Global economic prosperity is dependent on strong infrastructure networks that safely and reliably connect people and places. The Australian Government is investing a record AUD$100 billion over the next 10 years through its rolling infrastructure plan, which requires increasingly data-driven rationales and principles in decision making [1]. Digital engineering has emerged as a targeted approach to provide such evidence-based decision making, towards deriving better business, project and asset management outcomes.
The term digital engineering is increasingly referred to as “the art of creating, capturing and integrating data using a digital skillset” [2]. Digital engineering can connect emerging data curating and processing technologies with existing structured data sets already in existence. It can enable more collaborative and productive methods of project delivery and management of assets throughout an asset’s lifecycle. As phenomena such as real-time asset monitoring and ‘big data’ become prolific, digital engineering enables designers through to managers to gain a deeper understanding of asset performance including strengths and vulnerabilities, creating an evidence-base for cooperation and collaboration [3]. For these reasons, digital engineering is widely considered a significant opportunity to improve resilient decision-making, addressing project delivery challenges, and allowing for more timely delivery with better outcomes [4]. There is emerging consensus on the critical role of digital engineering in resilient decision making [5] and Figure 1 illustrates how digital engineering and information technology contributes to resilient decision making (Figure 1).
Digital engineering and resilient decision making [5]

Considering the benefits of digital engineering, this paper presents the first step in building a digital infrastructure asset management platform, by establishing a common language to discuss critical asset information requirements for road infrastructure and their interrelationships. The paper documents a series of semi-structured interviews with senior asset management decision-makers from road research institutes, and state and local government authorities in South East Queensland, Australia regarding their lived experiences in applying digital engineering in their workplaces. The research findings are discussed in relation to the topics of: managing complex data; current practices and outlook; asset information requirements; and, interrelationships between types – or ‘categories’ – of asset information requirements.
In this paper, the authors argue that an Asset Information Requirement (AIR) matrix is an essential decision support tool for authorities and practitioners to evaluate ‘when’ (i.e. at the right time), and ‘where’ (i.e. for the right locations) digital infrastructure data can enable resilient outcomes. The authors conclude with reflections on potential areas for further investigation, and the future potential for digital engineering to improve the resilience of road infrastructure through improved asset management and maintenance. The authors intend that this paper is a useful resource for academics, practitioners, and decision-makers to create a common language platform for engaging in digital engineering conversations. It will also inform subsequent efforts in developing a digital infrastructure asset management platform to achieve targets related to the United Nations Sustainable Development Goal (UNSDG) 9 on building resilient infrastructure.
Digital engineering (DE) can be described as the “convergence of emerging technologies such as Geographic Information Systems (GIS) and related systems to derive better business, project and asset management outcomes” [6]. The application of digital engineering for the infrastructure sector is a substantial conversation with many component discussions, as assets can vary in form and function. For example, assets can be linear (e.g. roads and rail) or vertical (e.g. buildings). They can also be a discrete entity (e.g. a stand-alone building), or act as part of a broader network (e.g. a road network).
Along this spectrum, there are many claims that digital engineering technologies are making significant improvements in project delivery in infrastructure asset management projects, globally [7], [8] and locally [9]. This includes, for example, offering significant benefits to infrastructure sectors such as railway, road and bridge sectors to integrate data about a construction and future function to develop the most efficient methods of delivery and operation [10]. As disruptions such as Covid19 affect supply and demand for materials in complicated ways, digital engineering approaches can enable supply chains to meet the challenges of project delivery while allowing projects to be delivered faster, better and cheaper [6]. While DE has emerged as a targeted approach for improved asset management, it is more than just using online tools and storing asset data electronically. To create a manageable DE environment, it is critical to first identify ‘when’ and under ‘what’ circumstances in the asset life cycle, digitised asset information requirements can inform data-driven rationales that enable authorities and practitioners to make evidence-based decisions. The quality of data for these identified ‘critical asset information requirements’ can then be addressed and prioritised for ongoing ‘data curation’. The outcomes of enabling such a managed DE environment span the whole asset lifecycle, from improved planning to procurement, design, construction, operation and end-of-life decommissioning.
Resilience is recognised as the capacity to adapt to reduce the vulnerability of assets in the face of disruption [11]. In the infrastructure sector, there is increasing evidence of climate-change-related disrupted asset functions, reduced lifespans, and increasing maintenance costs [12]. Linear infrastructure networks are particularly vulnerable to extreme weather events [13]. Decision-makers are subsequently seeking to understand and manage risks related to climate change for infrastructure and to improve infrastructure resilience [11], [14]. While traditional resilience approaches focus on increased strength and rigidity in infrastructure, there is also a growing appreciation of the need to prioritise infrastructure adaptability and flexibility [11].
Within this context of vulnerable infrastructure, there are increasing calls for digital engineering approaches to manage critical information requirements, towards accurately informing resilience planning efforts [4]. This includes improved monitoring and modelling to improve predictions of likely points of failure, enabling the accommodation of such failures, improving the resilience of individual assets, and larger networks to current and future disruptions [15]. There are also calls to develop consistent digital asset management models that facilitate continued flows of information – beyond typical planning/ design/ construction asset lifecycle interfaces – to include asset operation, maintenance, and end-of-life repurposing or disassembly [10], [16].
Digital engineering is being increasingly adopted by the Australian infrastructure sector, especially in rail and road infrastructure. For example, Transport for New South Wales (TfNSW) has committed to the strategic implementation of digital engineering in order to realise more significant infrastructure and customer outcomes. Due to digital engineering ability to transform the way project and asset information are managed (i.e. through the adoption of emerging technologies and new business processes) TfNSW sees potential in using digital engineering to create cost savings and improve productivity across the asset life cycle. TfNSW has developed a digital engineering framework (Figure 2) to provide incremental transition process to deliver projects, develop and manage information about its assets, and finally to becomes a more effective, data-centric organisation [17].
Such design and practice approaches and technologies span the fields of Geographic Information Systems (GIS), Building Information Modelling (BIM), Civil Information Modelling (CIM), Bridge Information Modelling (BRIM) and others [10]. Table 1 highlights the variety of digital engineering tools that can be used for effective management of infrastructure assets, and the variety of benefits including a higher level of data integration, communication and collaboration.
The Digital Engineering framework [17]

Technologies supporting digital infrastructure asset management
Digital process/Model type |
Key features |
Key reference/s |
---|---|---|
Building Information Modeling (BIM) |
|
|
Bridge Information Modeling (BrIM) |
|
|
Bridge Management System (BMS) |
|
|
Computer-aided design (CAD) |
|
|
3D Modelling |
|
[23] |
4D Modelling |
|
[28] |
Multidimensional (nD) Modelling |
|
[29] |
Asset Integrity Management (AIM) |
|
[30] |
Global Positioning System (GPS) |
|
|
Geographic Information Systems (GIS) |
|
Asset information is critical for evidence-based decision-making and developing priorities, being generated and used across the asset life cycle. While a range of digital technologies support infrastructure asset management, it is essential to firstly identify under ‘what’ circumstances and ‘when’ during the life cycle different asset information would be critical for managing resilient road infrastructure. In this research, we sought to prioritise a set of ‘critical’ asset information requirements (AIRs) to better manage road infrastructure. In the following paragraphs key literature is summarised, which formed the basis of the interview questions used in the research.
During an asset’s life cycle, asset information is used to ensure that an asset adheres to quality criteria such as completeness, correctness, consistency, clarity, integrity, and uniqueness [9]. It is therefore critical that this information is reliable and of high quality. For a given asset, information requirements also need to be defined at key project stages from planning through to maintenance, to manage asset condition and performance. Such information consists of data and documents as shown in Figure 3, where graphical data defines an asset graphical attribute (e.g. location, position, and spatial) and non-graphical data comprises attributes that describe the asset’s configuration, condition, operational, maintenance, organisational and financial condition.
Asset information component [9]
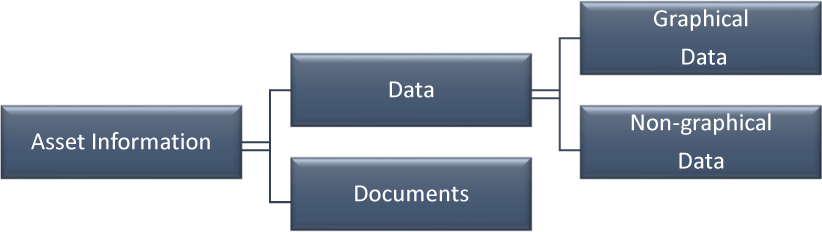
While asset information can offer insights into the asset’s significance or priority in a given scenario, and facilitate users quickly identifying suitable strategies or actions [35] identifying critical asset information requirements can provide authorities and practitioners with even more targeted and effective decision-support. Austroads provides data standards as presented in in Table 2, where key data categories are summarised from Austroads technical reports. However, there is limited discussion of priorities and the application of such data categories in the context of managing for improved resilience. The authors thus sought to build on these categories to consider ‘critical information requirements’.
Data categories and definitions [36]
Data categories |
Brief description |
---|---|
Network |
The road network and its links |
Classification |
The hierarchy and purpose for the links |
Inventory |
The asset register |
Condition |
The condition of the asset |
Demand |
The current user profiles and vehicle volumes |
Utilisation |
The usage of assets |
Criticality |
The importance of assets |
Risk |
The risks associated with assets |
Resilience |
The ability to restore asset service following an event |
Performance |
Finance (the costs of asset ownership)Service (the customer service performance of the asset) |
Access |
Any road user access restrictions |
Works and costs |
The physical work plan/achievement and related estimated/ actual costs |
With regard to the asset life cycle, the phases of ‘conception to disposal’ have been adopted from the Austroads asset management system, as presented in Figure 4. All activities highlighted in Figure 4 are directed towards delivering a service considering the sustainability of risk, cost and performance [37].
While AIRs are used in various phases of the asset life cycle as shown in Figure 4, there is limited research to indicate which AIR type is important for each life cycle phase. The authors sought to address this research gap by drawing on the lived experiences of industrial practitioners to develop a much-needed AIR matrix demonstrating the flow of AIR throughout the asset life cycle.
A qualitative exploratory study was deemed appropriate due to the novelty of digital engineering (DE), and therefore in-depth interviews were undertaken to gain insights from practitioners [38], [39]. Potential participants were invited to participate in semi-structured interviews through a method of snow-ball sampling, whereby interviewees were invited to nominate other potential participants. Invited participants included management and operations personnel with digital engineering/ asset management experience for over 5 years, from Government and industry.
Austroads Asset Management System [36]
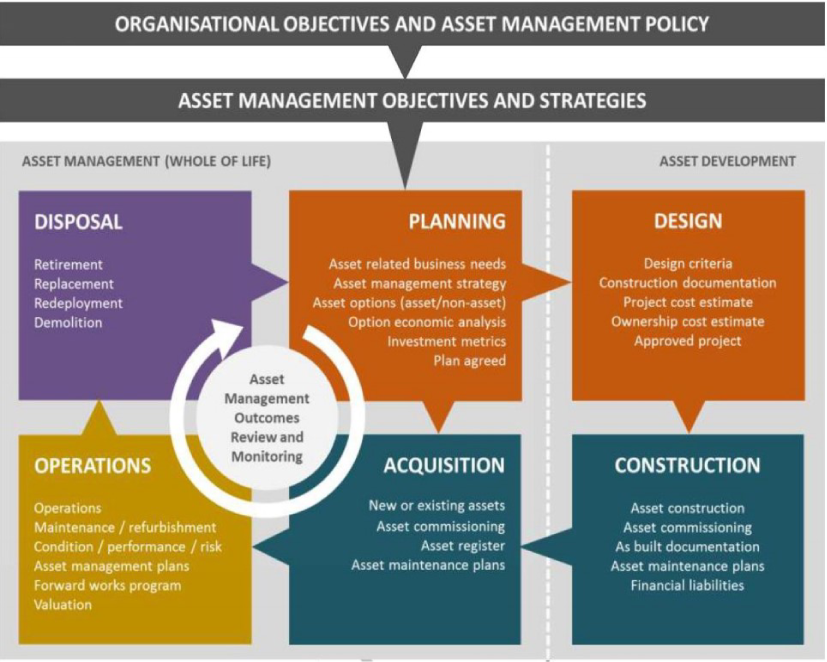
Interviews were conducted in person on a date and time of participant’s choice and convenience. The semi-structured interview questions were developed based on the findings of the literature review, and in total, there were eight in-depth interviews. The summary of the participants is presented in Table 3 with code names for the participants that are used in the findings and discussion section.
While the authors acknowledge that a small number of industry experts were interviewed in this qualitative study, however, it is important to emphasise that in qualitative research: the success of a study is not in the least dependent on sample size, it is not the case that a larger sample necessarily indicates a more painstaking or worthwhile piece of research. Additional interviews can perhaps add to the labour involved instead of contributing to the analysis [40].
Summary of the interview participants
Interview participants (P) |
Organisation |
No of interviews |
Interview duration |
---|---|---|---|
P1: Senior technology leader |
National road research organization |
2 |
90 min |
P2: BIM expert |
State government organization |
1 |
60 min |
P3: Asset management expert |
State government |
1 |
60 min |
P4: Asset management expert |
Local council 1 |
2 |
60 min |
P5: Asset management expert |
Local council 1 |
1 |
60 min |
P6: Asset management expert |
Local council 2 |
1 |
60 min |
Through the initial contextual inquiry questions, digital engineering insights were gathered from practitioners. More detailed questions then explored the types of digital engineering practices currently used in the industry, critical information requirements for decision making and future opportunities. The interview guide is attached in Appendix 1. The initial matrices used for the interviews included the asset functional groups adapted from the Austroads data standards, as shown in Table 2 Additional functional groups were identified from the interview transcripts.
The first step of the data preparation comprised manual transcription of the audio recording by the first author [41]. Data reduction methods were then used to read and analyse the transcribed data [42]. An inductive analysis method of in-vivo coding was conducted to form nodes from the interview data [43]. Through an iterative coding process, the emergent themes were identified. Within the coding process, initially, 155 in-vivo codes were developed labelling the data through a short word or phrase. Then these codes were categorised into 26 higher-order axial codes to deduce the interactions[44]. Finally, four key emergent themes were used to create the selective codes which demonstrated relationships to all categories [45]. Two researchers were involved in the coding process to corroborate the emergent themes and minimise possible bias the data analysis [46].
This section presents the interview and report data and evaluates the criticality of various asset information types for improved decision making. Four emergent topics of discussion were distilled from the thematic analysis, namely: managing complex data; current practices and outlook; key asset information requirements; and, key interrelationships including data structures, interactions and interdependencies. These four themes are used to present and discuss the findings in the following sub-sections. Quotes from interviewees are presented and discussed with references to supporting key literature.
Overall, there was a common appreciation of the need for digital engineering for asset managers to thrive in an increasingly connected, data-driven world. Many of the participants highlighted that they are still at the early phases of applying digital engineering in their areas of work to manage complex data. However, they aspire to integrate more digital approaches in the future. It was generally agreed that digital infrastructure asset management “means a lot of opportunities but at the moment not a lot of reality” (P1). It was commented that even with the abundance of information, it is challenging to make better decisions around performance, risk, and costs (P1). Another participant commented, “So, its fundamentally about making the right decisions, based on a broad range of inputs and understanding context and objectives” (P1). It was evident from all practitioners that there are clear opportunities for digital engineering as a targeted approach in realising better, resilient outcomes in infrastructure asset management.
Digital engineering was described as, “all of the engineering activities that sit around the modelling and data and information capture that can occur across any particular phase of design or construction, operations and maintenance” (P2). It was emphasised by several participants that digital engineering is integral to setting engineering standards and apply engineering standards to design or construction, operations, and maintenance. Digital Twin and BIM were two terms regularly associated with digital engineering during the interviews.
Digital twinning was identified as the latest manifestation of digital engineering, given that it is a digital representation of a physical asset, process, or system, as well as the engineering information that allows us to understand and model its performance. For example, “Digital twin is the new buzz word that came along where you do all that electronic and digitally first. if you put the construction cycle, the digital twin is been advocated heavily now” (P4). The current challenges regarding classes and other incompatibility issues were well-understood by participants in their conversations. Benefits were clear, evidenced in the commentary by P4, “By having that system from end to end, across the life cycle facing there is no need for file conversation and the data could be easily transferred and moved the across the system”.
Building Information Modeling (BIM) was mentioned by all participants as an example of digital engineering in practice. BIM is defined as a process that runs through the entire asset lifecycle [47]; and a Digital representation of physical & functional elements of an asset used for decision making [16]. According to one participant (P2), “if digital engineering was a broader umbrella of digital processes and practices that apply engineering principles and policies, [then] BIM is the data management of the data set that we generate through the design” (P2). Participants shared experiences about their early digital engineering journey being in the form of modelling for resilient road infrastructure projects.
Several participants referred to the SMEC Pavement Management System (PMS) as an example of a robust database designed to store and easily access a large range of information relating to roads, paths, and road inventory items existing in the road corridor. “The PMS is a planning tool that is able to model pavement and surface deterioration due to the effects of traffic and environmental ageing. It can be used to determine long-term maintenance funding requirements and to examine the consequences on network conditions if insufficient funding is available. The PMS is capable of producing accounting reports for asset replacement costs, current written down valuation, and depreciation” (P6).
The integration of digital engineering to the key elements of participants’ functional asset management system was identified as critical, particularly by local council participants (P4, P5, P6). Participants mentioned the criticality of connected maintenance management systems, asset accounting, and infrastructure risk management systems, for predictive modelling and mobility evaluation. For example, “For me, it’s about knowing what we got in terms of the asset register. Its make-up material, diameter, length, whatever it might be geographic positioning, digital asset management is then in our number of transactions, it might be a maintenance, condition assessment, and predictive modelling. There should be conditions performance management” (P6). P2 referred to the local council engaging in significant condition and performance monitoring to evaluate the utilisation of the asset does with regard to whether it meets requirements. That along with asset accounting, risk management, predictive and performance monitoring and maintenance (both proactive and reactive), and condition ratings, require data-rich environments.
All interviewed practitioners shared a variety of digital tools, technologies to manage complex data in managing their infrastructure, however, the described approaches were organic and variable, depending on the champions within the organisations and their individual experiences and expertise with specific systems. In addition to BIM (process) and Digital Twinning mentioned above, tools included Building Information Systems (BIS), Geographic Information Systems (GIS), proprietary pavement management system (PMS), AutoCAD, 2D, 3D, and 12D modelling, Bentley software and Autodesk. Participants collectively aspired to create an open environment to be able to easily share information between native file format design packages into something readily viewable and usable for other purposes. There was a strong consensus across interviews that the variety of technologies should enable practitioners to readily store, analyse, and visualise data to improve decision making.
Interviewees noted that the state government is currently using the building information system (BIS-1996 Oracle database), which is a data structure inventory tool. This contains all available inventory data/ information requirements. The asset management expert from Local Council 2 (P5) also expressed the interest and willingness to use BIM processes. For example, “I have a great interest currently in the BIM process at the moment. With some real value at the moment. Just done some training […] using Navisworks where it talks about clash detection and modelling and things like that. That was for infrastructure, so we know how to go through those processes” (P5). The senior technology leader (P1) added to this conversation they are highlighting the use of cloud systems in place where they are trying to receive all the information in the cloud as well, along with the field investigations and field inspections. Local Council 2 currently uses a combination of systems to store condition data with some links to SAP and ESRI GIS for registering, in the maintenance management system, and in the whole of life costing and performance monitoring or predictive modelling. They also have separate systems like SMEC PMS that manage predictive modelling for road pavements.
The state government organisation participant reflected on their journey of utilising the emerging digital technologies to convert 2D creations into 3D models. The BIM expert (P2) explained, “ the creation and capture of the information is happening through 3D modelling. The generation of 3D models and 2D drawings come from it. The step-change of the moment is moving to try to move away from 2D digital data capture, into a 3D object model” (P2). The participant reflected on the 12D model as world-leading software for surveying, civil and water engineering, “including a powerful programming language, which allows users to build their own options” (P2). Other participants mentioned Bentley software was as influential in accelerating project delivery and improving asset performance for the infrastructure that sustains our economy and our environment. Autodesk was also referred to with regard to generating 3D models.
The asset management expert (P4) considered digital infrastructure asset management as, “an integration of all these to start with the end in mind, to produce useful asset management planning outputs, evidence-based decision making about timing of either planning and maintenance intervention to do capital renewal investments”. The participant also emphasised that the digital landscape and technology are, “purely supporting those outputs the data requirements for the whole of life of infrastructure are independent of the tools and the users should be able to uplift and go into a new piece of technology” (P4).
The extracted information requirements were synthesised into a new ‘Asset Information Requirements’ (AIR) matrix, mapping the use of this information throughout the asset life cycles phases of planning, design, construction, acquisition, operations and disposal. Synthesising the interview transcripts and the literature review findings, the authors distilled the 66 data types into 13 key asset information categories, as summarised in Table 4 (in alphabetical order for each infrastructure data type). Each of the 13 categories was then evaluated (based on the interviews) according to the likelihood of data being available during each of the six life cycle phases [36], namely: planning, design, construction, acquisition, operations, and disposal. The likely availability of data is indicated by a dot in the relevant columns adjacent to the data types in Table 2.
Asset information requirements (AIR) matrix, clustering data types by functional group and across the asset life cycle
Infrastructure data type |
Asset information category |
Data requirements |
Data availability for each life cycle phase |
|||||
---|---|---|---|---|---|---|---|---|
Planning |
Design |
Construction |
Acquisition |
Operations |
Disposal |
|||
Road characteristics |
Classification |
Economic and social category |
⬤ |
⬤ |
|
|
⬤ |
|
Functional priority |
||||||||
Hierarchy |
||||||||
Service profile |
||||||||
Condition |
Bridge |
⬤ |
⬤ |
⬤ |
⬤ |
⬤ |
⬤ |
|
Kerb and channel |
||||||||
Pavement |
||||||||
Pathway/ footpaths |
||||||||
Surface/ elements |
||||||||
Remaining useful life |
||||||||
Unsealed roads |
||||||||
Inventory |
Classification |
⬤ |
⬤ |
⬤ |
⬤ |
⬤ |
⬤ |
|
Components |
||||||||
Design standards |
||||||||
Existing assets |
||||||||
Geometry |
||||||||
Jurisdiction |
||||||||
Location |
||||||||
Name |
||||||||
Unique Identifier |
||||||||
Road cost profile |
Capital renewal/ upgrades |
Capital value |
⬤ |
⬤ |
⬤ |
⬤ |
⬤ |
⬤ |
Residual capital value |
||||||||
Treatment options |
||||||||
Maintenance |
Maintenance cost |
⬤ |
⬤ |
⬤ |
⬤ |
⬤ |
⬤ |
|
Maintenance activities data |
||||||||
Disposal costs |
||||||||
Failure incident management data |
||||||||
Financial valuation |
Financial performance |
⬤ |
⬤ |
⬤ |
⬤ |
⬤ |
⬤ |
|
Replacement cost |
||||||||
Unit rate |
||||||||
Use of life/ Asset life |
||||||||
Road priority |
Criticality |
Importance of that route |
⬤ |
⬤ |
|
|
⬤ |
|
Service profile |
||||||||
Environmental |
Environmental data (weather, flooding, soil types) |
⬤ |
|
|
⬤ |
⬤ |
⬤ |
|
Geographic and geospatial referencing data |
||||||||
Susceptibility to natural hazards (fire) |
||||||||
Network |
Link |
⬤ |
|
|
⬤ |
⬤ |
⬤ |
|
Link sections |
||||||||
Network name |
||||||||
Node |
||||||||
Strength |
||||||||
Resilience |
Access state |
⬤ |
⬤ |
⬤ |
⬤ |
⬤ |
|
|
Damage state- failure mode |
||||||||
Duration |
||||||||
Distance to susceptible environments |
||||||||
Type of material |
||||||||
Risk |
Asset type |
⬤ |
|
⬤ |
|
⬤ |
⬤ |
|
Mitigation measures |
||||||||
Potential risks |
||||||||
Road activity |
Performance |
Asset life |
⬤ |
⬤ |
⬤ |
⬤ |
⬤ |
|
Achievement |
||||||||
Community/ customer input |
||||||||
Fit for purpose |
||||||||
Output |
||||||||
Service performance |
||||||||
Road utilisation |
Axle load |
|
|
|
|
⬤ |
|
|
Bicycles/Pedestrians |
||||||||
Capacity |
||||||||
Community users |
||||||||
Congestion |
||||||||
Freight use |
||||||||
Outputs |
||||||||
Traffic volumes |
||||||||
Traffic growth |
Drawing on interviewees’ reference to several priorities in considering road infrastructure resilience, the data type clusters in Table 4 are discussed in relation to these priorities, namely their characteristics, cost profile, criticality, and role within the road infrastructure network.
This section discusses the three asset information categories of classification, condition, and inventory data. Classification data reflects on the hierarchy and purpose for the links. Functional priority, service profile, hierarchy, economic, and social are useful in planning, design, and operation phases. Participant 2 added to this context by describing a real-world example of priorities when developing a program or project up for funding. Firstly, they classify the network need especially interrogating why they need to do a project in a particular location. To augment the previous step, they collect condition data/ crash history data, as a foundation to decide why they need to put up a project for funding.
As asset condition is the forefront of the planning and acquisition processes, where collecting the right attributes enables users to properly maintain the asset it is critical for monitoring and reducing risk at later stages of the asset life cycle. DE benefits span planning and acquisition, and operational processes. For example, “We just not only want to know the defects and the severity of the defects, condition assessment, determination of how it got left. When we talk about life to intervention, classic integration curve, we are not running it too. Is it condition zero, brand new or both. We basically want to do, there will be a whole heap of maintenance supplied in that period as well. So that’s an intervention point, we get in their re-seal or reconstruct” (P6). This participant also emphasised that identifying intervention points is critical to rolling out timely maintenance strategies.
One of the participants (P2) stated that inventory data and condition data go to the core of the operations. From their perspective, one of the key pieces that come out from the ARMIS system (Road management information system) is the cost element. Therefore, they have to ensure that the necessary pavement asset information is being captured so as to make evidence-based decisions. Inventory data captures the data related to asset register in order to check compliance of the description and definition of the asset’s physical properties. In an asset register, all assets and sub-assets are listed by items such as Name (defines the unique name of the whole road), Unique Identifier (links to one single road or part of the road), Location (locates the road, the most fundamental and critical attribute linked to all road-related data to each other), Jurisdiction (describes the legal status of the road including owner, managing agency and governmental jurisdiction), Geometry (includes size and other geometric characteristics defining the road), Constituents (states the composition and materials of the pavement in groups, pavement type, surface type, base type, sub-base type, subgrade, layer thickness for the above), and Classification (defines road significance and maintenance priorities based on the road function) [9].
This section reflects on the three asset information categories of capital renewal, financial valuation data and maintenance and. Capital renewal/ upgrade and financial valuation are two key cost categories in addition to maintenance costs. Critical renewals/upgrades are supported by critical sub-data types such as capital value, residual capital value, and treatment options. A financial valuation can be divided into replacement cost, use of life/ Asset life, unit rate, and financial performance which are used throughout the asset life cycle. While these functional groups reflects on road cost profile, there are clear relationships with condition data highlighted in the earlier section (road characteristics) as the remaining life can be evaluated based on the condition which will inform the decision regarding intervention.
The asset management expert from local council 2 (P6) reflected that they would treat financial evaluation separately to asset accounting. For example, “we are required under the accounting standards of the local government act. But what we use for the actual replacement cost is quite different. Valuing like examples put back service of pavement, in actual reality, it would be separate. Use for life, replacement cost, unit rate, measuring all square meters, using rest of the inventory data for that, square metre radius for the replacement cost that depreciates” (P6). Thirdly, maintenance costs include labour, material, plants and equipment, and contract, maintenance activities data (maintained activities data - to maintenance inspections, programmed maintenance and cyclic maintenance and reactive maintenance), and end-of-life costs (i.e.: labour, material, plants and equipment and contract). As highlighted above, financial resources are a pivotal factor in asset decisions. Therefore, works and costs are critical in the earlier phase of the asset life cycle.
This section reflects on the five asset information categories of criticality, environment, network, resilience, and risk data. Authorities and practitioners must consider these five categories if they are to improve agility and adaptability of road infrastructure. The criticality of road infrastructure is evaluated through the service profile and importance of the route. It is critical to assess the age of the asset, how it has been functioning, and if there is a natural disaster and damages the road that asset managers need to be conscious about. Within this conversation, the senior technology leader (P1) expressed that criticality and resilience are deeply embedded within the performance. For example, “ We try and build that resilience, so the longevity of life is really key for us. We will continue to research how well certain pavements will react for certain conditions” (P1). This highlights the critical need to invest in ongoing research to discover materials, methods to facilitate an improved level of infrastructure resilience.
Resilience data shows the ability to restore asset services following an event (i.e. a disruption). Participants emphasised the increasing attention to the concept of resilience and the associated concept of biomimicry to create innovative solutions inspired by nature. The asset management expert from local council 2 (P6) mainly focused on flood mitigation drainage assets, beaches, and waterways assets where they are subject to tide and inundation. P6 commented that “In terms of resilience it goes back to the inventory data one of the examples is we got a lot of concrete bridge piles and stormwater pipes and make suffice concrete cover over the reinforcements. That cover eroded over the time or micro-crack or cracking allows saltwater to penetrate and then it gets spoilt and it gets into failure mode”. A clear relationship between resilience and performance and inventory were identified. For example, “ when there is cracking off the concrete and what we have to do is then how it erupts the pile, realign the pile or rip it out. That’s a performance issue. In terms of resilience, for me, it probably comes back to inventory data, the class of pipe, or standards used to construct the asset. From an asset manager, we are yet to come back with evidence around the true impact of an asset. What if it is coming out through our condition assessment” (P6).
Reflecting on the impacts of climate change with regard to road assets, several participants highlighted the critical need for more data and evidence on what mitigations measures can be taken, and how these measures could be informed through an augmented data repository including inventory, condition performance. Within the context of failure mode, these participants noted the criticality of identifying what the failure modes are for, to what percentage of failure, what are the deterioration factors acting upon asset types, what is a factor could be oxidisation, saltwater corrosion, tidal inundation, temperature, humidity, rainfall. It was noted that engineers and asset managers have to carefully assess each of these elements to decide the extent of the impact by those deterioration factors and treatment options. For example, “ specific to the asset type, road pavement- patching, spray sealing, stabilisation reconstruction, then you got the maintenance, crack sealing. Once you got those what our overarching asset management strategy, based on the whole of life cost analysis we are going to apply, maintenance strategy, capital renewal strategy, and then breaking that down” (P4).
An overall environmental condition can be captured by considering items such as geographical data (i.e. topography), climate data (i.e. temperature regime, rainfall), and construction data [9]. Most participants concluded the following three key environmental data types as critical for planning, acquisition, operations and disposal phases of the asset management/development system: Physical geographic and geospatial referencing data, Environmental data (i.e. weather, flooding, soil types), and Susceptibility to natural hazards (fire). P4 reflected that “networks or sub-networks heavily flooded and heavily goes underwater there will be a different way of managing them. They have higher susceptibility of damage, and similar types of soil will have an impact.” Furthermore, when maintaining/modifying assets for wider roads, additional factors become significant including trees, biodiversity corridors, fish passages, etc.
The risk was another key category helping to capture priority actions, in relation to the asset type and mitigation measures available. According to participants, such data types are used mainly in the planning, construction, operation, and end-of-life planning stages. The asset management expert from Local Council 2 shared that “A potential risk might be a catastrophic failure or a failure of a component of an asset or whatever that might be. You will have various mitigation measures, one of that would be regular maintenance inspection which is a valid one. Others could be proactive maintenance, others could be a hierarchy of control” (P6). This participant also pointed out how risks are related to maintenance data and condition data, especially to programmed maintenance and cyclic maintenance and reactive maintenance.
This section considers performance, and utilisation e data, with regard to the contributions of this cluster of activity. Asset performance can be attributed to finance (the cost of asset ownership) and service (the customer service performance of the asset). Key elements under this category would be asset life, outputs, service performance, fit for purpose, community inputs, and achievement. Asset management expert from Local Council 2 (P6) expert provided a summary, reflecting that “performance is really about the asset meeting the design intent” (P6). Such data is used throughout the asset life cycle especially in planning, design, construction, acquisition, and operations. Austroads data standard was recognised as a widely used data standard for managing roads in South East Queensland. Both local council experts (P4, P6) emphasised the value of this document in having standard definitions and defined data architecture which enables consistent use of language and coding in road asset management. Moving forward, P4 proposed the use of digital communication between assets and data to be stored in clouds would facilitate the creation of a whole digital asset environment. They clearly distinguished the difference between the human’s ability to identify physical assets and the asset’s artificial intelligence could go beyond that leveraging sensor technologies, BIM, and other digital engineering methods to achieve this blue-sky thinking.
Road utilisation captures the usage of assets. Within this category traffic volumes, congestion, capacity, outputs, bicycles/pedestrians, axle load, freight use, community users, traffic growth are key subcategories predominantly used in the planning and operations phase. The asset management expert from Local Council 1 (P4) highlighted the importance of engaging community members in receiving feedback on congestions, rideability, and noise amount, condition as they are key inputs for decision making. Freight use was also re-iterated by this participant as this kind of road utilisation has a major impact on the asset. For example, “That’s one of the many challenges with regards to decision making, that network from geometry perspective or strength perspective is adequate for different kinds of loads.” Road use could be a very broad asset information requirement and having a deeper understanding of the types of uses enables more effective and efficient management of these road assets.
The authors then developed an augmented ‘Asset Interaction’ (AI) matrix to map the temporal, spatial and logical relationships between the 13 identified key asset information requirement categories. While the critical asset information requirements are identified it is also important to understand the relationships between these asset information requirements to make more prudent decision related to resilience of road infrastructure. Over the life cycle of a road, information related to the road is generated, including spatial information, such as geographical and positional attributes, and temporal information, such as the time that service opens and closes to the public [48]. At a macro-level, understanding the relationships and patterns between these pieces of information can be helpful in learning from historical data, identifying emergent trends, and predicting future scenario performance.
Table 5 synthesises the relationships derived from the interview transcripts, noting that the grey boxes represent the cross-matching of the same asset management category (i.e. not applicable). Empty cells indicate that no relationship was identified during the interviews, between the two corresponding categories. The following paragraphs discuss these logical, spatial [32] and temporal [49] relationships between various information asset requirements, and the potential for further mapping to build on this initial appreciation, where:
Spatial attributes represent three-dimensional data, taking elevation into account [50];
Temporal attributes represent data as a state in time; and
Logical attributes represent related entities and their data flow associations.
Spatial relationships discuss the data or information that identifies the geographic location of features and boundaries. Participant 1 expressed the importance of visualising spatial relationships between each category and how it will enable digital engineering to harness better outcomes in each asset information category. Key demonstrations between environment and condition data would enable decision-makers to factor in environmental changes, risk, characteristics and its impact on asset conditions. For example, “ if flooding risk were to be spatially visualised though a map and that could be a useful resource for asset managers to take necessary action to preserve the road conditions” (P1).
An asset that can be spatially visualised can then help to appreciate the road hierarchy (i.e. network critical asset) and relationships between network components. Participant 4 shared that knowing where within the tunnel they could be certain to find items (e.g. extraction fence electrical conduits), is critical to safety - having such spatial awareness is key and this could be related to the risk category as well. The categories of road use and access how clear spatial relationships. For example, “in our organisation we have interactive maps which are very similar google earth we have a number of map layers that we can turn particular things on, and off which can show us where a particular object is whichever. So, if we know what we got to do, regular maintenance needs to happen, that spatial relationship which how’s which bridge and where that show we go and inspect such and such bridge.” (P2).
There is also a spatial and logical relationship between environment and resilience categories. For example, the participant 5 shared thoughts on the recent environmental catastrophe Cyclone Debby, “First thought is the way the roads got terribly hammered after cyclone Debby and the flooding we had the last couple of years. You can put those two things down as it’s a summertime issue. And the way that flooding situation, they are one day and the next day they are gone. So, there is a timely situation there. It’s almost seasonal. How our roads respond is almost seasonal” (P5).
Preliminary Asset Interaction (AI) matrix, showing the relationships mapped during the interviews
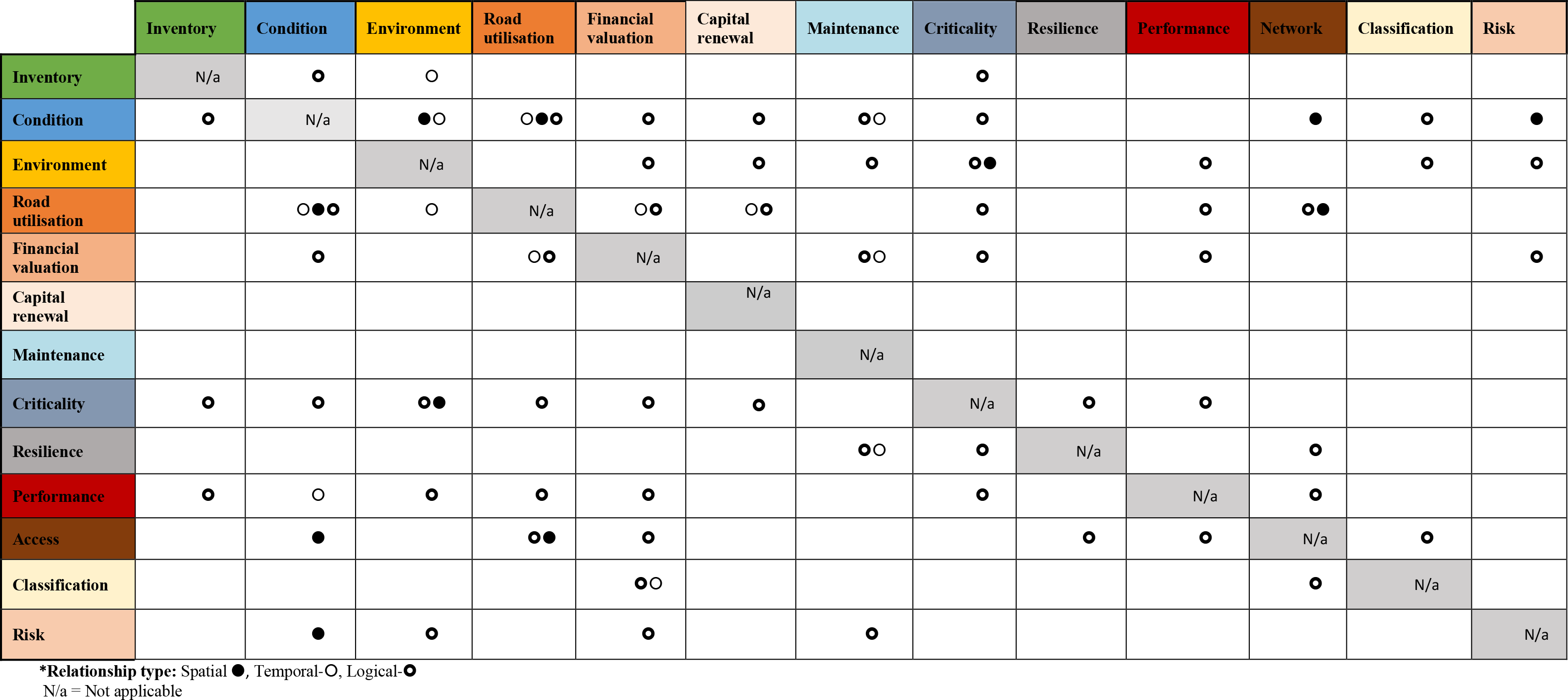
Some participants reflected that being able to visualise the network data spatially would be convenient for condition assessment. Furthermore, it was considered essential to bring in classification into every other data category, in particular connecting it to inventory data and road use types. For example, “We need to bring classification into everything, that helps us to understand what inventory data may be used. Then the condition data. For us knowing spatially where everything is key to us so then we can actually look at how do we bring into the maintenance operation of that information” (P4).
Inventory and condition, network and environment are two other key categories which would demonstrate spatial relationships. In addition, these relationships can be leveraged to manage congestion, road use, maintenance, and access. For example, “when we are designing and thinking of it, [how can] the ease of access and maintenance down the track [..] be facilitated?” (P2). Understanding these spatial relationships are therefore critical for authorities and practitioners in resilient decision making.
As discussed under spatial relationships, it is critical to identify both spatial and temporal relationships as these could have cumulative impacts on decision making. Temporal data that represents road use and condition data shows a clear temporal relationship. Participant 4 expressing their ideas on the road management, evaluation of congestion data and its impact on the road use and access, “At the point of what [asset] was down [compromised], access to certain roads might be restricted. And the road use also has a temporal relationship” (P4).
Temporal relationships are readily visible when considering environmental conditions, as they clearly vary periodically and from time to time. This has a relationship between inventory data (geographic location and environmental data). For example,” Identifying the geographic location of environmental data. That will have an impact on the way it’s been utilised … Not too long ago - 5 years ago, we looked at roads that were affected by acid sulphite (AS) soils. And AS soils budding up parts of your road network. And say you’ve stormwater network, you have created a little hotspot for potential works/ for something to happen and make sure your response is not just a band-aid response, but you would really want to take it from end to end and having a look at all your joins all your connections down the track and either side” (P5).
Network conditions, criticality and maintenance data also have clear temporal relationships. For example, “ Temporal can be in different categories, in a day congestion might be in peak time. And then a week in congestions- not that busy in the weekend. In the monthly and yearly congestion is in school holidays and non-school holidays. And season aspect and environmental aspect (P4)”
Participant 4 emphasised on the importance of defining the time domain in terms of short, medium, long terms; network, or project level. This domain brings a variety in the types of inspection and condition assessments done for infrastructure assets. For example, “Looking forward to we collect condition data every three years. Which is more network-level condition data. But our construction and maintenance branch do it every few months. They look at potholes and patches and all those operational maintenance issues. And we are looking at network improvement issues which are good enough to do every couple of years. And in a long-term indicator and not so immediate. So, this domain of short-term vs long-term, daily vs hourly, yearly vs monthly, will give you a different answer for temporal (P4)”. Above example clearly demonstrate that considering temporal relationships are essential for right time, right place use of infrastructure data for resilient outcomes. It is essential to monitor spatial and temporal relationships to comprehend the changes in assets and their impact on natural resources and environmental systems.
Logical relationships represented by standardising the people, places, entities, and the rules and relationships between them using a standard language and notation, i.e. organisational ownership and provided services are associated with operational conditions to proactively identify and respond to potential problems, relationship between assets and critical services the asset supports. In an asset management perspective, AIR demonstrates a logical relationship with each other. This consists of key examples such as condition data and inventory data. For example, Condition data and inventory data, we do try and capture the installation date and the age of the asset and some them may have that longevity, the other are starting to think again about tunnels and extraction fans the maintenance arioso there or we need to know that maintenance regime, often we need to go out maintenance. This shows a link to maintenance as well” (P6).
Condition data and financial evaluations have a unique logical relationship. For example, “ If we think about how well each different pavement types perform- expectation about an optimal lifetime- truck may come along as an increase over the lifetime a great increase in heavy vehicles starts to erode its performance. Then the surface needs to be maintained than earlier would have when planned at the installation. Traffic volumes and other outside factors will affect the resilience” (P1)
The road use and the environment/ network have a close spatial and logical relationship. For example, “We looked at roads that were running through Koala corridors. We were looking at roads that went close to hospitals, close to shopping centres, schools. Close to school is interesting because you got heavy busloads (P5).”
Resilience and condition data, maintenance data also demonstrate a logical relationship. For example, “Inspection of a 6 monthly regular maintenance needs to occur; you do get the resilience piece out as well. That you install. To maintain the longevity of life. Resilience comes back to the fact that dollars spent as well. Let’s get longevity per dollar, high price and if something fails that’s a problem” (P4).
Performance can be taken as an example of a different level of road use and works and costs. For example, “Performance connected to works and costs. Road use – changes of volume- temporal relationship” P2). The costs, network, and classification also show a logical relationship. For example, the type of the network, it sits in the hierarchy of our planning process that we will look at that network need may be, need to connect to finances.
There was a clear logical relationship shared among network and environment. For example, “Capacity on the network and the environment – carbon- through a failure of the network that leads to congestion actually has an impact in the environment- greater carbon exposure” (P1). In addition, another participant added, “ construction of the network its impact on the environment and the material we use – build particular assets -highlighted for us a concrete- connection- embodied energy” (P2). A deeper understanding of logical relationships is therefore vital for decision making related to complex data associations.
With the abovementioned logical, spatial, and temporal relationships among AIRs authorities and decision makers can now make more informed decision to manage resilience in infrastructure. Figure 5 presents the identified links and relationships between asset information requirements and decision making.
According to the interviewees the decision-making process consists of key steps including review context, define objectives, evaluate influencing factors, investigate causes of action, engage stakeholders, and plan. With these clearly defined steps decision makers can now adopt an evidence-based approach to better manage resilient road infrastructure.
This study contributes to the infrastructure asset management field by providing insights into the experiences and perspectives of senior decision-makers from the asset management sector (industry and government) regarding critical asset information requirements. It is clear from analysing the interviews data that digital engineering creates a myriad of opportunities for infrastructure management to streamline operations and improve overall asset performance. The authors conclude the importance of a common understanding of asset information requirements for authorities and practitioners to evaluate right time, right place capture of infrastructure data for resilient outcomes.
Asset information and decision-making process
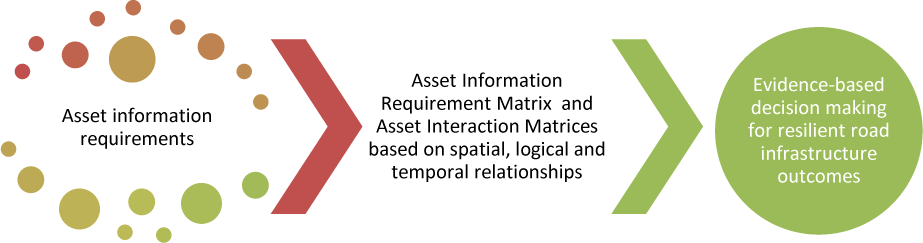
The presented ‘Asset Information Requirement Matrix’ categorises 66 data requirements across four key infrastructure data types (including 13 information categories), summarising the relevance of these data requirements for the six key phases of planning, design, construction, acquisition, operations and end-of-life treatment. The presented ‘Asset Interaction Matrix’ depicts the temporal, spatial and logical relationships between the 13 information categories. This level of granularity of data type, categorised by function, can assist decision-makers in understanding the potential for further enquiry into the existing available data, and potential opportunities for gathering other meaningful data to support their asset management decisions going forward. Furthermore, DE technologies such as BIM, GIS, 2D, 3D and multidimensional models then enable better recording, visualisation, and communication of asset information requirements using collaborative and productive methods through the asset lifecycle.
This study has immediate practical implications for asset managers/ owners to improve their performance by considering the data types outlined. Considering the potential for DE in enabling resilient road infrastructure, through digitalized data management and streamlined processes authorities and practitioners can improve road resilience in the face of extreme weather events. Early planning for resilience will improve the lifespan of infrastructure reducing the risks of failure. Such planning can also achieve cost savings in terms of energy and resources used of maintenance, re-work and rebuilding of road infrastructure and manage it in a sustainable manner.
Within the construction sector, immediate opportunities exist for mainstreaming the use of DE in enabling better infrastructure outcomes. This includes replicating successful digital strategies and tools for managing complex data related to asset management and interoperable systems, beyond champion-based, ad hoc examples, and case studies. It also includes raising awareness between key stakeholders including councils, contractors, and government, regarding the potential for DE to support various road management responsibilities and programs of works.
Looking beyond the construction sector, there are wider applications in having a common language to discuss the spectrum of data types, including for example the machine and process industry. There is also the potential for artificial intelligence to use existing data sets to ‘learn’ how to optimise road infrastructure management, enabling direct communication among infrastructure assets, and interact with transport technology such as autonomous vehicles. With this future in mind, herein the authors intend to refine the matrices, enabling further dialogue between local council, industry and government towards digital engineering practices that can benefit resilient infrastructure, streamlined data systems and interoperability.
AIR |
Asset Information Requirement |
AIM |
Asset Integrity Management |
BIM |
Building Information Modeling |
BMS |
Bridge Management System |
BrIM |
Bridge Information Modeling |
CAD |
Computer-aided design |
DE |
Digital engineering |
GIS |
Geographic Information Systems |
GPS |
Global Positioning System |
UNSDG |
United Nations Sustainable Development Goal |
- Department of Infrastructure, Transport, Cities and Regional Development Australian Government, Perspectives on Australian transport infrastructure 2019, https://www.infrastructure.gov.au/department/media/mr_201906-perspectives-on-australian-transport-infrastructure.aspx
- What is digital engineering 2018, https://www.aurecongroup.com/expertise/digital-engineering-and-advisory/defining-digital-engineering
- Digital Engineering 2020, http://www.laingorourke.com/engineering-the-future/digital-engineering-2.aspx
- The Department of Infrastructure, Transport, Cities and Regional Development Australian Government, National Digital Engineering Policy Principles 2016, https://www.infrastructure.gov.au/infrastructure/ngpd/files/Principles-for-DE_Template_2.pdf
- Digital Engineering and the Future of Operations 2020, https://www.idc.com/getdoc.jsp?containerId=US46217020
- Department of Infrastructure, Transport, Cities and Regional Development Australian Government, National Digital Engineering Policy Principles 2018, https://www.infrastructure.gov.au/infrastructure/ngpd/files/Principles-for-DE_Template_2.pdf
Exploring the role of digital twin for asset lifecycle management. ,IFAC-PapersOnLine. , Vol. 51 ,pp 790-795 , 2018, https://doi.org/https://doi.org/10.1016/j.ifacol.2018.08.415
, Systems information modelling: Enabling digital asset management. ,Advances in Engineering Software. , Vol. 102 (1),pp 155-165 , 2016, https://doi.org/http://doi.org/10.1016/j.advengsoft.2016.10.007
, - Digital Asset Information Management (DAIM): Case Studies, 2018, https://sbenrc.com.au/app/uploads/2019/05/SBE0281.pdf
Systems information modelling: Enabling digital asset management. ,Advances in Engineering Software. , Vol. 102 (1),pp 155-165 , 2016, https://doi.org/http://doi.org/10.1016/j.advengsoft.2016.10.007
, Leveraging socio-ecological resilience theory to build climate resilience in transport infrastructure. ,Transport Reviews. , Vol. 39 (5),pp 677-699 , 2019, https://doi.org/https://doi.org/10.1080/01441647.2019.1612480
, Escalating impacts of climate extremes on critical infrastructures in Europe. ,Global Environmental Change. , Vol. 48 ,pp 97-107 , 2018, https://doi.org/https://doi.org/10.1016/j.gloenvcha.2017.11.007
, Operational models of infrastructure resilience. ,Risk Analysis. , Vol. 35 (4),pp 562-586 , 2015, https://doi.org/https://doi.org/10.1111/risa.12333
, A resilience engineering approach for sustainable safety in green construction. ,Journal of Sustainable Development of Energy, Water and Environment Systems. , Vol. 5 (4),pp 480-495 , , https://doi.org/https://doi.org/2017, 10.13044/j.sdewes.d5.0174
, Do you have a Plan B? Integrating adaptive capacities into infrastructure preparedness planning. ,Journal of Contingencies and Crisis Management. , Vol. 26 (3),pp 368-376 , 2018, https://doi.org/https://doi.org/10.1111/1468-5973.12203
, BIM for infrastructure: An overall review and constructor perspective. ,Automation in Construction , Vol. 71 ,pp 139-152 , 2016, https://doi.org/https://doi.org/10.1016/j.autcon.2016.08.019
, - New South Wales Government, T.f.W.S.W. Digital engineering Framework 2020, https://www.transport.nsw.gov.au/digital-engineering/digital-engineering-framework
Historic Building Information Modelling: performance assessment for diagnosis-aided information modelling and management. ,Automation in Construction. , Vol. 86 ,pp 256-276 , 2018, https://doi.org/https://doi.org/10.1016/j.autcon.2017.11.009
, Developing owner information requirements for BIM-enabled project delivery and asset management. ,Automation in Construction. , Vol. 83 ,pp 169-183 , 2017, https://doi.org/https://doi.org/10.1016/j.autcon.2017.08.006
, Implementing BIM on infrastructure: Comparison of two bridge construction projects. ,Practice Periodical on Structural Design and Construction. , Vol. 20 (4),pp 04014044 1-8 , 2014, https://doi.org/https://doi.org/10.1061/(ASCE)SC.1943-5576.0000239
, From justification to evaluation: Building information modeling for asset owners. ,Automation in construction. , Vol. 35 ,pp 208-216 , 2013, https://doi.org/https://doi.org/10.1016/j.autcon.2013.05.008
, Bridge Information Modeling for the Life Cycle. ,Proceedings of Tenth International Conference on Bridge and Structure Management ,pp 313-323 ,
, Application of 3D bridge information modeling to design and construction of bridges. ,Procedia Engineering. , Vol. 14 ,pp 95-99 , 2011, https://doi.org/https://doi.org/doi:10.1016/j.proeng.2011.07.010
, Bridge information modeling in sustainable bridge management, Proceedings of International Conference on Sustainable Design and Construction (ICSDC) ,pp 457-466 , 2011
, SeeBridge as next generation bridge inspection: overview, information delivery manual and model view definition. ,Automation in Construction. , Vol. 90 ,pp 134-145 , 2018, https://doi.org/https://doi.org/10.1016/j.autcon.2018.02.033
, Asset management as integral part of road economy. ,Procedia Engineering. , Vol. 91 ,pp 481-486 , 2014, https://doi.org/https://doi.org/10.1016/j.proeng.2014.12.030
, Documentation errors in instrumentation and electrical systems: Toward productivity improvement using System Information Modeling. ,Automation in Construction. , Vol. 35 ,pp 448-459 , 2013, https://doi.org/https://doi.org/10.1016/j.autcon.2013.05.028
, - , 4D visualization of highway construction projects. Proceedings of Seventh International Conference on Information Visualization
Using nD technology to develop an integrated construction management system for city rail transit construction. ,Automation in Construction. , Vol. 21 ,pp 64-73 , 2012, https://doi.org/https://doi.org/10.1016/j.autcon.2011.05.013
, Towards a comprehensive asset integrity management (AIM) approach for European infrastructures. ,Transportation Research Procedia. , Vol. 14 ,pp 4060-4069 , 2016, https://doi.org/https://doi.org/10.1016/j.trpro.2016.05.50
, Bringing infrastructure into pricing in road freight transportation–A measuring concept based on navigation service data. ,Transportation research procedia. , Vol. 25 ,pp 794-805 , 2017, https://doi.org/https://doi.org/10.1016/j.trpro.2017.05.458
, The role of GIS in asset management: Integration at the Otay Water Distict, M.Sc. Thesis, Faculty of the USC Graduate School , 2012
, An integrated system framework of building information modelling and geographical information system for utility tunnel maintenance management. ,Tunnelling and Underground Space Technology. , Vol. 79 ,pp 263-273 , 2018, https://doi.org/https://doi.org/10.1016/j.tust.2018.05.010
, WebGIS for Asset Management of Land and Building of Madiun City Government. ,Procedia Computer Science. , Vol. 124 ,pp 437-443 , 2017, https://doi.org/https://doi.org/10.1016/j.procs.2017.12.175
, Application areas and data requirements for BIM-enabled facilities management. ,Journal of construction engineering and management. , Vol. 138 (3),pp 431-442 , 2011, https://doi.org/https://doi.org/10.1061/(ASCE)CO.1943-7862.0000433
, - Data Standard for Road Management and Investment in Australia and New Zealand: Version 2 2018, https://austroads.com.au/publications/asset-management/ap-t334-18
- Data standard for road management and investment in australia and new zealand., 2019, https://austroads.com.au/publications/asset-management/ap-t334-18/media/AP-T334-18_Austroads_Data_Standard_V2.pdf
Transforming manufacturing to be ‘good for planet and people’, through enabling lean and green thinking in small and medium-sized enterprises. ,Sustainable Earth , Vol. 2 (1),pp 1-4 , 2019, https://doi.org/https://doi.org/10.1186/s42055-019-0011-z
, Taking qualitative methods in organization and management research seriously. ,Qualitative Research in Organizations and Management: An International Journal , Vol. 1 (1),pp 4-12 , 2006, https://doi.org/https://doi.org/10.1108/17465640610666606
, - , Discourse and social psychology: Beyond attitudes and behaviour, 1987
- , Qualitative data analysis with NVivo, 2013
- , Using Nvivo as a research management tool: A case narrative. in Quality and Impact of Qualitative Research, Proceedings of the 3rd International Conference on Qualitative Research in IT & IT in Qualitative Research., 2006
- , The discovery of grounded theory: Strategies for qualitative research, 2009
- , Qualitative research: the essential guide to theory and practice, 2013
Using thematic analysis in psychology. ,Qualitative research in psychology , Vol. 3 (2),pp 77-101 , 2006, https://doi.org/https://doi.org/10.1191/1478088706qp063oa
, Longitudinal field research on change: Theory and practice. ,Organization science , Vol. 1 (3),pp 267-292 , 1990, https://doi.org/http://dx.doi.org/10.1287/orsc.1.3.267
, Building information modelling–Experts’ views on standardisation and industry deployment. ,Advanced engineering informatics , Vol. 22 (2),pp 271-280 , 2008, https://doi.org/https://doi.org/10.1016/j.aei.2007.03.001
, The circle: architecture for integrating software. ,Journal of computing in civil engineering , Vol. 9 (2),pp 122-133 , 1995, https://doi.org/https://doi.org/10.1061/(ASCE)0887-3801(1995)9:2(122)
, A framework for road change detection and map updating. International archives of the PhotogrammetryRemote sensing and Spatial information sciences , Vol. 35 (2B),pp 720-734 , 2004
, Data model–centered four-dimensional information management system for road maintenance. ,Journal of computing in civil engineering , Vol. 27 (5),pp 497-510 , 2013, https://doi.org/https://doi.org/10.1061/(ASCE)CP.1943-5487.0000176
,