where p(x,xi) is the probability density and compares it with a Laplacian distribution Figure 5, in which the dotted line is the Gaussian and the upper continuous is the Laplacian signal and between both, the real signal for different levels of the signal analyzed in this case the superficial EMG signals.
The approximation of the signals to a Gaussian distribution rather than a Laplacian one is observed, this phenomenon is repeated for many real phenomena.
Gaussian and Laplacian distribution
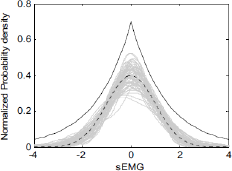
Using a Gaussian distribution for two characteristic axes, the mean, variance, and covariance must be found to determine the line or trend of the data.
Generalization of Gaussian distributions [23] can be rewritten as follows:
where μ = E[x] is the mean expected value:
∑ = E[(x - μ)(x - μ)T] is the covariance matrix
Case 1: If σ12 = 0 and σ grouped data forming a circumference centered on µ;
Case 2: If σ12 = 0 and the data form an ellipse with major axis x1;
Case 3: If σ12 = 0 and the data form an ellipse with major axis x2;
Case 4: If σ12≠0 and the data form an ellipse with major axis forming an angle between x1 y x2.
This work takes into account part of the work developed in [19] but improves the quality of the samples, increases the number of samples and provides a Bayesian classification method to determine the state of health from the measured UVC transmittance and taking into account 5 classes or SOH ranges, the SEM analysis of the electrode of one of the batteries experienced is also carried out as useful life is lost.
On the other hand, although there is no experimental result that demonstrates the relationship of the possible presence of lead sulfate species in the electrodes with respect to the electrolyte, it could be observed that while the energy capacity of the battery is lost due to the obstruction of lead oxide lead and lead by sulfate species, there is also a variation in the optical transmittance ewn the electrolyte.
The process of charging and discharging in the battery causes its electrodes to undergo structural changes due to the accumulation of lead sulfate that cannot be released, exhausting the effective area of the plates that act as anode and cathode. Especially an accumulation of lead sulfate is observed in the spongy lead plates that serve as the anode and that when they are not in use, they present a micrometric structure (scale of 10 μm) like the one seen in Figure 6a and the structural change with use when the battery has depleted 70% of its initial capacity Figure 6b, recorded by an Scanning electron microscopy (SEM).
Battery electrode structural changes recorded by SEM: New battery (a); Battery with 70% of use (b)
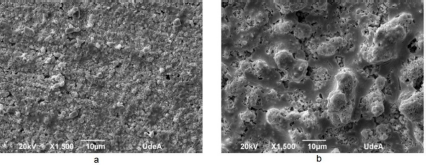
Although the electrolyte presents a visual change due to the detachment of the electrode particles, these remain at the bottom of the battery due to their density, leaving the electrolyte on the surface in a translucent manner. Figure 7a shows a surface electrolyte sample taken from a new battery. Contrasting with Figure 7b in which the surface sample is taken but from a used battery, in a visual approximation no differences between both electrolytes are appreciated, including the battery electrolyte. The new battery Figure 7c, also becomes translucent like the previous ones, the opposite happens with electrolyte taken from the bottom (Figure 7d) of the used battery which is quite cloudy just after it is extracted and it becomes more transparent as solid particles of higher density are precipitated (Figure 7e). For this reason, a non-visual sample analysis method was used and manage a spectral range in which you could appreciate the differences in the surface electrolyte that you have access to without destroying the battery.
Using a UVC sensor outlined in the previous section, it is possible to experiment with samples subjected to a wavelength of 254 nm, passing the light beam through quartz cuvettes such as those in Figure 7, but taking the surface electrolyte and with different levels of SOH.
Electrolyte tone at the bottom and surface of the battery: New battery surface electrolyte (a); Used battery surface electrolyte (b); New battery bottom electrolyte (c); Used battery bottom electrolyte (d); Same electrolyte of image d, after 5 minutes (e)
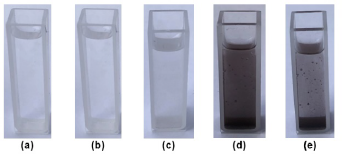
Each battery cell delivers a transmittance value that represents a characteristic from the % transmittance to predict the %SOH. This is achieved by segmenting the transmittance for each of the cells analyzed (cells 2 to 5) and being able to generate four transmittance characteristics, which allow the separation between the classes of each interval of the SOH as shown in Figure 8, where for dimensional reasons only cells 2, 3 and 4 are graphed.
Transmittance in three battery cells grouping different health state ranges
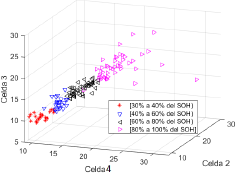
In the end, the Bayesian classifier presents a good classification handling four intervals of transmittance % with only 2, which indicates a correct classification of 98.5%, erroneous samples in the classifier test, as indicated by the confusion matrix in Figure 9, the training time it was 135 seconds. The indices of the confusion matrix (see Table 2) indicate the selected intervals for the %SOH.
Bayesian classifier confusion matrix
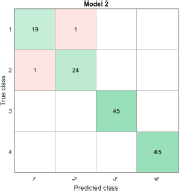
Representation of the indices of the confusion matrix for 4 output intervals each indicating a class or range of %SOH.
Index | % Variable representing the index |
---|---|
1 | SOH between [30% and 40%) |
2 | SOH between [40% and 60%) |
3 | SOH between [60% and 80%) |
4 | SOH between [80% and 100%] |
According to the results of the SEM microscopy, it can be interpreted that the presence of sulfate species that adhere to the electrode and that account for the reduction in the health status of the battery, can influence the variation of the optical transmittance of the electrode. Electrolyte due to species that remain suspended in the latter.
The literature consulted does not address this type of characterization of battery electrolytes, which makes it a novel method applied to these compounds. The classification of the state of health through the measurement of UVC transmittance is still exploratory method that can be used in the analysis of the state of health of other types of liquid electrolyte batteries different from those reported in this paper.
Optimal management energy system and control strategies for isolated hybrid solar-wind-battery-diesel power system ,Emerg. Sci. J. , Vol. 5 (2),pp 111–124 , 2021, https://doi.org/https://doi.org/10.28991/esj-2021-01262
, Machine Learning-Based Monitoring Method for the Electricity Consumption of a Healthcare Facility in Italy ,J. Sustain. Dev. Energy, Water Environ. Syst. Orig. , Vol. 10 (4),pp 1–22 , 2022, https://doi.org/https://doi.org/https://doi.org/10.13044/j.sdewes.d10.0421
, SoH Analysis of the Lead Acid (LA) Battery Using a Novel Vibration Tool ,ICPEE 2021 - 2021 1st Int. Conf. Power Electron. Energy ,pp 9–14 , 2021, https://doi.org/https://doi.org/10.1109/ICPEE50452.2021.9358741
, An improved battery characterization method using a two-pulse load test ,IEEE Trans. Energy Convers. , Vol. 23 (2),pp 708–713 , 2008, https://doi.org/https://doi.org/10.1109/TEC.2007.914329
, Online Impedance Measurement of Batteries Using the Cross-Correlation Technique ,IEEE Trans. Power Electron. , Vol. 35 (4),pp 4365–4375 , 2020, https://doi.org/https://doi.org/10.1109/TPEL.2019.2939269
, Estimation of state of charge, unknown nonlinearities, and state of health of a lithium-ion battery based on a comprehensive unobservable model ,IEEE Trans. Ind. Electron. , Vol. 61 (3),pp 1335–1344 , 2014, https://doi.org/https://doi.org/10.1109/TIE.2013.2259779
, Electrochemical energy storage devices in pulsed power ,IEEE Trans. Plasma Sci. , Vol. 42 (10),pp 3034–3042 , 2014, https://doi.org/https://doi.org/10.1109/TPS.2014.2334396
, Combined estimation of State-of-Charge and State-of-Health of Li-ion battery cells using SMO on electrochemical model ,Proc. IEEE Work. Appl. Comput. Vis. , 2014, https://doi.org/https://doi.org/10.1109/VSS.2014.6881140
, Reactivation of dead sulfide species in lithium polysulfide flow battery for grid scale energy storage ,Nat. Commun. , Vol. 8 (1),pp 462 , 2017, https://doi.org/https://doi.org/10.1038/s41467-017-00537-0
, Residual lifetime prediction for lithium-ion battery based on functional principal component analysis and Bayesian approach ,Energy , Vol. 90 ,pp 1983–1993 , 2015, https://doi.org/https://doi.org/10.1016/j.energy.2015.07.022
, Local probabilistic model for Bayesian classification: A generalized local classification model ,Appl. Soft Comput. J. , Vol. 93 ,pp 106379 , 2020, https://doi.org/https://doi.org/10.1016/j.asoc.2020.106379
, Lifetime estimation tool of lead-acid batteries for hybrid power sources design ,Simul. Model. Pract. Theory , Vol. 54 ,pp 36–48 , 2015, https://doi.org/https://doi.org/10.1016/j.simpat.2015.03.001
, Energy management of a hybrid photovoltaic-wind system with battery storage: A case report ,J. Sustain. Dev. Energy, Water Environ. Syst. , Vol. 7 (3),pp 399–415 , 2019, https://doi.org/https://doi.org/10.13044/j.sdewes.d6.0233
, Electrochemistry of thin-plate lead-carbon batteries employing alternative current collectors ,J. Power Sources , Vol. 352 ,pp 194–207 , 2017, https://doi.org/https://doi.org/10.1016/j.jpowsour.2017.03.129
, Novel 10-Bit Impedance-To-Digital Converter for Electrochemical Impedance Spectroscopy Measurements ,IEEE Trans. Biomed. Circuits Syst. , Vol. 11 (2),pp 370–379 , 2017, https://doi.org/https://doi.org/10.1109/TBCAS.2016.2592511
, State of Health Estimation of Li-Ion batteries using Electrochemical Impedance Spectroscopy ,Transp. Electrif. Conf. Expo ,pp 108–112 , 2017, https://doi.org/https://doi.org/10.1109/ITEC.2017.7993255
, Controllable synthesis of spinel lithium nickel manganese oxide cathode material with enhanced electrochemical performances through a modified oxalate co-precipitation method ,J. Power Sources , Vol. 274 ,pp 1180–1187 , 2015, https://doi.org/https://doi.org/10.1016/j.jpowsour.2014.10.154
, Structural and electrochemical investigations of the electrodes obtained by recycling of lead acid batteries ,J. Electroanal. Chem. , Vol. 780 ,pp 187–196 , 2016, https://doi.org/https://doi.org/10.1016/j.jelechem.2016.09.025
, Changes in electrolyte transmittance at 254 nm according to the state of health of lead acid batteries ,Inmateh-Agricultural Eng. , Vol. 68 (3),pp 599–606 , 2022, https://doi.org/https://doi.org/10.35633/inmateh-68-59
, Bayesian classifier with multivariate distribution based on D-vine copula model for awake/drowsiness interpretation during power nap ,Biomed. Signal Process. Control , Vol. 56 ,pp 101686 , 2020, https://doi.org/https://doi.org/10.1016/j.bspc.2019.101686
, Local probabilistic model for Bayesian classification: A generalized local classification model ,Appl. Soft Comput. J. , Vol. 93 ,pp 106379 , 2020, https://doi.org/https://doi.org/10.1016/j.asoc.2020.106379
, Bayesian classifier with multivariate distribution based on D-vine copula model for awake/drowsiness interpretation during power nap ,Biomed. Signal Process. Control , Vol. 56 ,pp 101686 , 2020, https://doi.org/https://doi.org/10.1016/j.bspc.2019.101686
, , Introduction to Pattern Recognition: A Matlab Approach , 2009, https://doi.org/https://doi.org/10.1016/B978-1-59749-272-0.50003-7
,